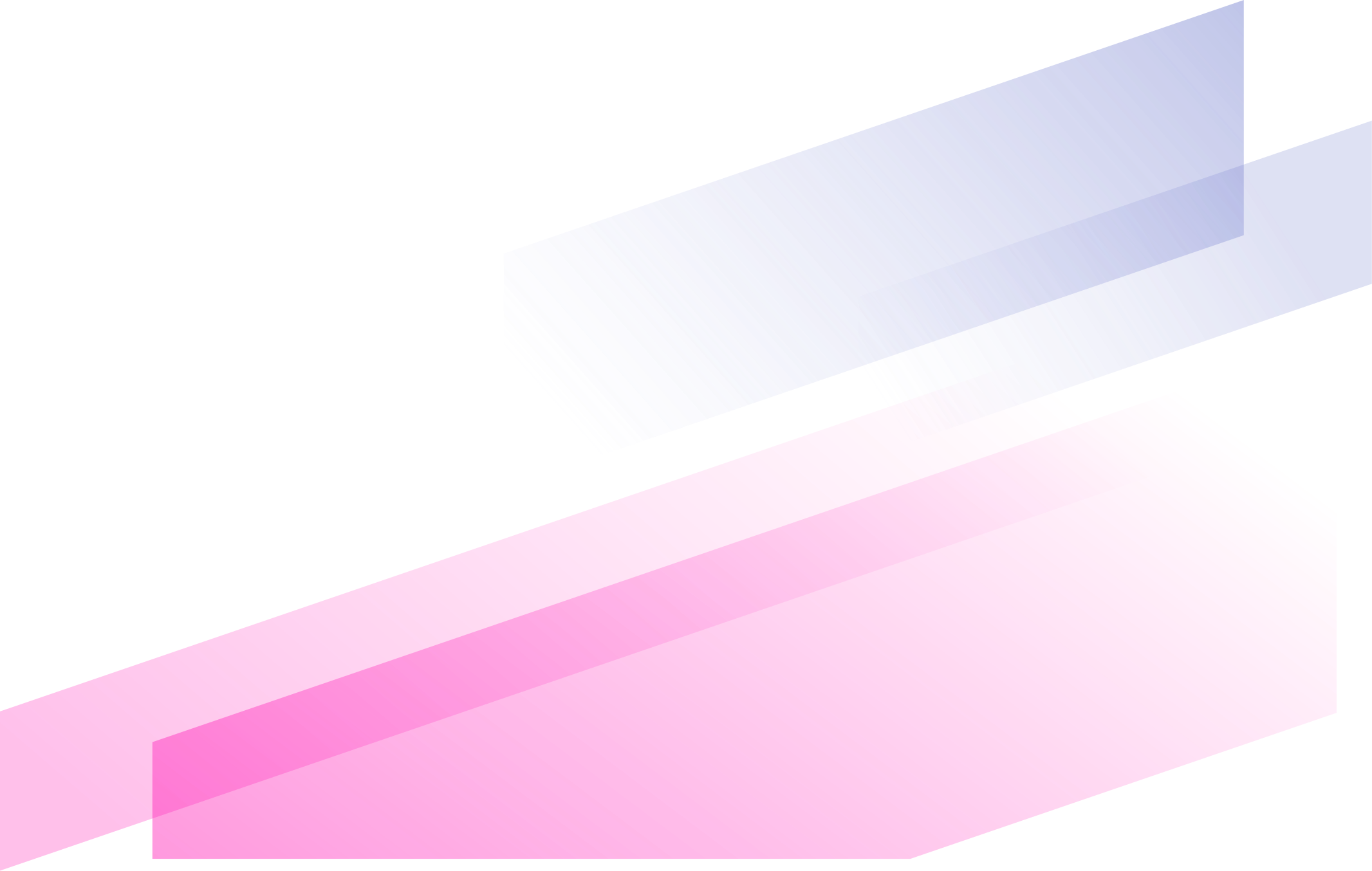
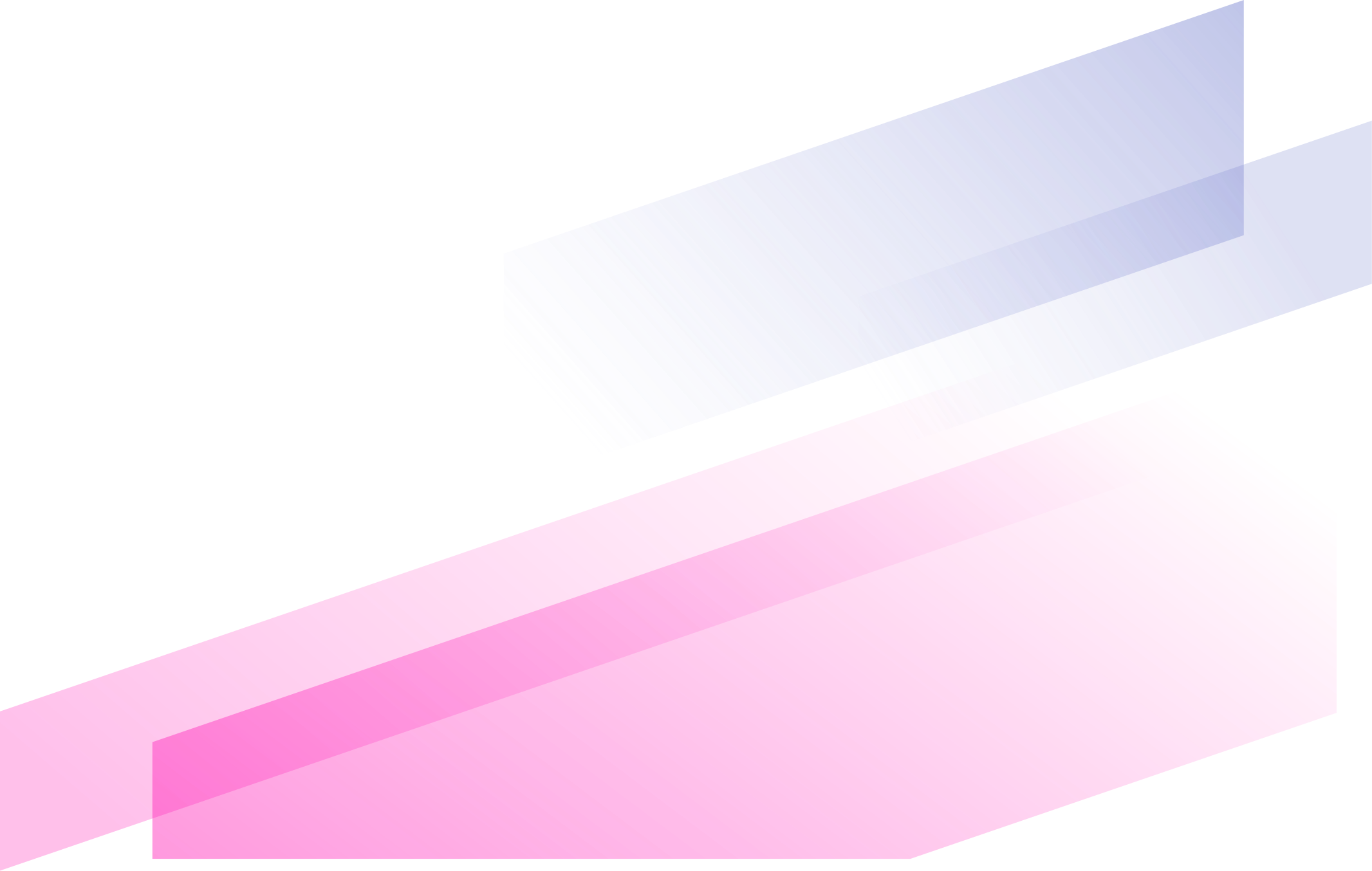
Physiologically Based Pharmacokinetic Modelling to Describe the Pharmacokinetics of Risperidone and 9-Hydroxyrisperidone According to Cytochrome P450 2D6 Phenotypes.
Evidence-Based Network Approach to Recommending Targeted Cancer Therapies.
A practical view of fine-mapping and gene prioritization in the post-genome-wide association era.
Small molecule screening identifies inhibitors of the Epstein-Barr virus deubiquitinating enzyme, BPLF1.
Gastric cancer during pregnancy: A report on 13 cases and review of the literature with focus on chemotherapy during pregnancy.
Bioinformatics for Renal and Urinary Proteomics: Call for Aggrandization.
Tamoxifen induces fatty liver disease in breast cancer through the MAPK8/FoxO pathway.
Optimal doses of antidepressants in dependence on age: Combined covariate actions in Bayesian network meta-analysis.
Tissue-guided LASSO for prediction of clinical drug response using preclinical samples.
Prediction of Drug-Drug Interactions by Using Profile Fingerprint Vectors and Protein Similarities.