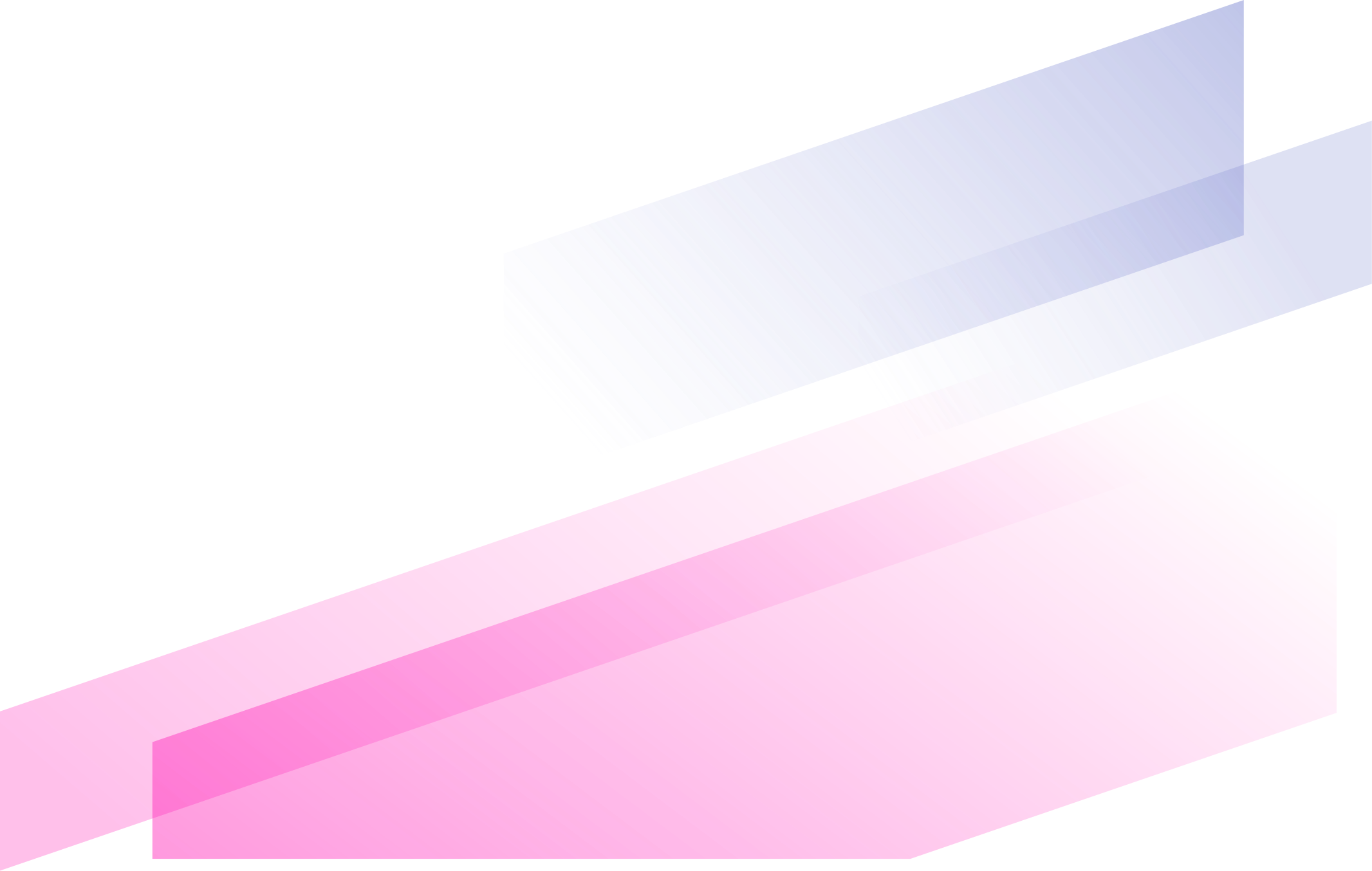
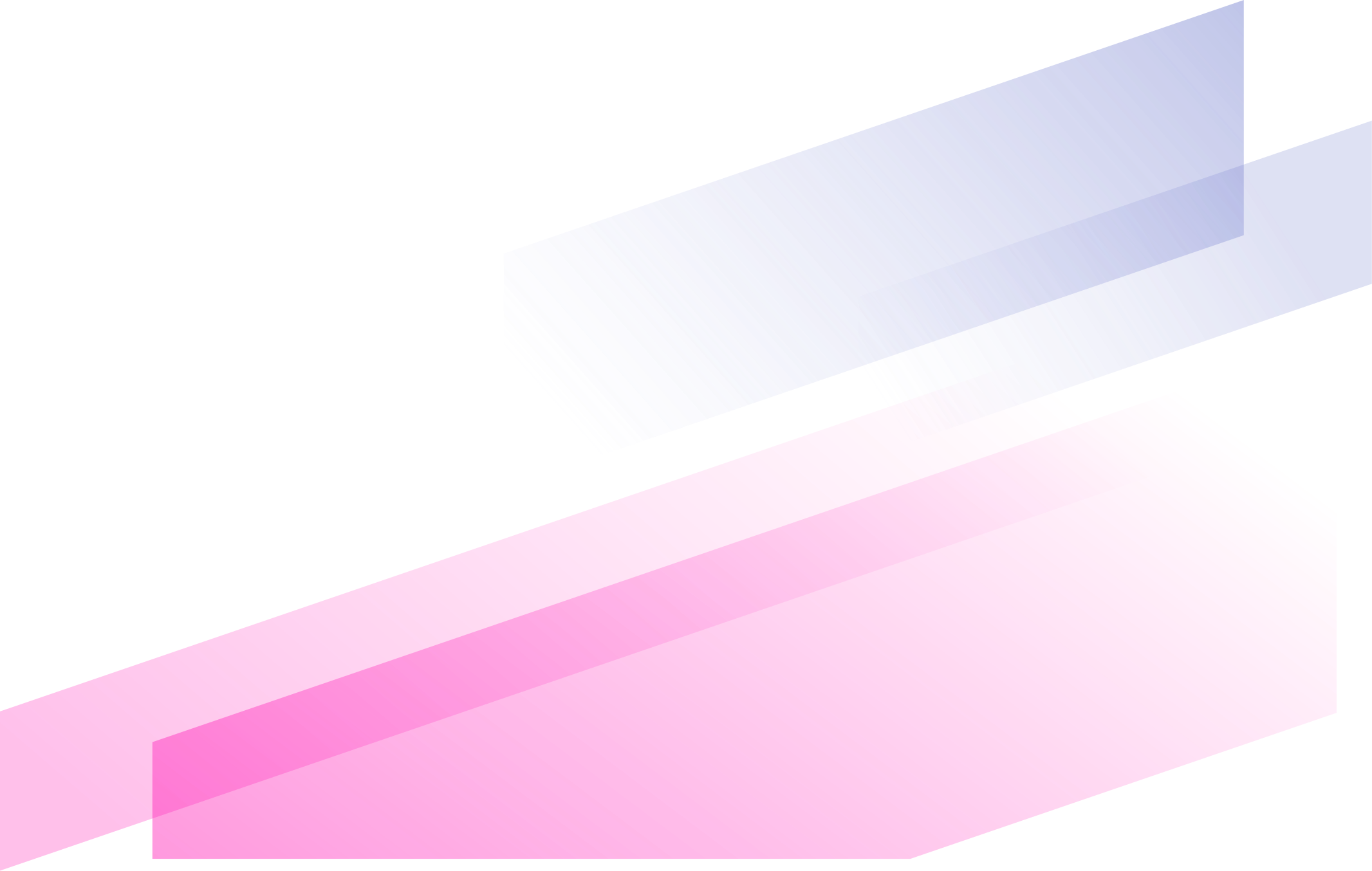
The Role of PARP1 in Monocyte and Macrophage Commitment and Specification: Future Perspectives and Limitations for the Treatment of Monocyte and Macrophage Relevant Diseases with PARP Inhibitors.
Multiomics dissection of molecular regulatory mechanisms underlying autoimmune-associated noncoding SNPs.
Targeting the SARS-CoV-2 main protease using FDA-approved Isavuconazonium, a P2-P3 alpha-ketoamide derivative and Pentagastrin: An in-silico drug discovery approach.
Delta-9-Tetrahydrocannabinol and Cannabidiol Drug-Drug Interactions.
A machine learning and network framework to discover new indications for small molecules.
Genomic landscape of the immune microenvironments of brain metastases in breast cancer.
Supportive care medications coinciding with chemotherapy among children with hematologic malignancy.
Predicting novel drugs for SARS-CoV-2 using machine learning from a >10 million chemical space.
Investigating Core Signaling Pathways of Hepatitis B Virus Pathogenesis for Biomarkers Identification and Drug Discovery via Systems Biology and Deep Learning Method.
Predictive features of gene expression variation reveal mechanistic link with differential expression.