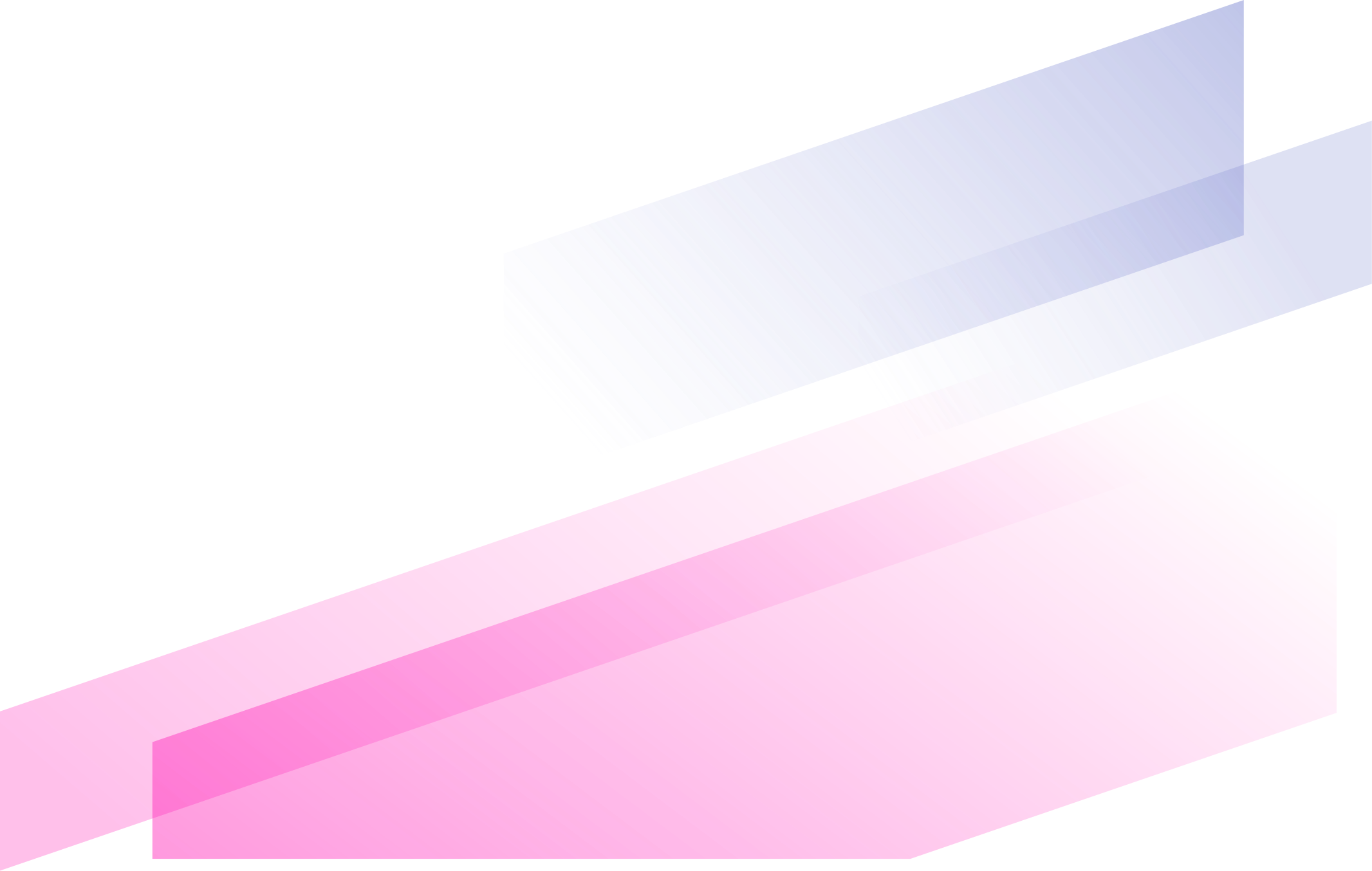
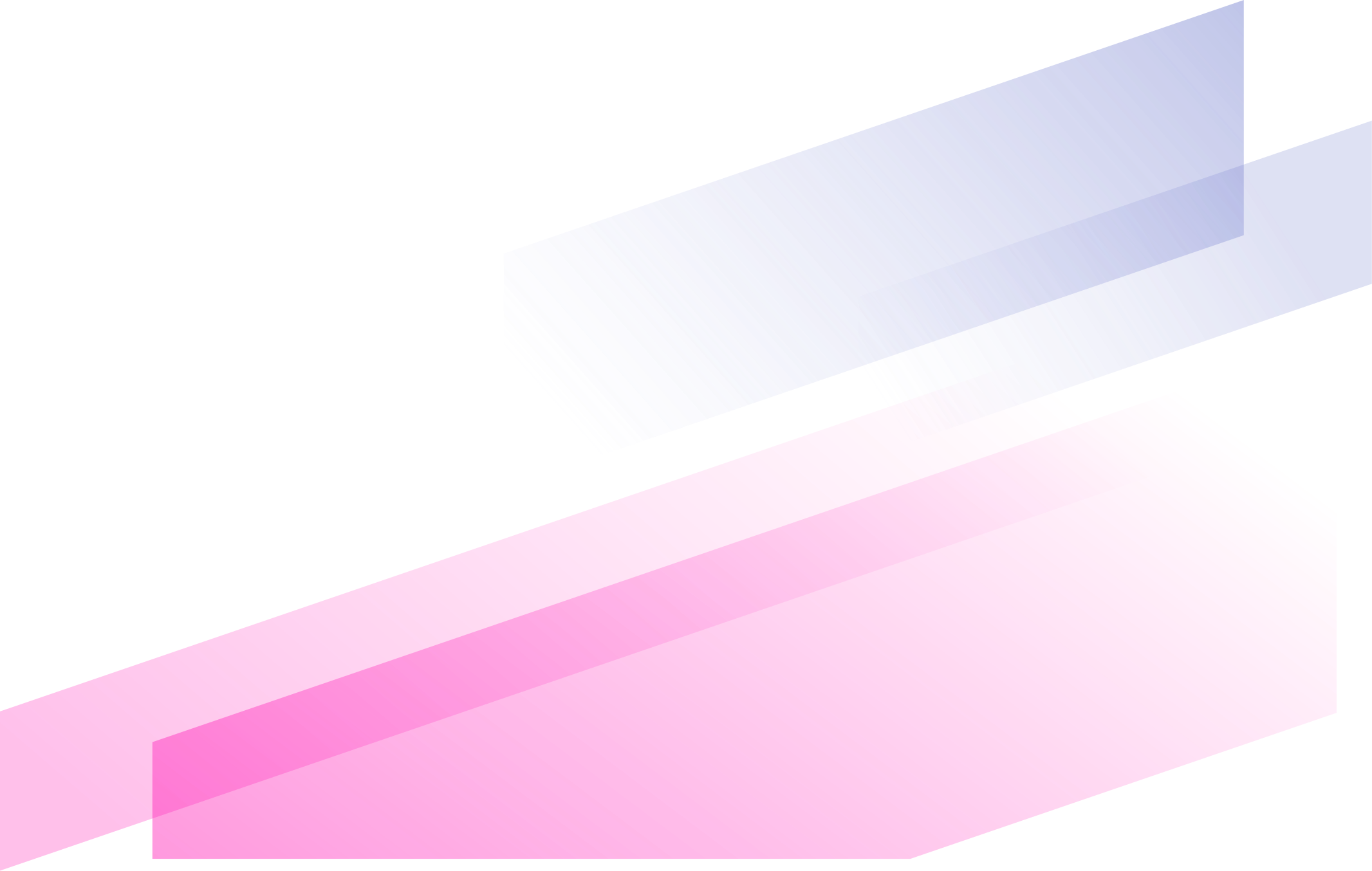
Network-driven analysis of human-Plasmodium falciparum interactome: processes for malaria drug discovery and extracting in silico targets.
Towards Drug Repurposing in Cancer Cachexia: Potential Targets and Candidates.
Target Discovery for Host-Directed Antiviral Therapies: Application of Proteomics Approaches.
Mapping Compound Databases to Disease Maps-A MINERVA Plugin for CandActBase.
Natural products for infectious microbes and diseases: an overview of sources, compounds, and chemical diversities.
Prediction of severe adverse events, modes of action and drug treatments for COVID-19's complications.
Context-aware multi-token concept recognition of biological entities.
A Maximum Flow-Based Approach to Prioritize Drugs for Drug Repurposing of Chronic Diseases.
Analysis of physicochemical properties of protein-protein interaction modulators suggests stronger alignment with the "rule of five".
Recent applications of quantitative systems pharmacology and machine learning models across diseases.