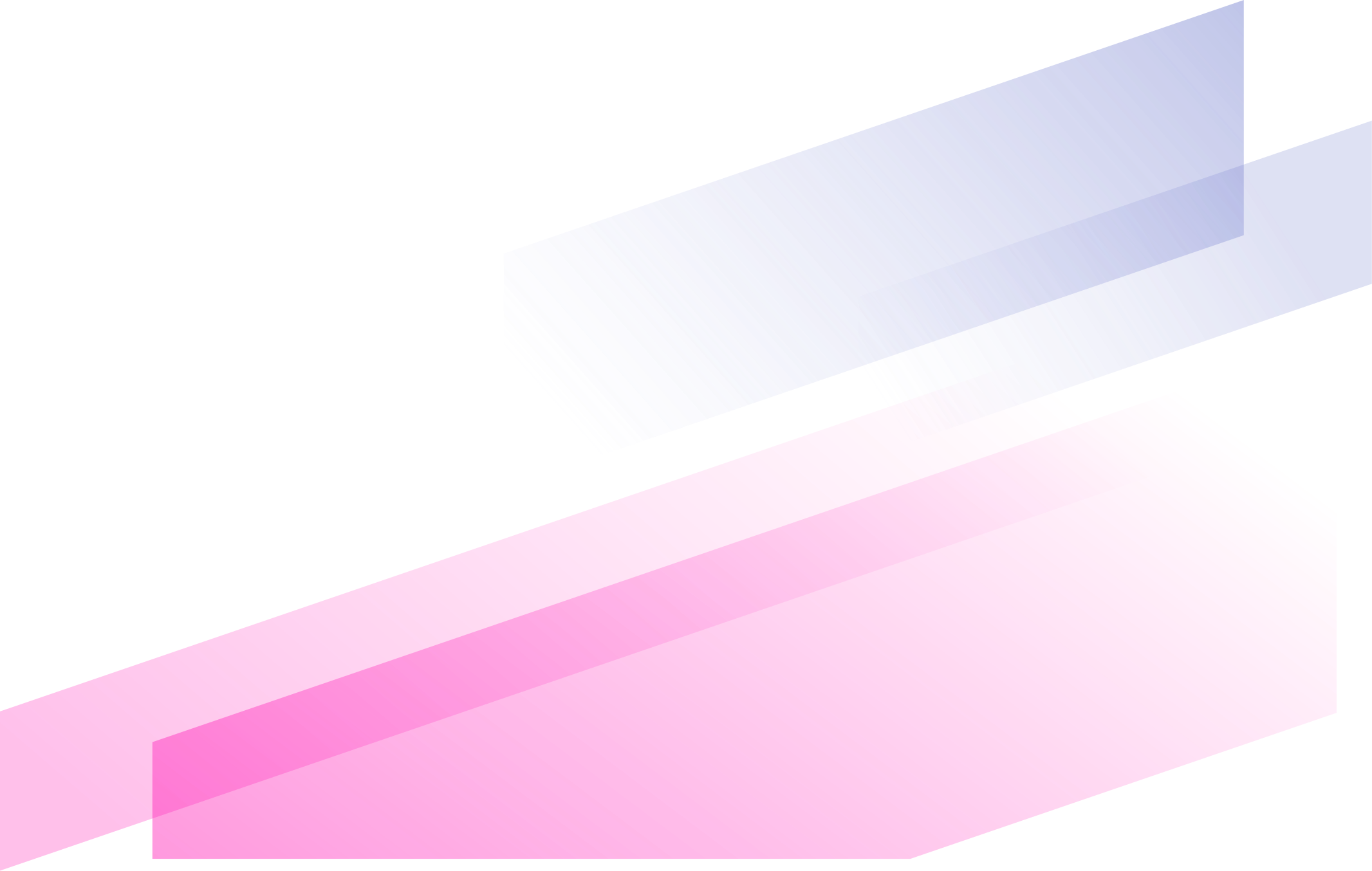
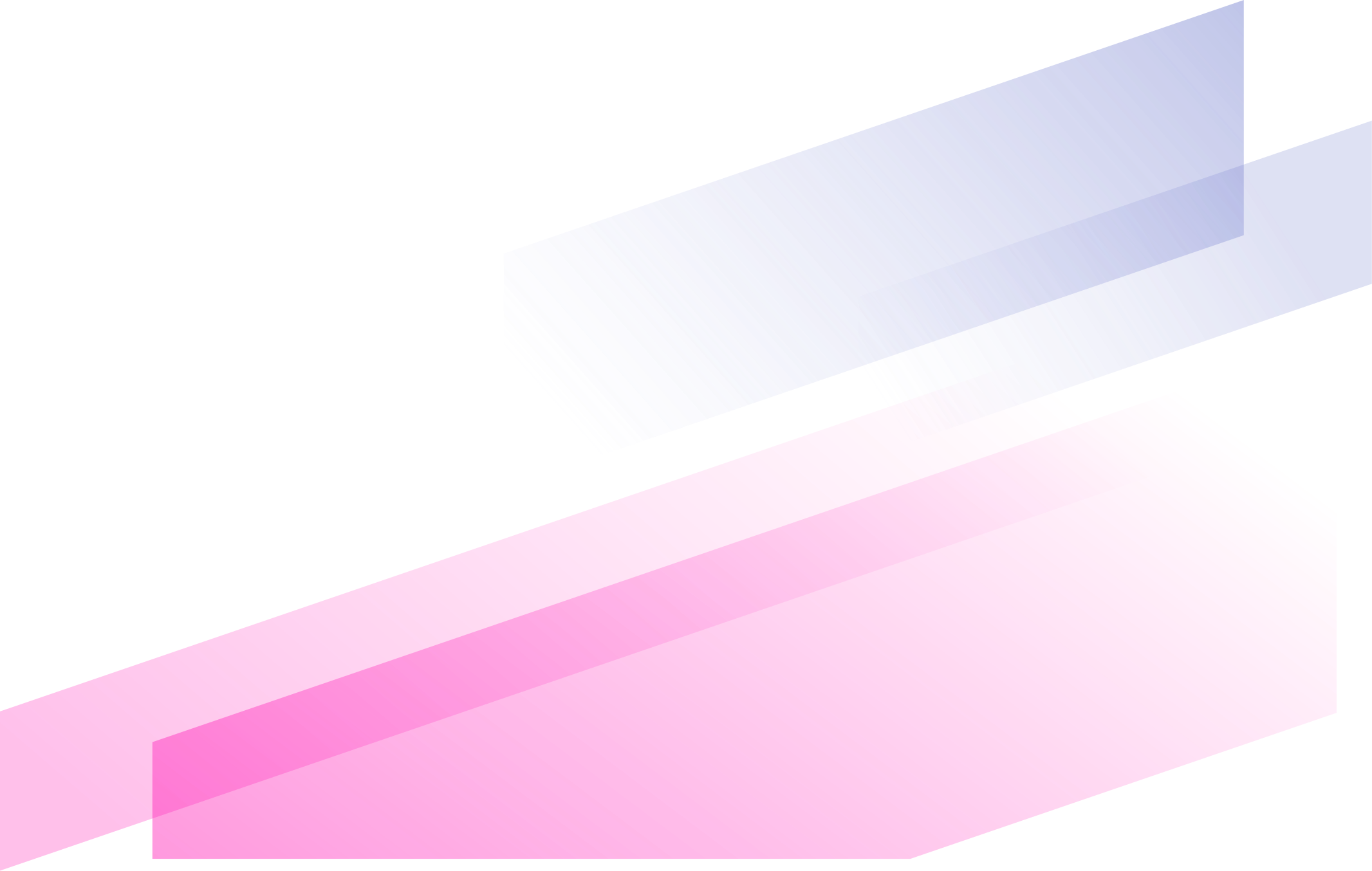
miR-9 Upregulation Integrates Post-ischemic Neuronal Survival and Regeneration In Vitro.
Bi-submodular Optimization (BSMO) for Detecting Drug-Drug Interactions (DDIs) from On-line Health Forums.
Mutations in gliclazide-associated genes may predict poor bladder cancer prognosis.
A genome-wide association study of shared risk across psychiatric disorders implicates gene regulation during fetal neurodevelopment.
Understanding Human-Virus Protein-Protein Interactions Using a Human Protein Complex-Based Analysis Framework.
Prediction of drug-disease associations based on ensemble meta paths and singular value decomposition.
Structure-Based in Silico Screening Identifies a Potent Ebolavirus Inhibitor from a Traditional Chinese Medicine Library.
Encircling the regions of the pharmacogenomic landscape that determine drug response.
Deep Learning-Based Prediction of Drug-Induced Cardiotoxicity.
Prediction of Membrane Permeation of Drug Molecules by Combining an Implicit Membrane Model with Machine Learning.