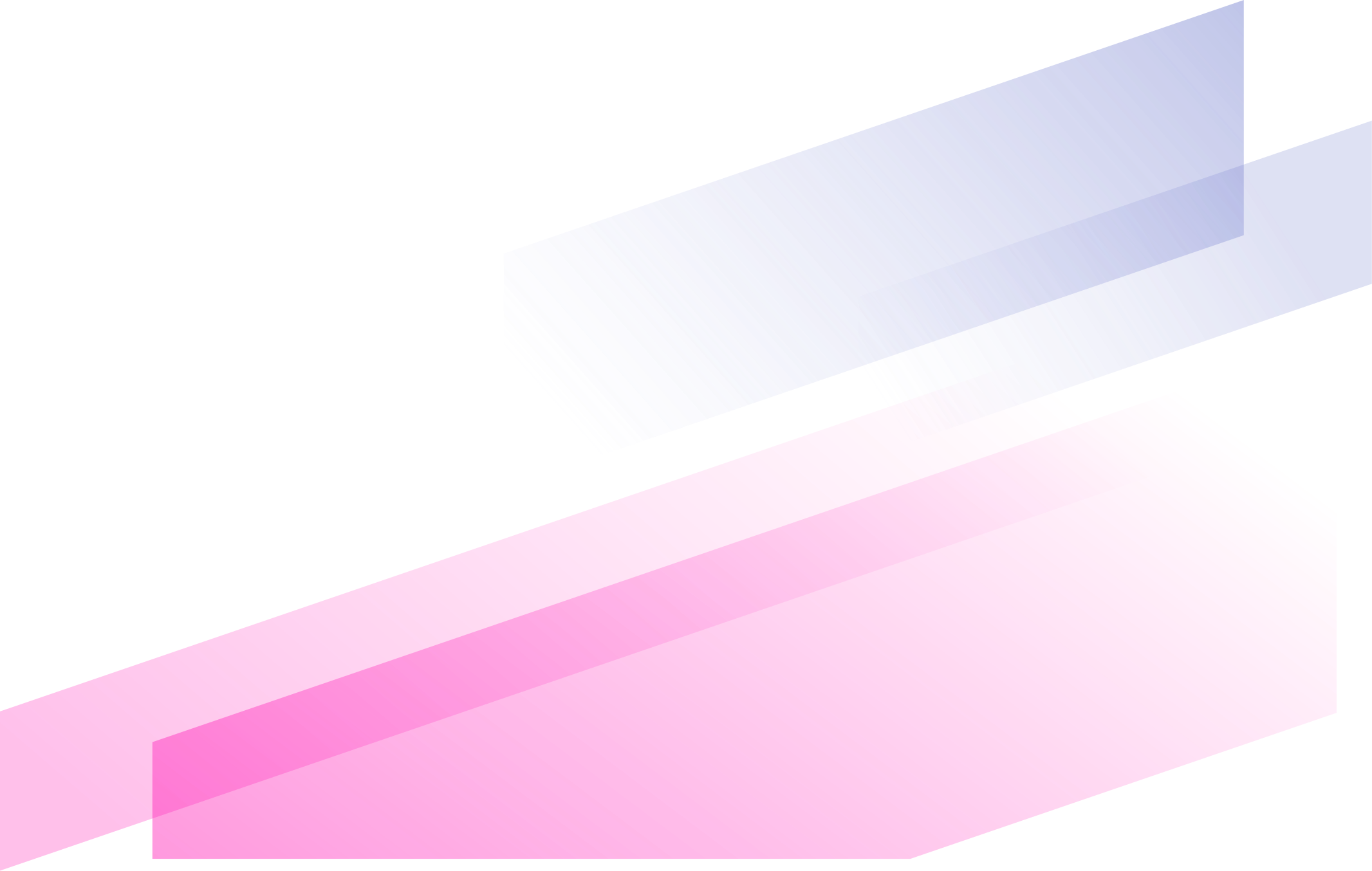
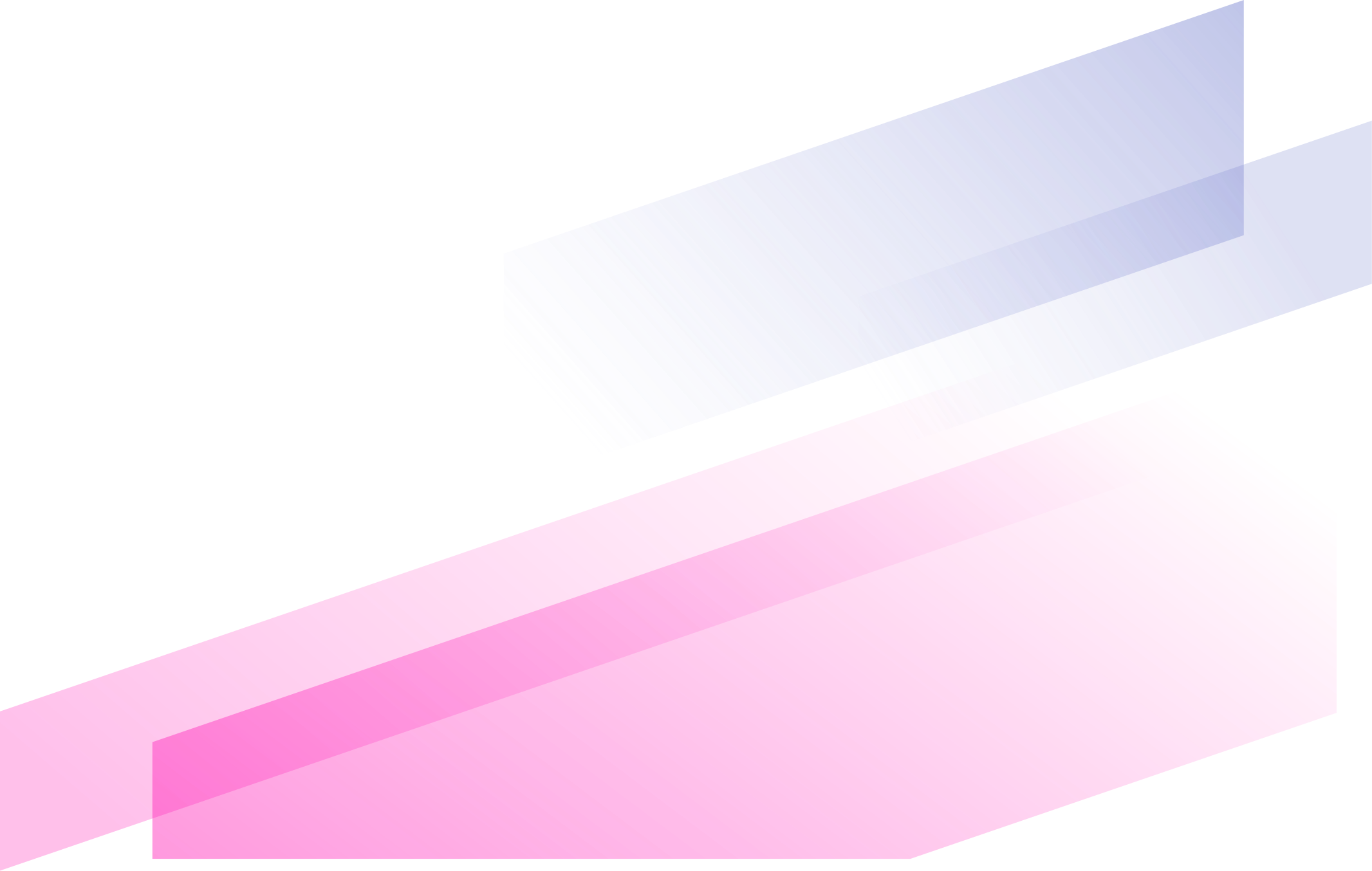
ASD v2.0: updated content and novel features focusing on allosteric regulation.
Gene3D: Multi-domain annotations for protein sequence and comparative genome analysis.
UniHI 7: an enhanced database for retrieval and interactive analysis of human molecular interaction networks.
Toward creation of a cancer drug toxicity knowledge base: automatically extracting cancer drug-side effect relationships from the literature.
ArchDB 2014: structural classification of loops in proteins.
Therapeutic target database update 2014: a resource for targeted therapeutics.
PDBsum additions.
The Reactome pathway knowledgebase.
FireDB: a compendium of biological and pharmacologically relevant ligands.
Engineered reversal of drug resistance in cancer cells--metastases suppressor factors as change agents.