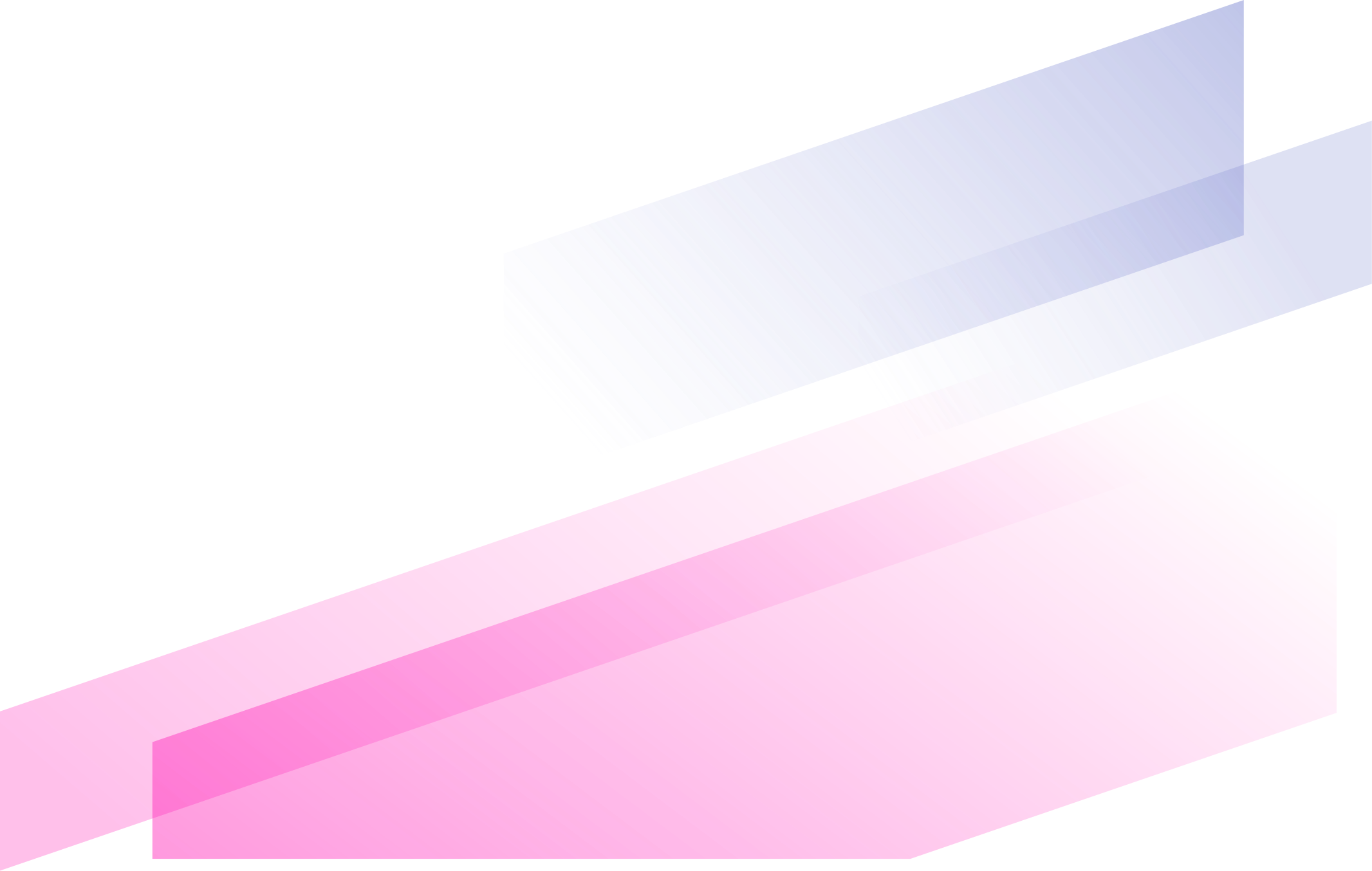
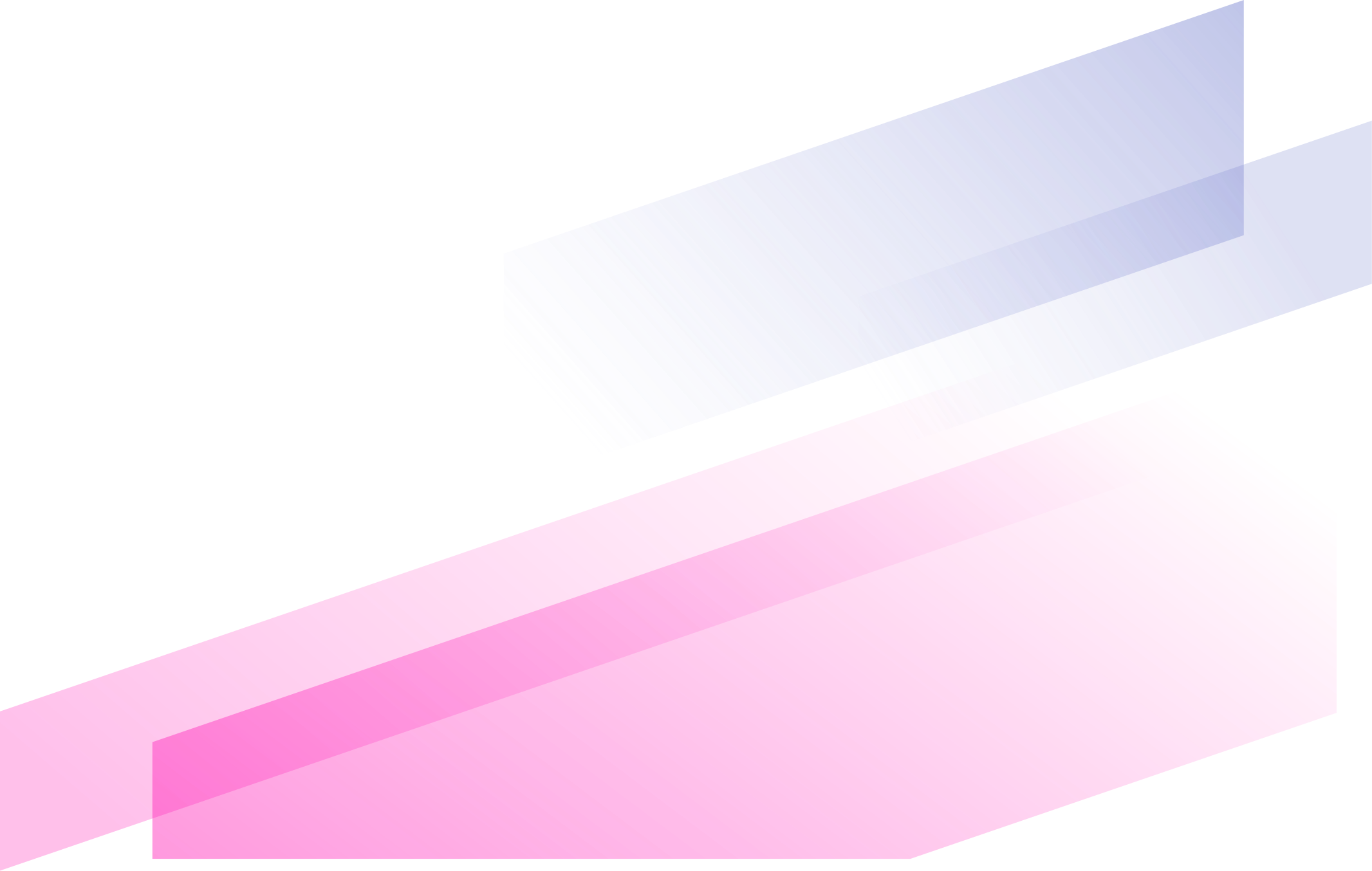
Publications Search
Explore how scientists all over the world use DrugBank in their research.
Showing 1601 to 1604 of 1625 entries
Published on July 31, 2008
Drug-therapy networks and the prediction of novel drug targets.
Abstract: A recent study in BMC Pharmacology presents a network of drugs and the therapies in which they are used. Network approaches open new ways of predicting novel drug targets and overcoming the cellular robustness that can prevent drugs from working.
Published on July 30, 2008
Distinguishing compounds with anticancer activity by ANN using inductive QSAR descriptors.
Abstract: This article describes a method developed for predicting anticancer/non-anticancer drugs using artificial neural network (ANN). The ANN used in this study is a feed-forward neural network with a standard back-propagation training algorithm. Using 30 'inductive' QSAR descriptors alone, we have been able to achieve 84.28% accuracy for correct separation of compounds with- and without anticancer activity. For the complete set of 30 inductive QSAR descriptors, ANN based method reveals a superior model (accuracy = 84.28%, Q(pred) = 74.28%, sensitivity = 0.9285, specificity = 0.7857, Matthews correlation coefficient (MCC) = 0.6998). The method was trained and tested on a non redundant data set of 380 drugs (122 anticancer and 258 non-anticancer). The elaborated QSAR model based on the Artificial Neural Networks approach has been extensively validated and has confidently assigned anticancer character to a number of trial anticancer drugs from the literature.
Published on July 24, 2008
Machine learning based analyses on metabolic networks supports high-throughput knockout screens.
Abstract: BACKGROUND: Computational identification of new drug targets is a major goal of pharmaceutical bioinformatics. RESULTS: This paper presents a machine learning strategy to study and validate essential enzymes of a metabolic network. Each single enzyme was characterized by its local network topology, gene homologies and co-expression, and flux balance analyses. A machine learning system was trained to distinguish between essential and non-essential reactions. It was validated by a comprehensive experimental dataset, which consists of the phenotypic outcomes from single knockout mutants of Escherichia coli (KEIO collection). We yielded very reliable results with high accuracy (93%) and precision (90%). We show that topologic, genomic and transcriptomic features describing the network are sufficient for defining the essentiality of a reaction. These features do not substantially depend on specific media conditions and enabled us to apply our approach also for less specific media conditions, like the lysogeny broth rich medium. CONCLUSION: Our analysis is feasible to validate experimental knockout data of high throughput screens, can be used to improve flux balance analyses and supports experimental knockout screens to define drug targets.
Published on July 1, 2008
Prediction of drug-target interaction networks from the integration of chemical and genomic spaces.
Abstract: MOTIVATION: The identification of interactions between drugs and target proteins is a key area in genomic drug discovery. Therefore, there is a strong incentive to develop new methods capable of detecting these potential drug-target interactions efficiently. RESULTS: In this article, we characterize four classes of drug-target interaction networks in humans involving enzymes, ion channels, G-protein-coupled receptors (GPCRs) and nuclear receptors, and reveal significant correlations between drug structure similarity, target sequence similarity and the drug-target interaction network topology. We then develop new statistical methods to predict unknown drug-target interaction networks from chemical structure and genomic sequence information simultaneously on a large scale. The originality of the proposed method lies in the formalization of the drug-target interaction inference as a supervised learning problem for a bipartite graph, the lack of need for 3D structure information of the target proteins, and in the integration of chemical and genomic spaces into a unified space that we call 'pharmacological space'. In the results, we demonstrate the usefulness of our proposed method for the prediction of the four classes of drug-target interaction networks. Our comprehensively predicted drug-target interaction networks enable us to suggest many potential drug-target interactions and to increase research productivity toward genomic drug discovery. AVAILABILITY: Softwares are available upon request. SUPPLEMENTARY INFORMATION: Datasets and all prediction results are available at http://web.kuicr.kyoto-u.ac.jp/supp/yoshi/drugtarget/.
Published on July 1, 2008
MADNet: microarray database network web server.
Abstract: MADNet is a user-friendly data mining and visualization tool for rapid analysis of diverse high-throughput biological data such as microarray, phage display or even metagenome experiments. It presents biological information in the context of metabolic and signalling pathways, transcription factors and drug targets through minimal user input, consisting only of the file with the experimental data. These data are integrated with information stored in various biological databases such as NCBI nucleotide and protein databases, metabolic and signalling pathway databases (KEGG), transcription regulation (TRANSFAC(c)) and drug target database (DrugBank). MADNet is freely available for academic use at http://www.bioinfo.hr/madnet.
Published on March 4, 2008
A global view of drug-therapy interactions.
Abstract: BACKGROUND: Network science is already making an impact on the study of complex systems and offers a promising variety of tools to understand their formation and evolution in many disparate fields from technological networks to biological systems. Even though new high-throughput technologies have rapidly been generating large amounts of genomic data, drug design has not followed the same development, and it is still complicated and expensive to develop new single-target drugs. Nevertheless, recent approaches suggest that multi-target drug design combined with a network-dependent approach and large-scale systems-oriented strategies create a promising framework to combat complex multi-genetic disorders like cancer or diabetes. RESULTS: We here investigate the human network corresponding to the interactions between all US approved drugs and human therapies, defined by known relationships between drugs and their therapeutic applications. Our results show that the average paths in this drug-therapy network are shorter than three steps, indicating that distant therapies are separated by a surprisingly low number of chemical compounds. We also identify a sub-network composed by drugs with high centrality measures in the drug-therapy network, which represent the structural backbone of this system and act as hubs routing information between distant parts of the network. CONCLUSION: These findings provide for the first time a global map of the large-scale organization of all known drugs and associated therapies, bringing new insights on possible strategies for future drug development. Special attention should be given to drugs which combine the two properties of (a) having a high centrality value in the drug-therapy network and (b) acting on multiple molecular targets in the human system.
Published in February 2008
Quantitative systems-level determinants of human genes targeted by successful drugs.
Abstract: What makes a successful drug target? A target molecule with an appropriate (druggable) tertiary structure is a necessary but not the sufficient condition for success. Here we analyzed specific properties of human genes and proteins targeted by 919 FDA-approved drugs and identified several quantitative measures that distinguish them from other genes and proteins at a highly significant level. Compared to an average gene and its encoded protein(s), successful drug targets are more highly connected (but far from being the most highly connected), have higher betweenness values, lower entropies of tissue expression, and lower ratios of nonsynonymous to synonymous single-nucleotide polymorphisms. Furthermore, we have identified human tissues that are significantly over- or undertargeted relative to the full spectrum of genes that are active in each tissue. Our study provides quantitative guidelines that could aid in the computational screening of new drug targets in human cells.
Published in January 2008
SuperTarget and Matador: resources for exploring drug-target relationships.
Abstract: The molecular basis of drug action is often not well understood. This is partly because the very abundant and diverse information generated in the past decades on drugs is hidden in millions of medical articles or textbooks. Therefore, we developed a one-stop data warehouse, SuperTarget that integrates drug-related information about medical indication areas, adverse drug effects, drug metabolization, pathways and Gene Ontology terms of the target proteins. An easy-to-use query interface enables the user to pose complex queries, for example to find drugs that target a certain pathway, interacting drugs that are metabolized by the same cytochrome P450 or drugs that target the same protein but are metabolized by different enzymes. Furthermore, we provide tools for 2D drug screening and sequence comparison of the targets. The database contains more than 2500 target proteins, which are annotated with about 7300 relations to 1500 drugs; the vast majority of entries have pointers to the respective literature source. A subset of these drugs has been annotated with additional binding information and indirect interactions and is available as a separate resource called Matador. SuperTarget and Matador are available at http://insilico.charite.de/supertarget and http://matador.embl.de.
Published in January 2008
GLIDA: GPCR--ligand database for chemical genomics drug discovery--database and tools update.
Abstract: G-protein coupled receptors (GPCRs) represent one of the most important families of drug targets in pharmaceutical development. GLIDA is a public GPCR-related Chemical Genomics database that is primarily focused on the integration of information between GPCRs and their ligands. It provides interaction data between GPCRs and their ligands, along with chemical information on the ligands, as well as biological information regarding GPCRs. These data are connected with each other in a relational database, allowing users in the field of Chemical Genomics research to easily retrieve such information from either biological or chemical starting points. GLIDA includes a variety of similarity search functions for the GPCRs and for their ligands. Thus, GLIDA can provide correlation maps linking the searched homologous GPCRs (or ligands) with their ligands (or GPCRs). By analyzing the correlation patterns between GPCRs and ligands, we can gain more detailed knowledge about their conserved molecular recognition patterns and improve drug design efforts by focusing on inferred candidates for GPCR-specific drugs. This article provides a summary of the GLIDA database and user facilities, and describes recent improvements to database design, data contents, ligand classification programs, similarity search options and graphical interfaces. GLIDA is publicly available at http://pharminfo.pharm.kyoto-u.ac.jp/services/glida/. We hope that it will prove very useful for Chemical Genomics research and GPCR-related drug discovery.
Published in January 2008
PROCOGNATE: a cognate ligand domain mapping for enzymes.
Abstract: PROCOGNATE is a database of protein cognate ligands for the domains in enzyme structures as described by CATH, SCOP and Pfam, and is available as an interactive website or a flat file. This article gives an overview of the database and its generation and presents a new website front end, as well as recent increased coverage in our dataset via inclusion of Pfam domains. We also describe navigation of the website and its features. The current version (1.3) of PROCOGNATE covers 4123, 4536, 5876 structures and 377, 326, 695 superfamilies/families in CATH, SCOP and Pfam, respectively. PROCOGNATE can be accessed at: http://www.ebi.ac.uk/thornton-srv/databases/procognate/
Showing 1601 to 1604 of 1625 entries