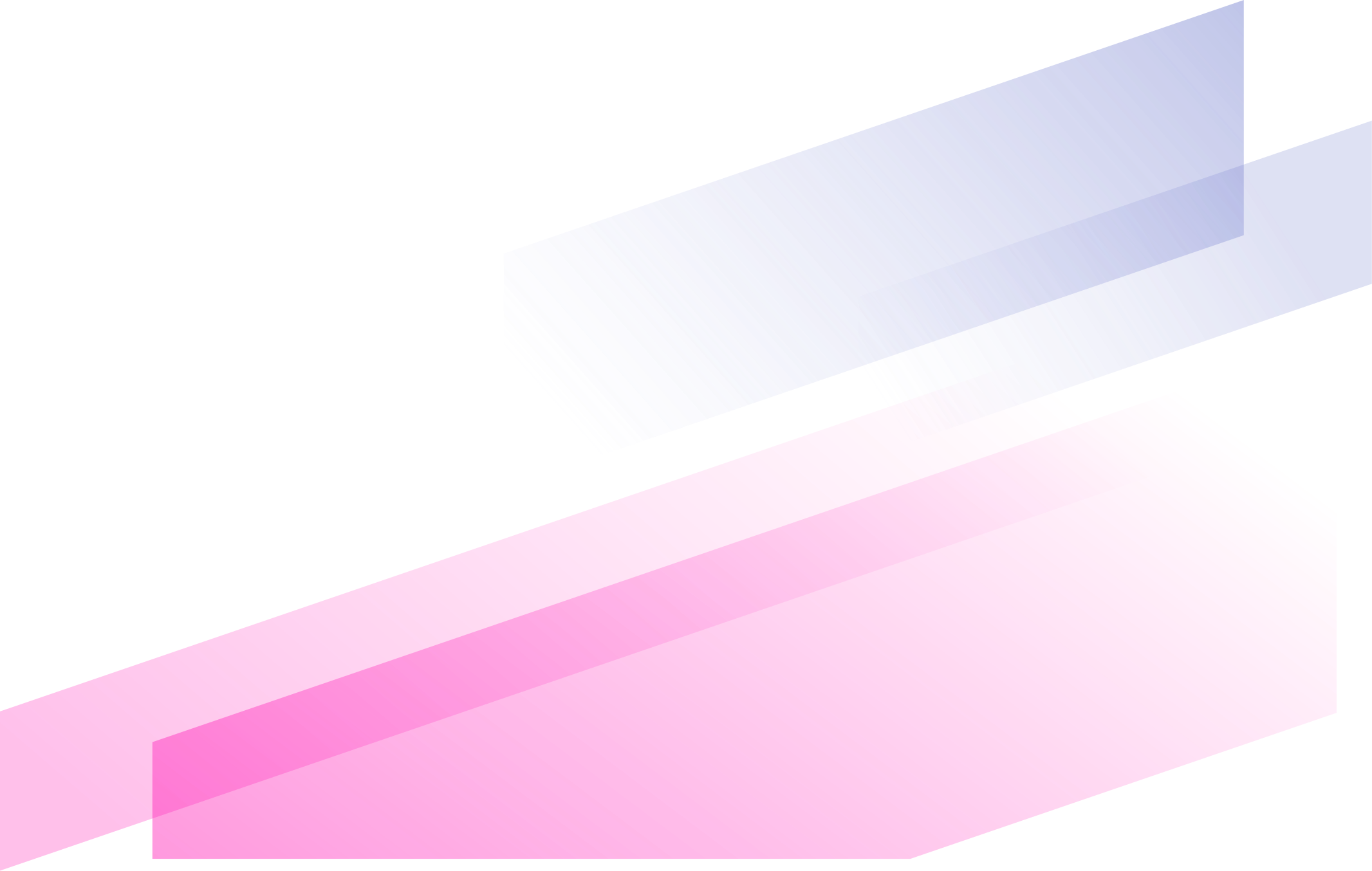
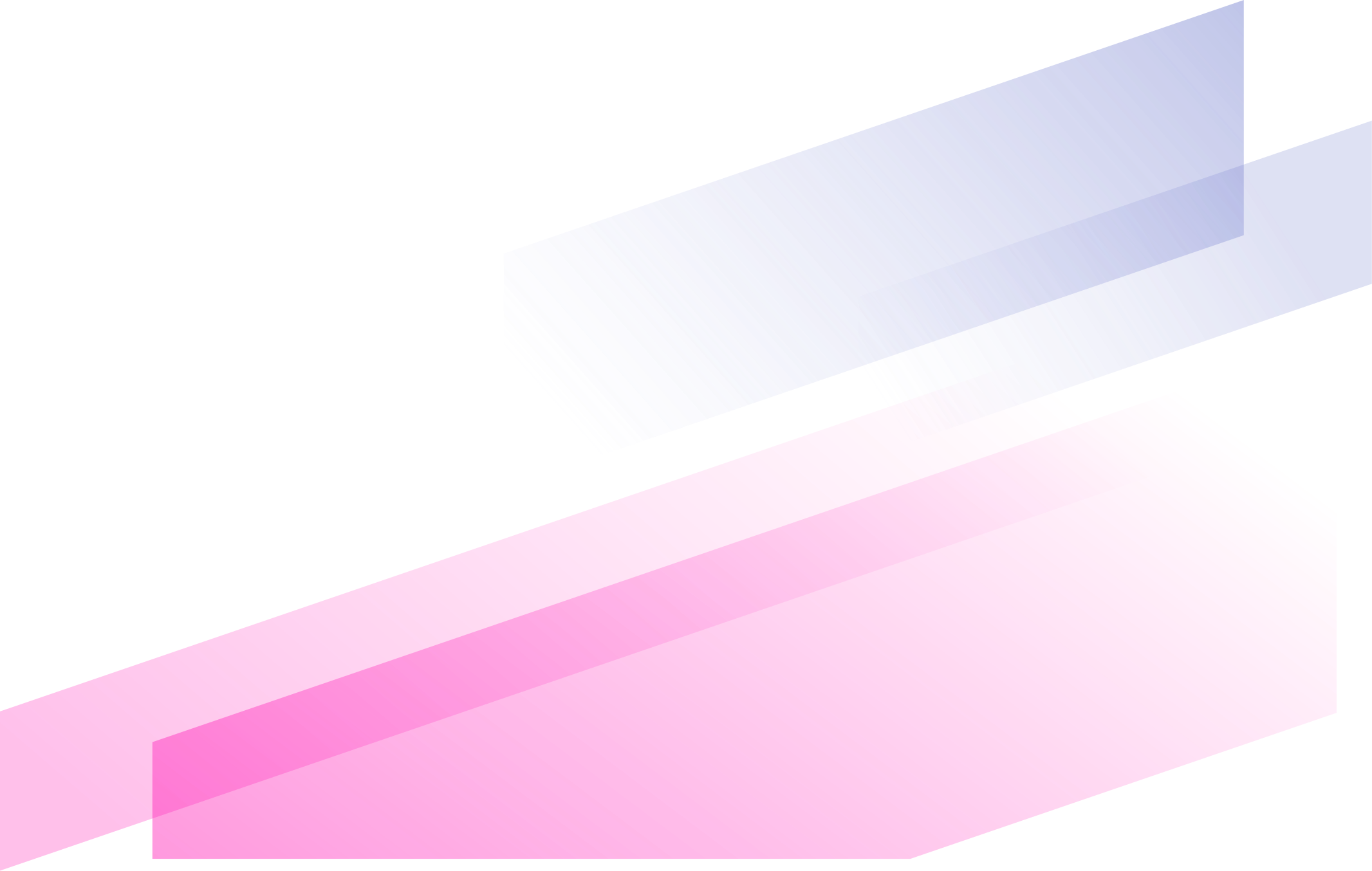
Drug triggered pruritus, rash, papules, and blisters - is AGEP a clash of an altered sphingolipid-metabolism and lysosomotropism of drugs accumulating in the skin?
Drug Interactions for Patients with Respiratory Diseases Receiving COVID-19 Emerged Treatments.
Integrative analysis of the plasma proteome and polygenic risk of cardiometabolic diseases.
Artificial intelligence for the discovery of novel antimicrobial agents for emerging infectious diseases.
A multiple network-based bioinformatics pipeline for the study of molecular mechanisms in oncological diseases for personalized medicine.
Knowledge bases and software support for variant interpretation in precision oncology.
Utilizing graph machine learning within drug discovery and development.
Artificial intelligence and machine learning methods in predicting anti-cancer drug combination effects.
Learning from low-rank multimodal representations for predicting disease-drug associations.
Towards Biomanufacturing of Cell-Derived Matrices.