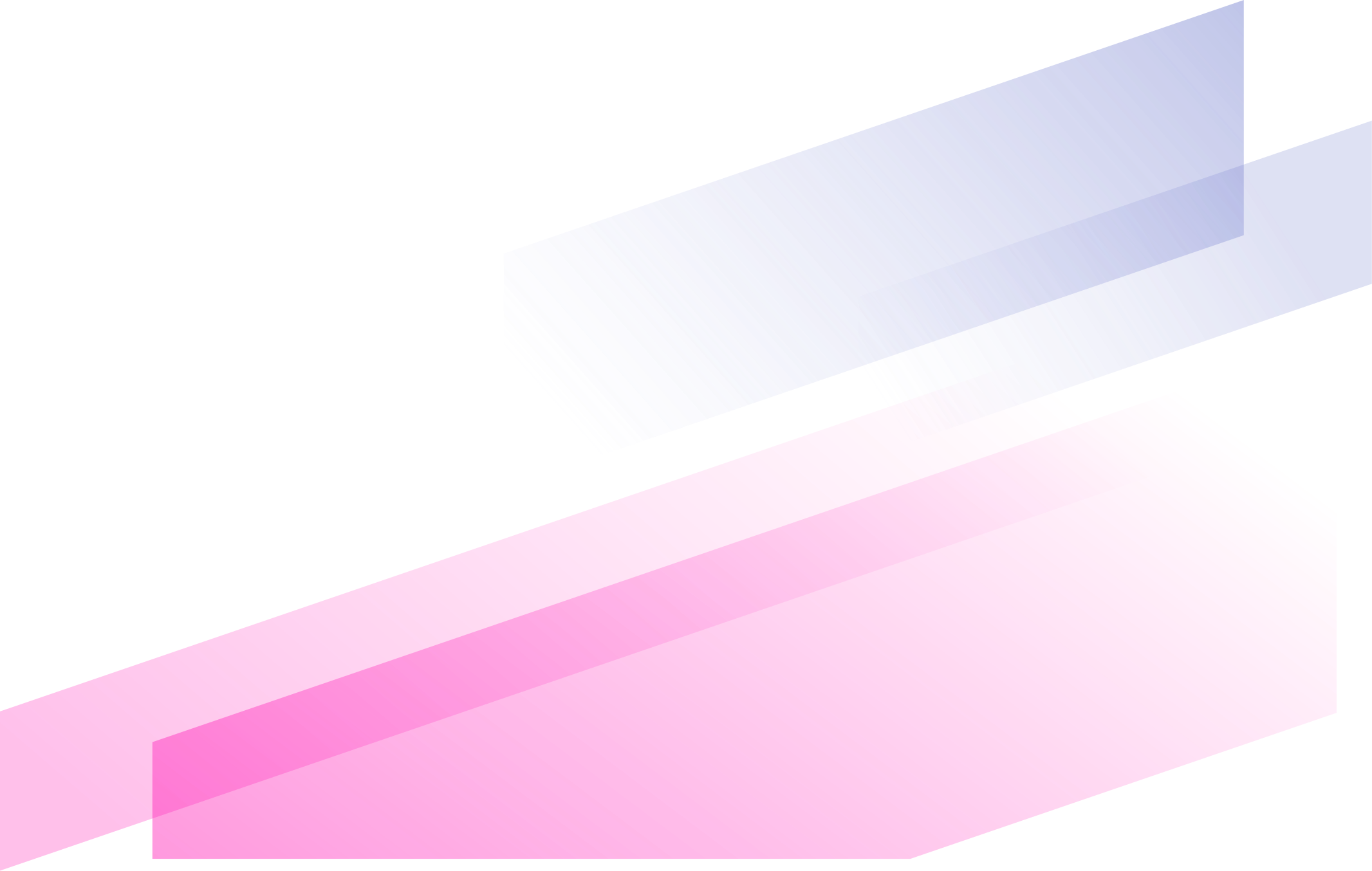
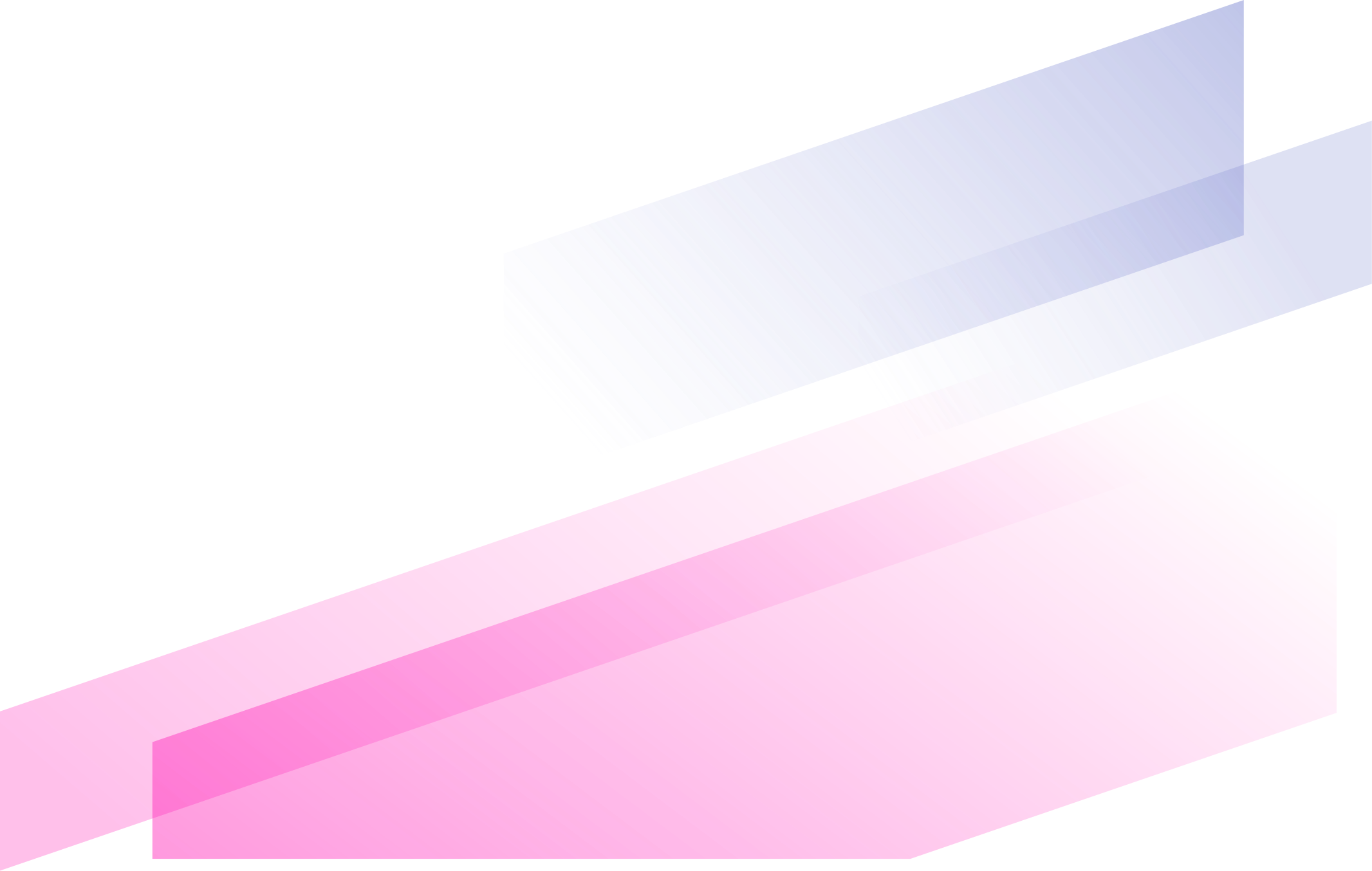
Mono- and combinational drug therapies for global viral pandemic preparedness.
Machine learning to predict metabolic drug interactions related to cytochrome P450 isozymes.
Omics-based ecosurveillance for the assessment of ecosystem function, health, and resilience.
From pharmacogenetics to pharmaco-omics: Milestones and future directions.
A Consensus Compound/Bioactivity Dataset for Data-Driven Drug Design and Chemogenomics.
HGDTI: predicting drug-target interaction by using information aggregation based on heterogeneous graph neural network.
A conditional gene-based association framework integrating isoform-level eQTL data reveals new susceptibility genes for schizophrenia.
Deep Neural Network-Assisted Drug Recommendation Systems for Identifying Potential Drug-Target Interactions.
Implications of Bariatric Surgery on the Pharmacokinetics of Antiretrovirals in People Living with HIV.
HINT: Hierarchical interaction network for clinical-trial-outcome predictions.