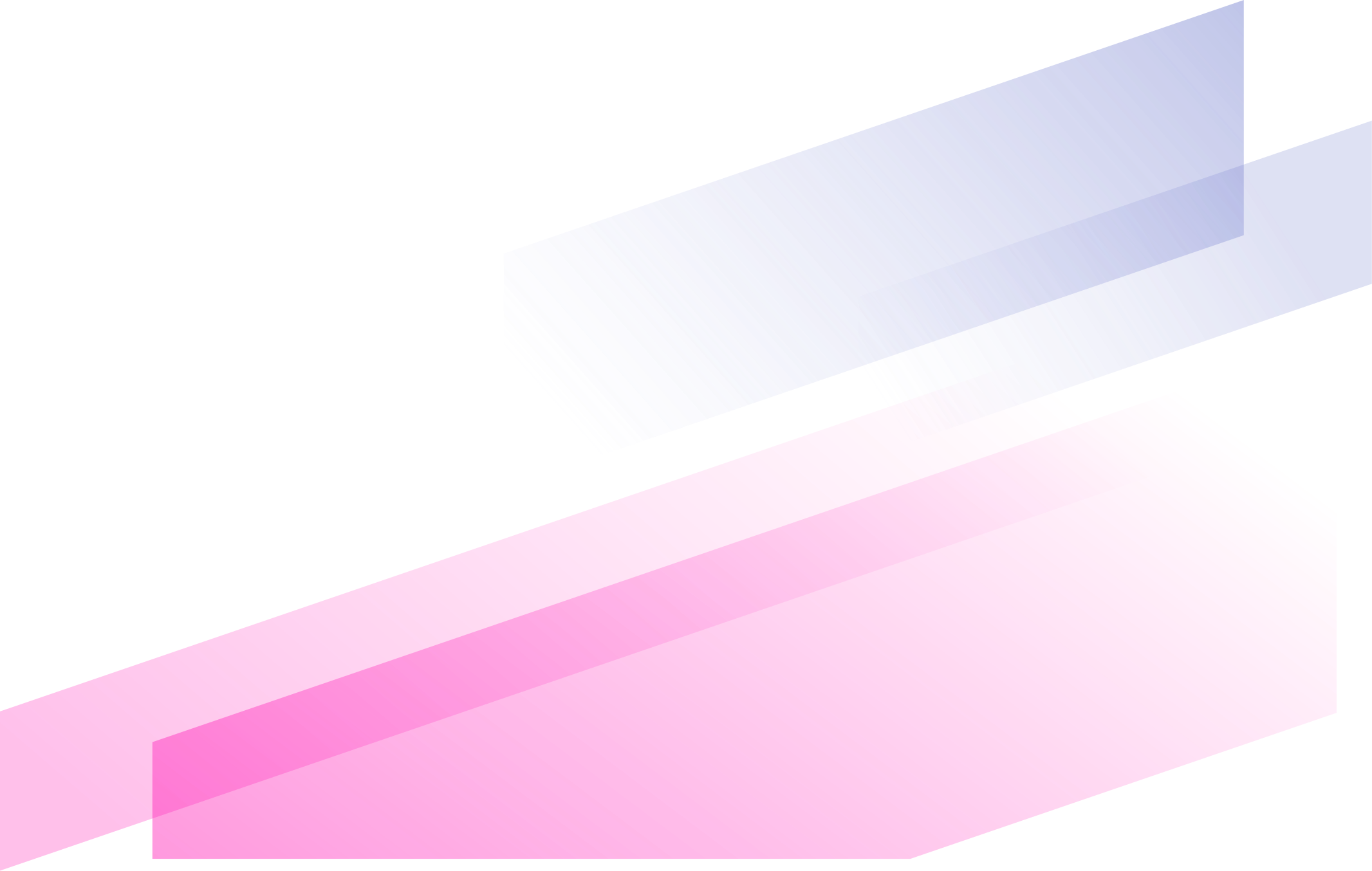
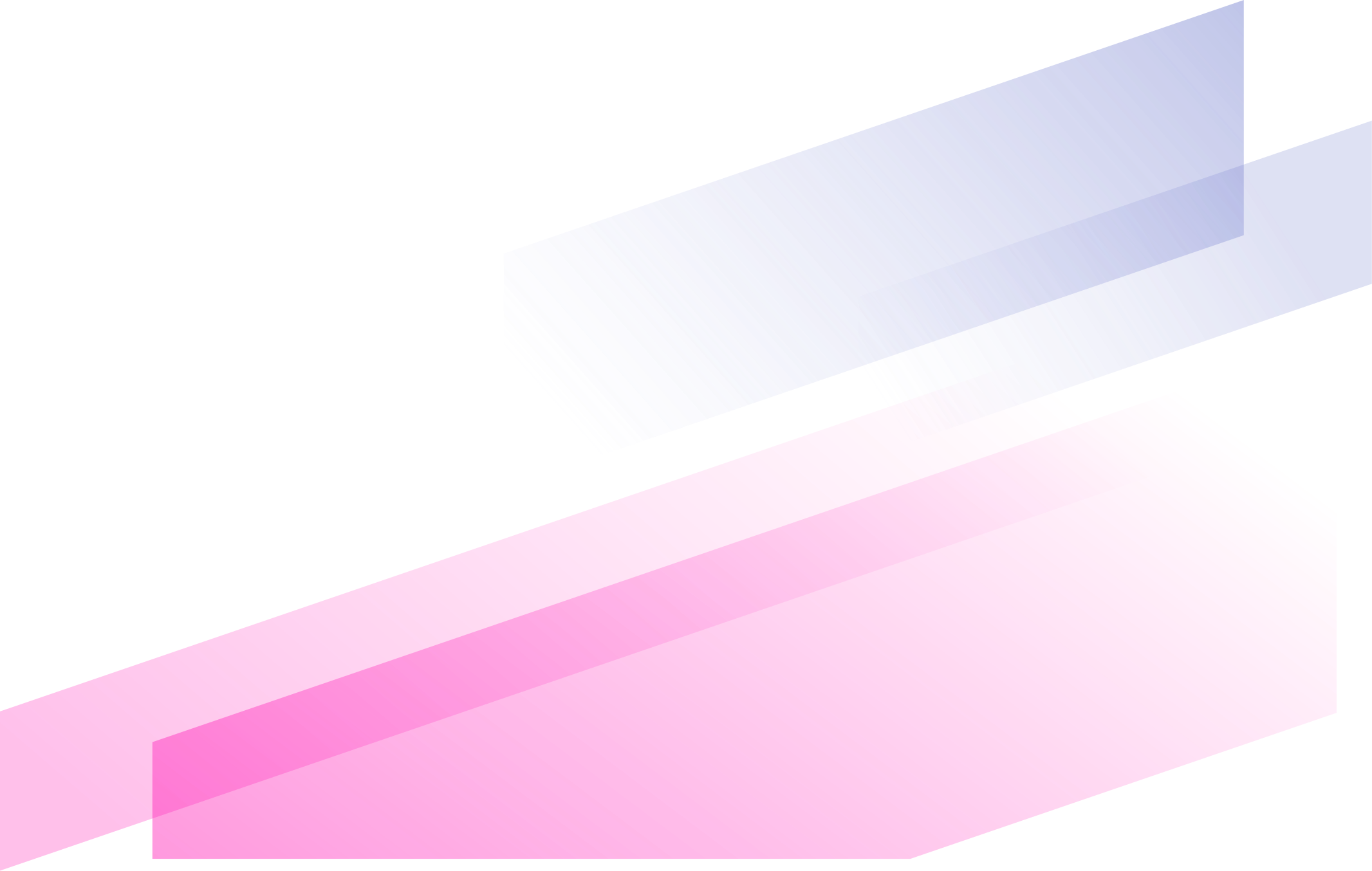
Study on the Antibreast Cancer Mechanism and Bioactive Components of Si-Wu-Tang by Cell Type-Specific Molecular Network.
Pharmacodynamics of Ceftiofur Selected by Genomic and Proteomic Approaches of Streptococcus parauberis Isolated from the Flounder, Paralichthys olivaceus.
TCMIO: A Comprehensive Database of Traditional Chinese Medicine on Immuno-Oncology.
Integration of transcriptomic profile of SARS-CoV-2 infected normal human bronchial epi-thelial cells with metabolic and protein-protein interaction networks.
A systematic strategy for the investigation of vaccines and drugs targeting bacteria.
Constructing knowledge graphs and their biomedical applications.
A Novel Triple Matrix Factorization Method for Detecting Drug-Side Effect Association Based on Kernel Target Alignment.
Elucidation of the Mechanism of Action of Ginseng Against Acute Lung Injury/Acute Respiratory Distress Syndrome by a Network Pharmacology-Based Strategy.
DaiCee: A database for anti-cancer compounds with targets and side effect profiles.
Identifying Small Molecule-miRNA Associations Based on Credible Negative Sample Selection and Random Walk.