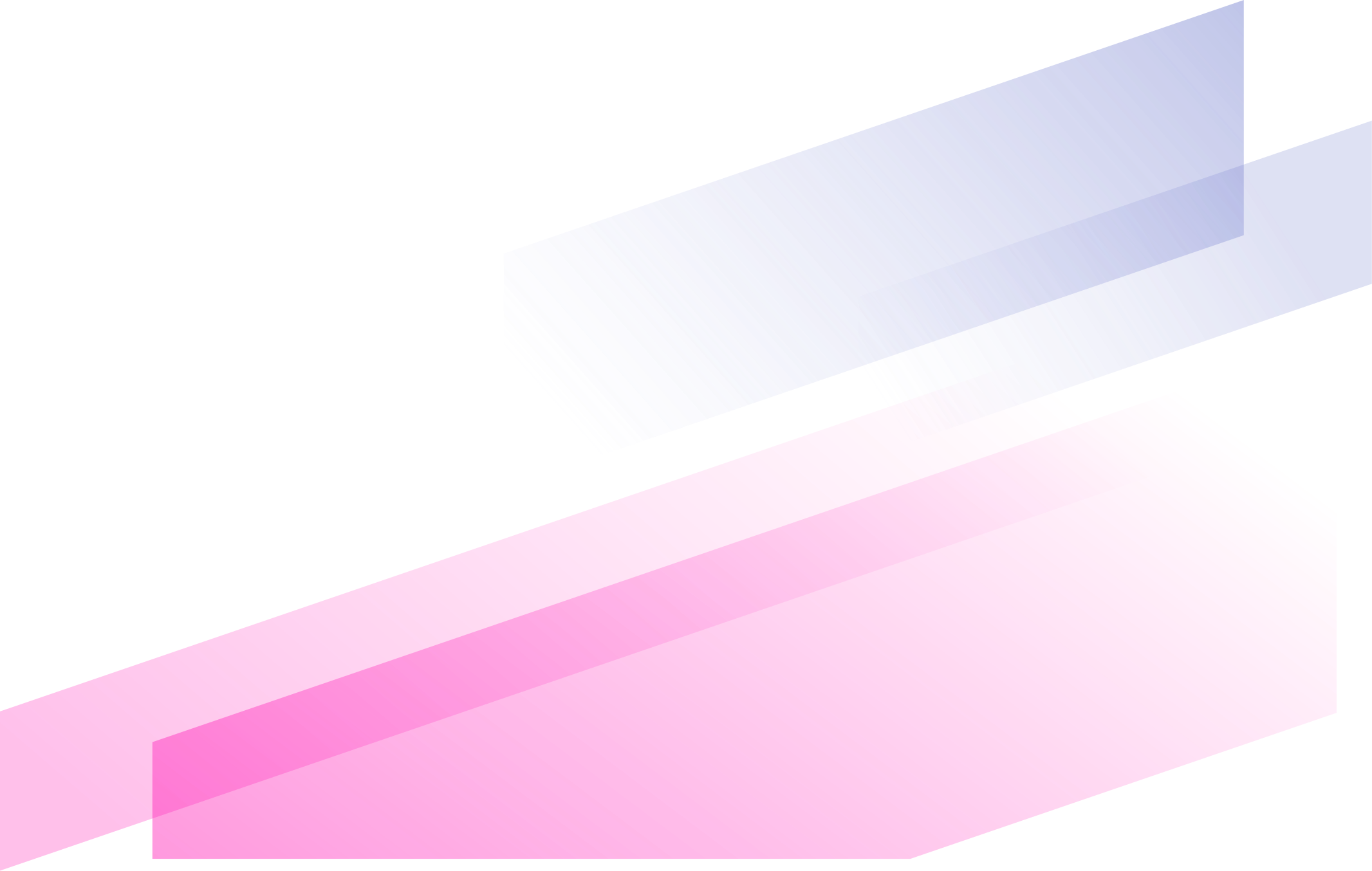
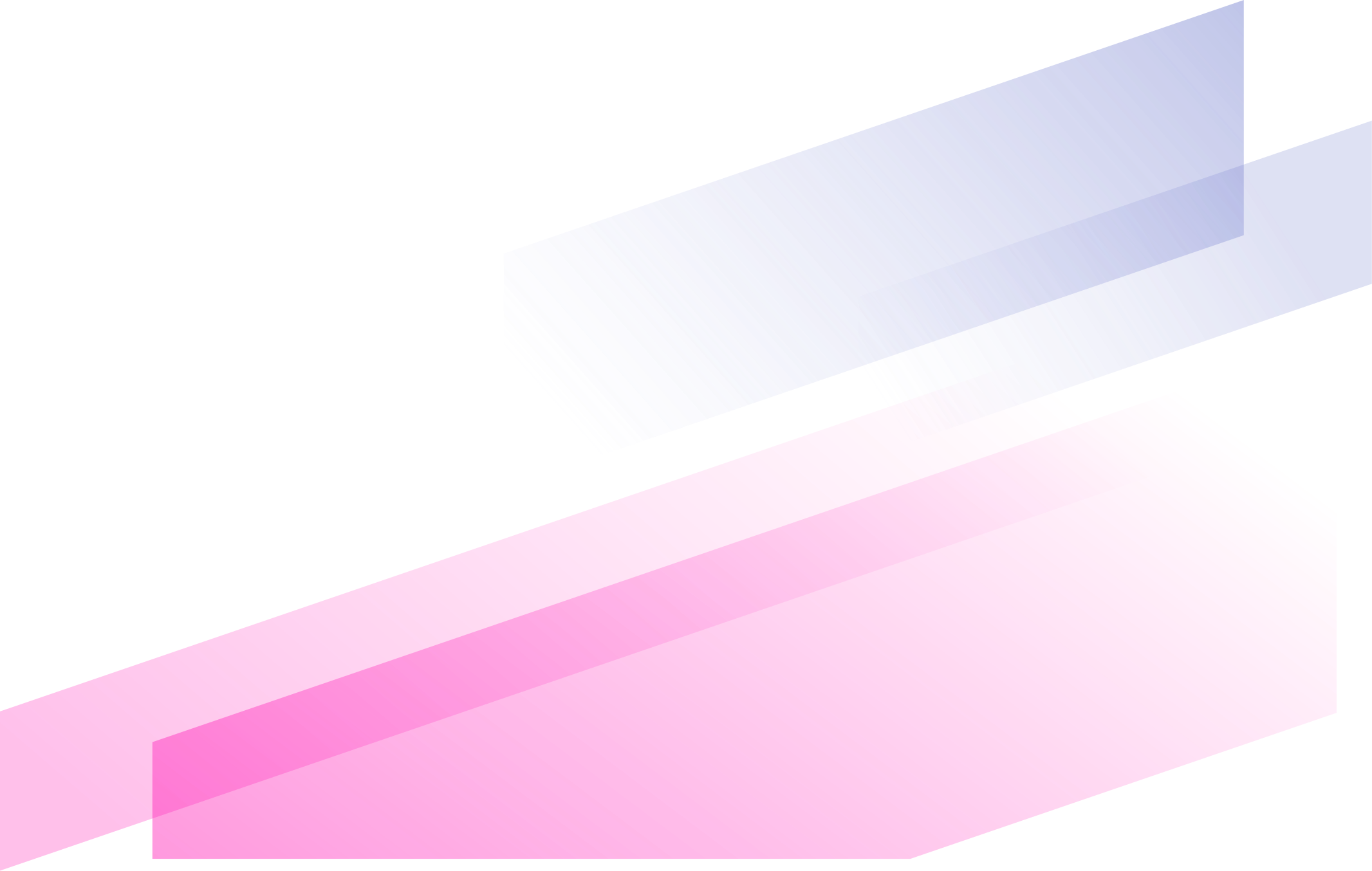
Node Similarity Based Graph Convolution for Link Prediction in Biological Networks.
Systems pharmacogenomics identifies novel targets and clinically actionable therapeutics for medulloblastoma.
Natural Phytochemicals Derived from Gymnosperms in the Prevention and Treatment of Cancers.
Predicting Drug-Target Interactions Based on the Ensemble Models of Multiple Feature Pairs.
MolDiscovery: learning mass spectrometry fragmentation of small molecules.
External Evaluation of Two Pediatric Population Pharmacokinetics Models of Oral Trimethoprim and Sulfamethoxazole.
Meropenem Pharmacokinetics and Target Attainment in Critically Ill Patients Are Not Affected by Extracorporeal Membrane Oxygenation: A Matched Cohort Analysis.
A structural deep network embedding model for predicting associations between miRNA and disease based on molecular association network.
Discovery of a Novel Non-Narcotic Analgesic Derived from the CL-20 Explosive: Synthesis, Pharmacology, and Target Identification of Thiowurtzine, a Potent Inhibitor of the Opioid Receptors and the Ion Channels.
Genome-wide discovery of hidden genes mediating known drug-disease association using KDDANet.