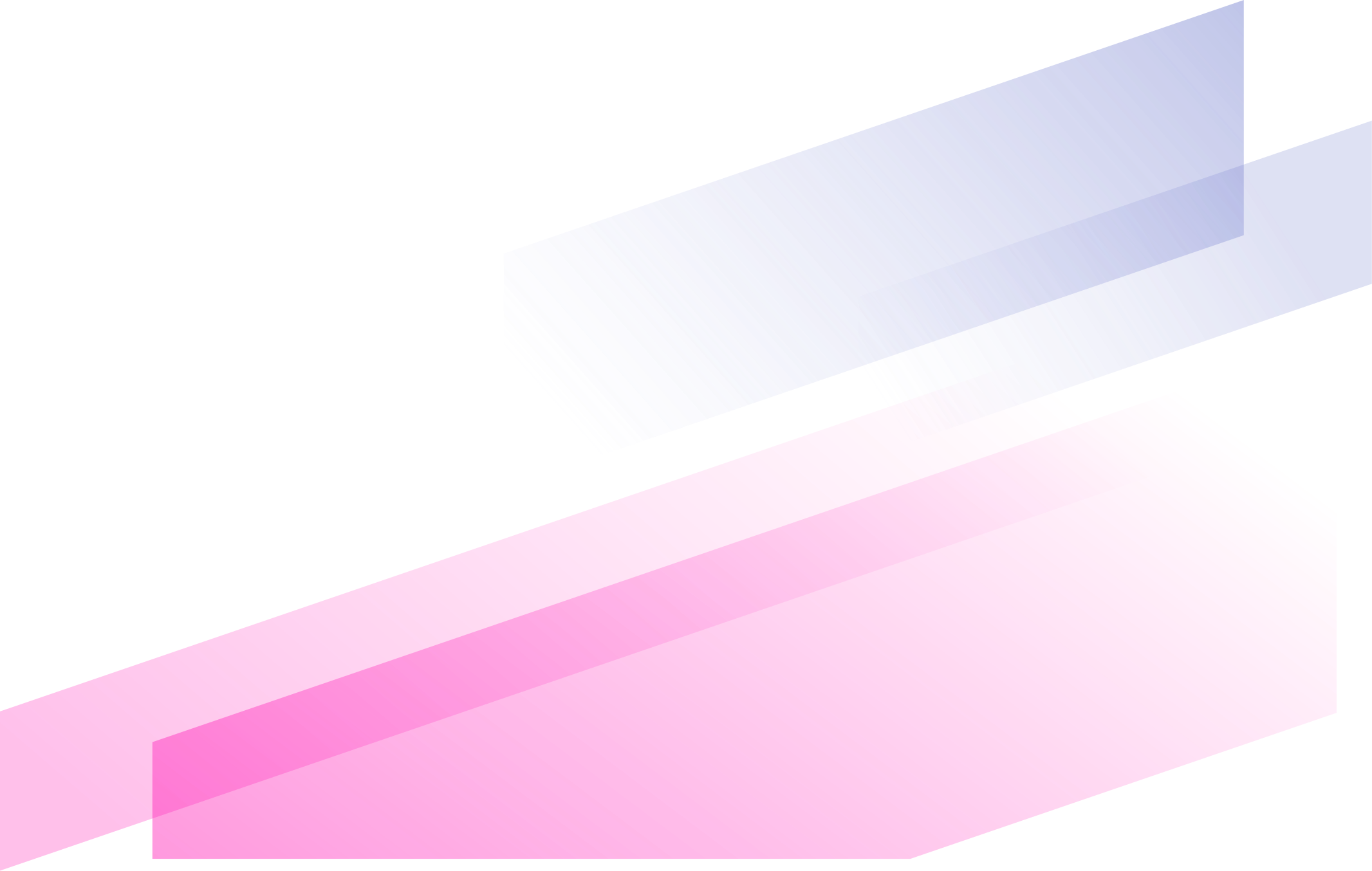
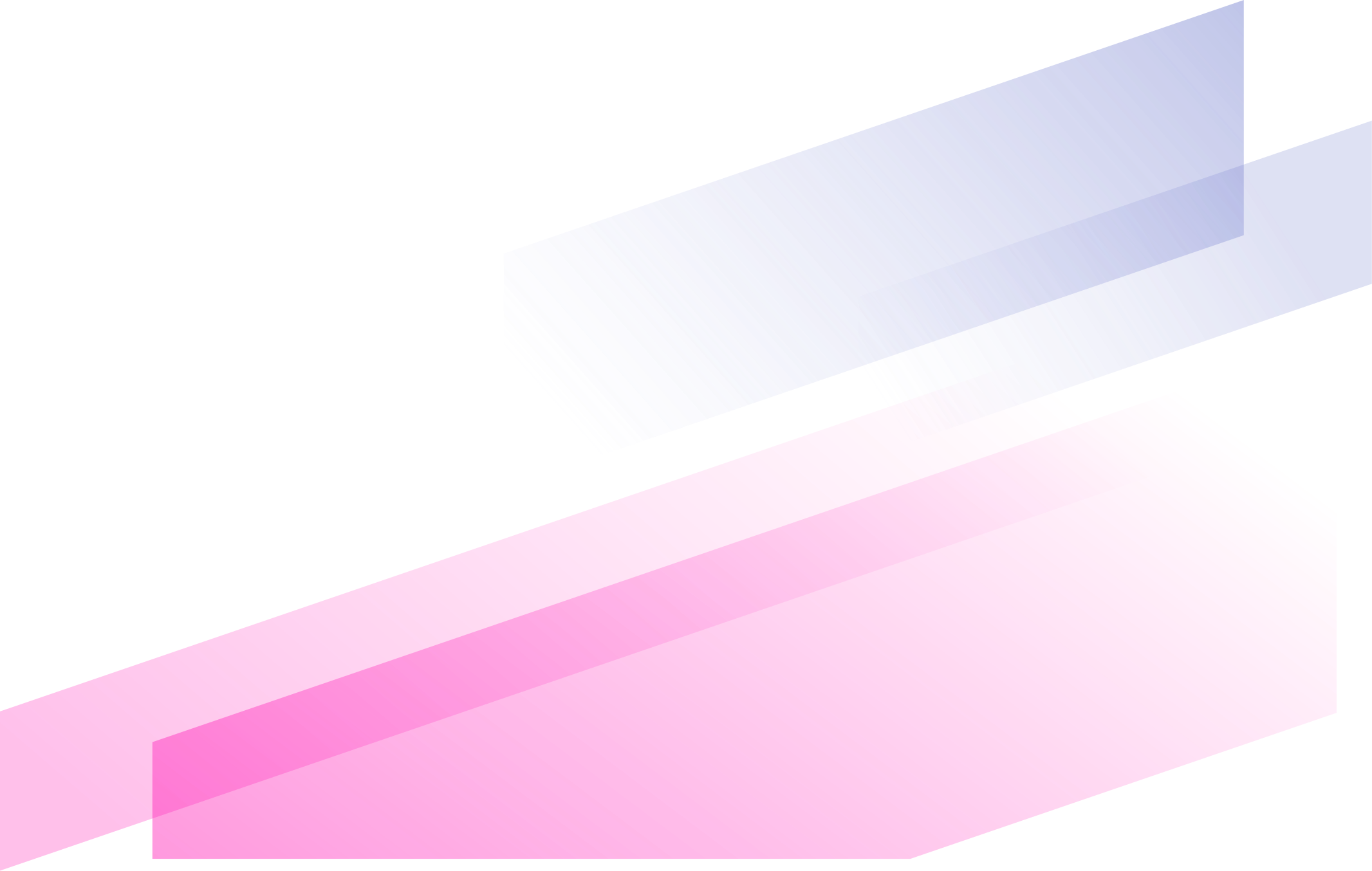
Cell Taxonomy: a curated repository of cell types with multifaceted characterization.
Multiscale Analysis and Validation of Effective Drug Combinations Targeting Driver KRAS Mutations in Non-Small Cell Lung Cancer.
The Advancement of Biodegradable Polyesters as Delivery Systems for Camptothecin and Its Analogues-A Status Report.
Rare variant analyses across multiethnic cohorts identify novel genes for refractive error.
From 'Omics to Multi-omics Technologies: the Discovery of Novel Causal Mediators.
Integrating heterogeneous knowledge graphs into drug-drug interaction extraction from the literature.
VSTH: a user-friendly web server for structure-based virtual screening on Tianhe-2.
Prediction of drug side effects with transductive matrix co-completion.
DFinder: a novel end-to-end graph embedding-based method to identify drug-food interactions.
Anti-asthmatic fraction screening and mechanisms prediction of Schisandrae Sphenantherae Fructus based on a combined approach.