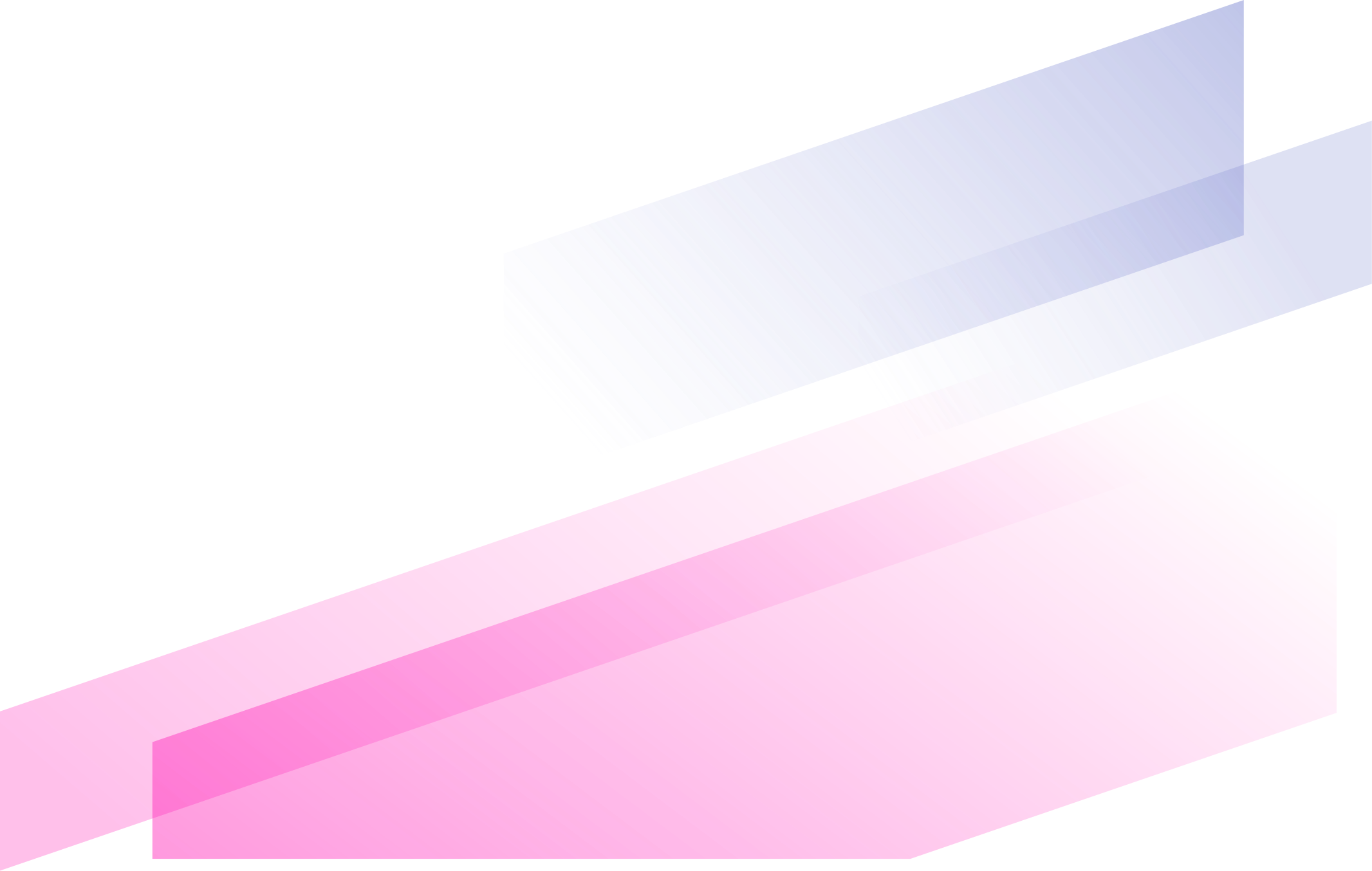
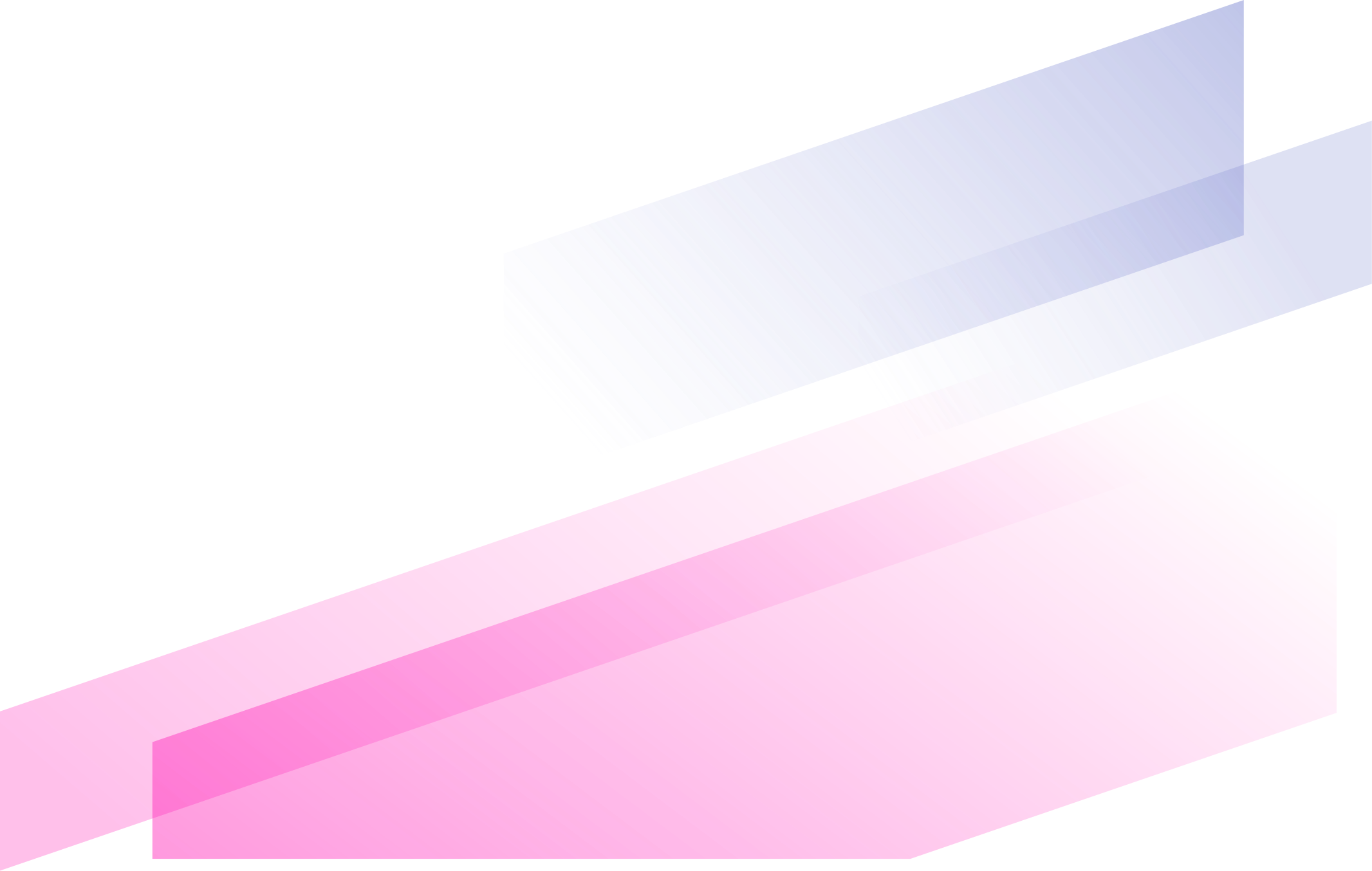
Prediction of Side Effects Using Comprehensive Similarity Measures.
Automated recognition of functional compound-protein relationships in literature.
PHARMIP: An insilico method to predict genetics that underpin adverse drug reactions.
In-silico simulated prototype-patients using TPMS technology to study a potential adverse effect of sacubitril and valsartan.
Zein nanoparticles as nontoxic delivery system for maytansine in the treatment of non-small cell lung cancer.
Towards FAIR protocols and workflows: the OpenPREDICT use case.
Predicting Tumor Cell Response to Synergistic Drug Combinations Using a Novel Simplified Deep Learning Model.
Flavonoids mitigate neurodegeneration in aged Caenorhabditis elegans by mitochondrial uncoupling.
Drug-Target Interaction Network Analysis of Gene-Phenotype Connectivity Maintained by Genistein.
A New Metric Quantifying Chemical and Biological Property of Small Molecule Metabolites and Drugs.