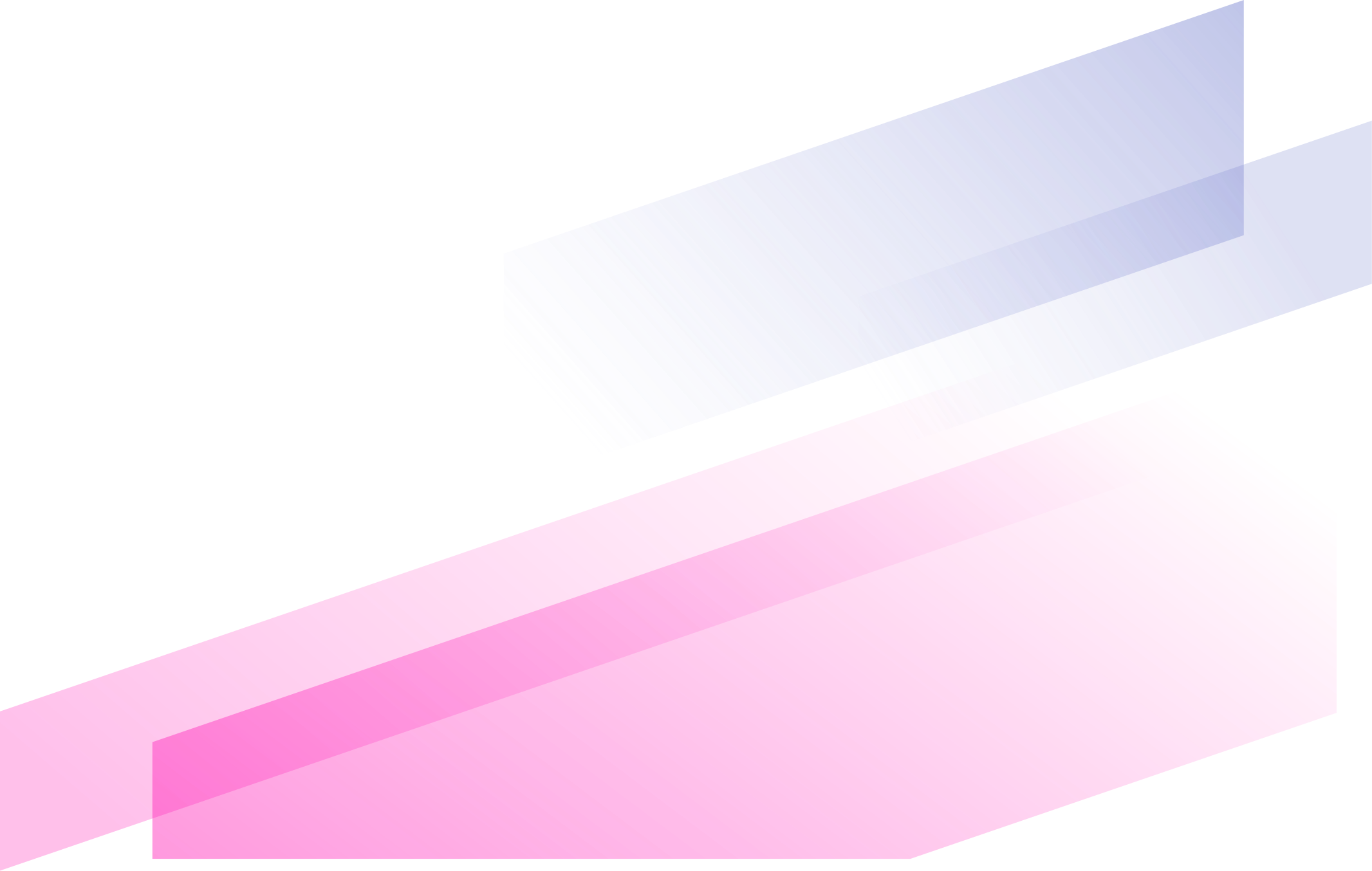
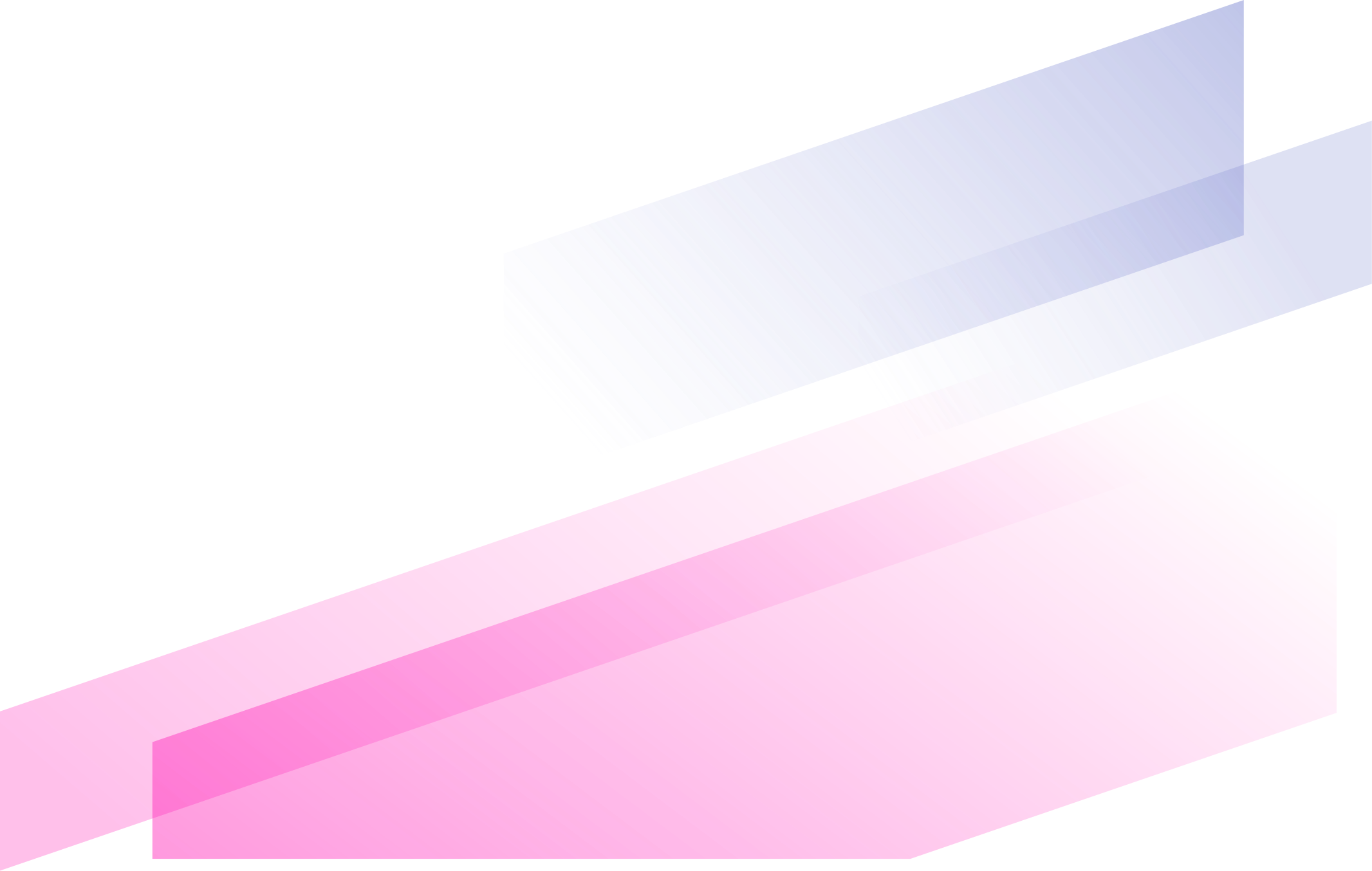
In silico identification of the rare-coding pathogenic mutations and structural modeling of human NNAT gene associated with anorexia nervosa.
Identification of hub pathways and drug candidates in gastric cancer through systems biology.
High-Throughput Measurement and Machine Learning-Based Prediction of Collision Cross Sections for Drugs and Drug Metabolites.
Advancing drug safety science by integrating molecular knowledge with post-marketing adverse event reports.
Further delineation of familial polycystic ovary syndrome (PCOS) via whole-exome sequencing: PCOS-related rare FBN3 and FN1 gene variants are identified.
Controlling astrocyte-mediated synaptic pruning signals for schizophrenia drug repurposing with deep graph networks.
A Network Pharmacology Analysis of Cytotoxic Triterpenes Isolated from Euphorbia abyssinica Latex Supported by Drug-likeness and ADMET Studies.
Machine Learning in Causal Inference: Application in Pharmacovigilance.
Immune-Related Biomarkers Associated with Lung Metastasis from the Colorectal Cancer Microenvironment.
Targeted Therapy for Adrenocortical Carcinoma: A Genomic-Based Search for Available and Emerging Options.