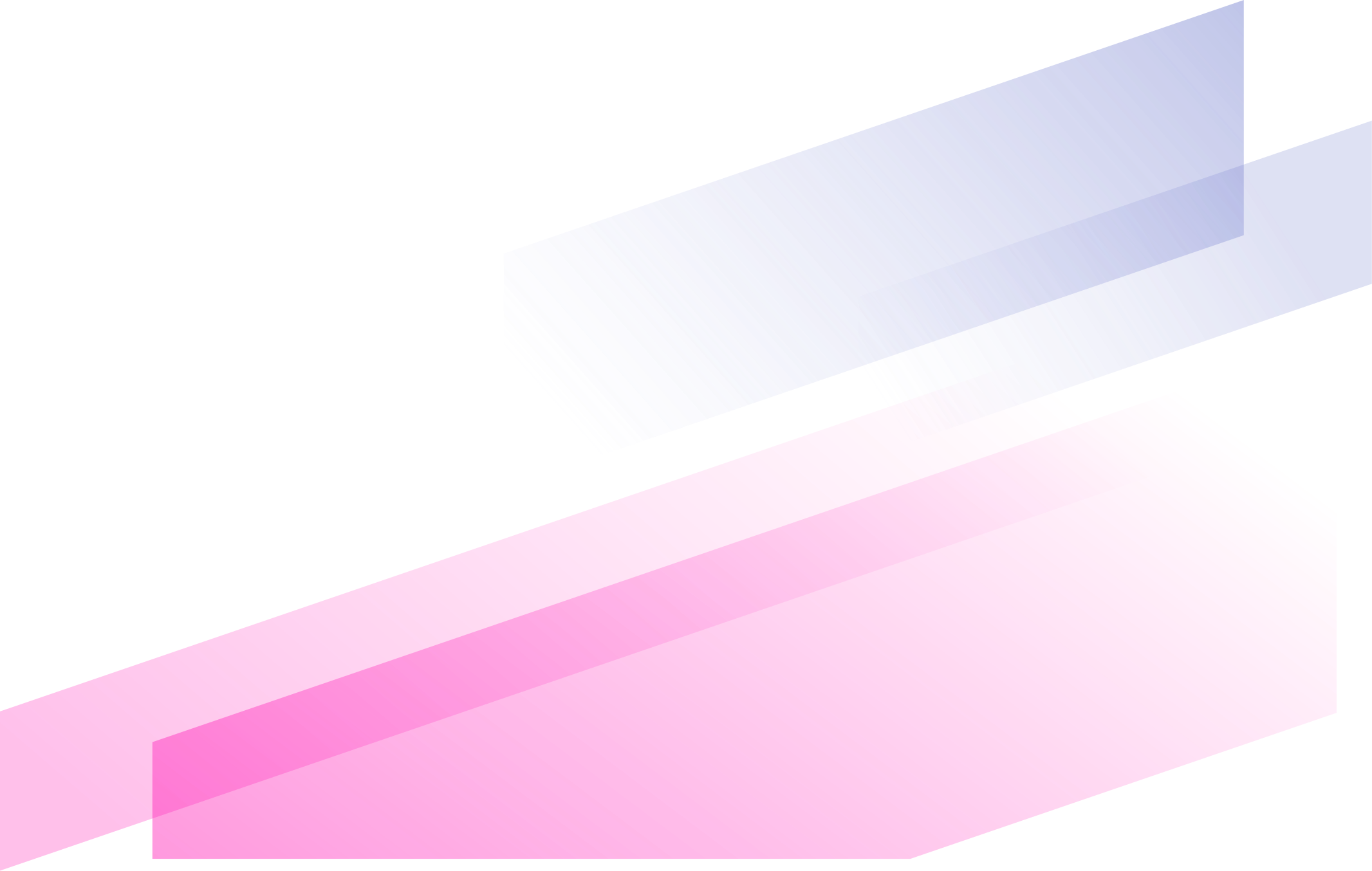
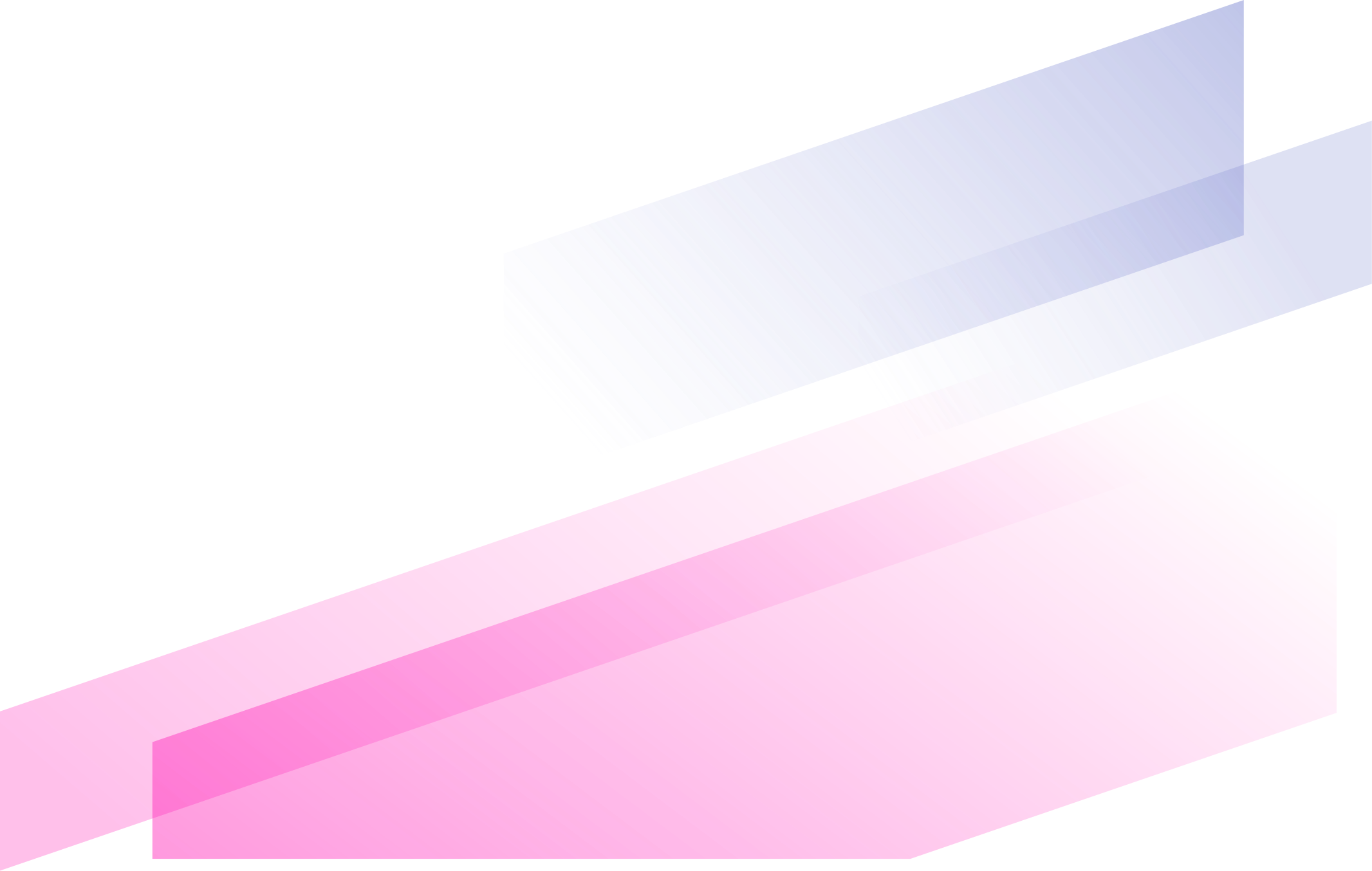
Non-interacting, Non-opioid, and Non-barbiturate Containing Acute Medication Combinations in Headache: A Pilot Combinatorics Approach Based on DrugBank Database.
An Open Access Database of Licensed Cancer Drugs.
The Relevance of Bioinformatics Applications in the Discovery of Vaccine Candidates and Potential Drugs for COVID-19 Treatment.
Computational screening of FDA approved drugs of fungal origin that may interfere with SARS-CoV-2 spike protein activation, viral RNA replication, and post-translational modification: a multiple target approach.
Drug-Target Interaction Prediction via Dual Laplacian Graph Regularized Logistic Matrix Factorization.
Chemoinformatic Analysis of Psychotropic and Antihistaminic Drugs in the Light of Experimental Anti-SARS-CoV-2 Activities.
SeBioGraph: Semi-supervised Deep Learning for the Graph via Sustainable Knowledge Transfer.
Ligand Docking Methods to Recognize Allosteric Inhibitors for G-Protein-Coupled Receptors.
Functional drug-target-disease network analysis of gene-phenotype connectivity for curcumin in hepatocellular carcinoma.
Pharmacological Mechanism of Danggui-Sini Formula for Intervertebral Disc Degeneration: A Network Pharmacology Study.