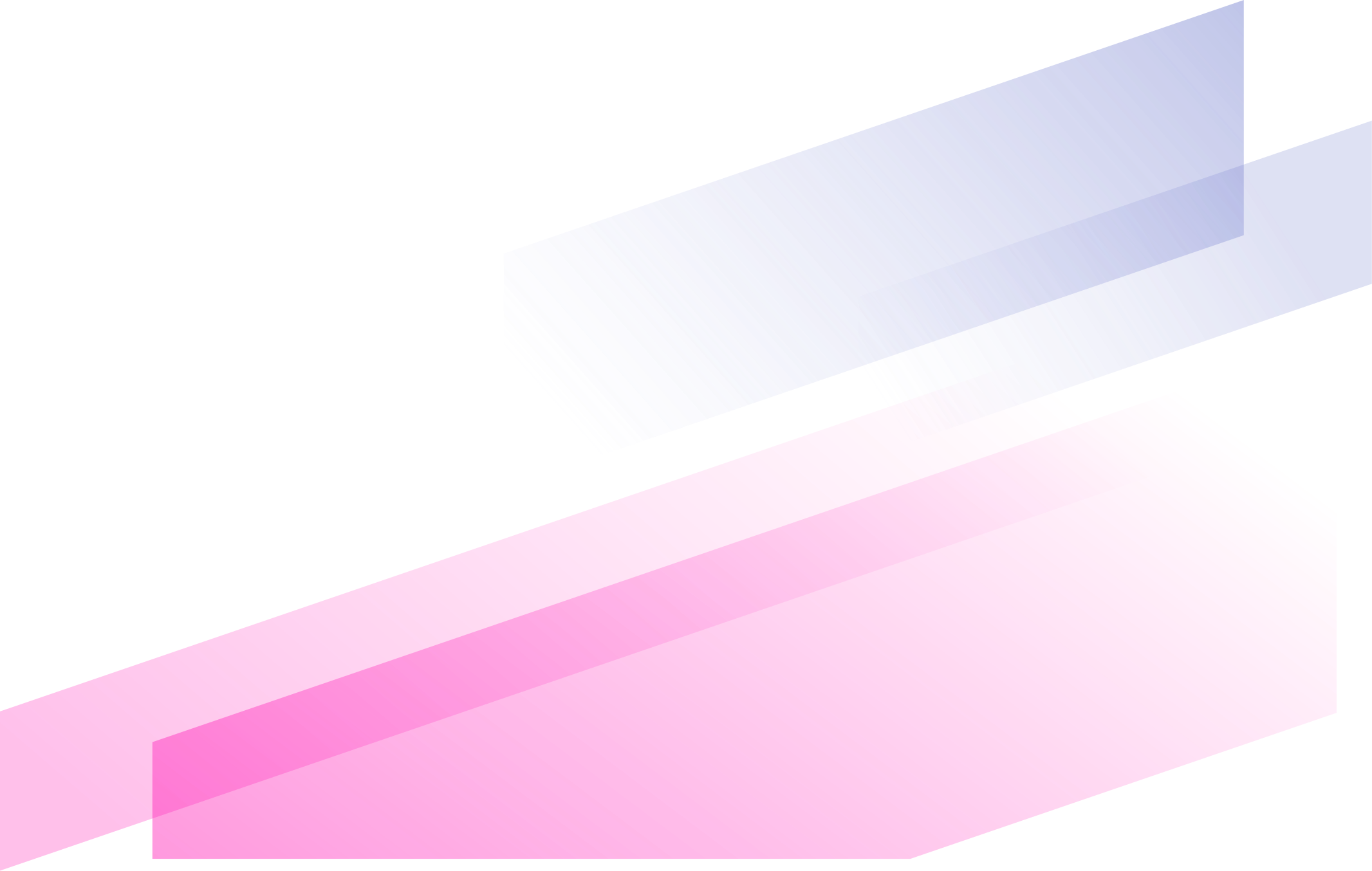
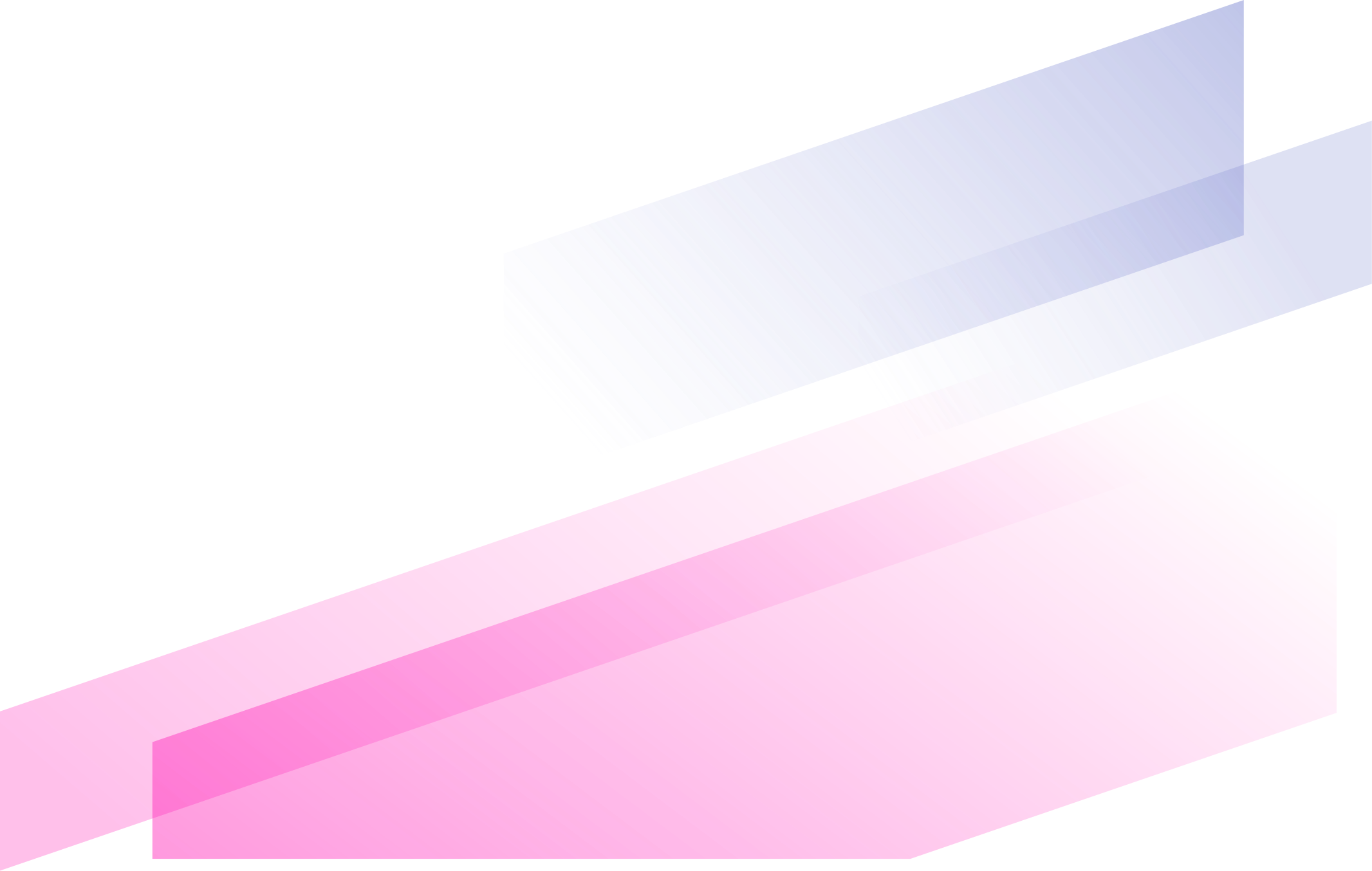
Effects of Sulfamethoxazole on Fertilization and Embryo Development in the Arbacia lixula Sea Urchin.
Signatures of Co-Deregulated Genes and Their Transcriptional Regulators in Lung Cancer.
Prediction of drug-drug interaction events using graph neural networks based feature extraction.
Generation of dual-gRNA library for combinatorial CRISPR screening of synthetic lethal gene pairs.
Computational prediction and interpretation of druggable proteins using a stacked ensemble-learning framework.
DrDimont: explainable drug response prediction from differential analysis of multi-omics networks.
Implications of the Essential Role of Small Molecule Ligand Binding Pockets in Protein-Protein Interactions.
An unexpected single crystal structure of nickel(II) complex: Spectral, DFT, NLO, magnetic and molecular docking studies.
Therapeutic Targeting the Allosteric Cysteinome of RAS and Kinase Families.
Fragment-Based Drug Discovery against Mycobacteria: The Success and Challenges.