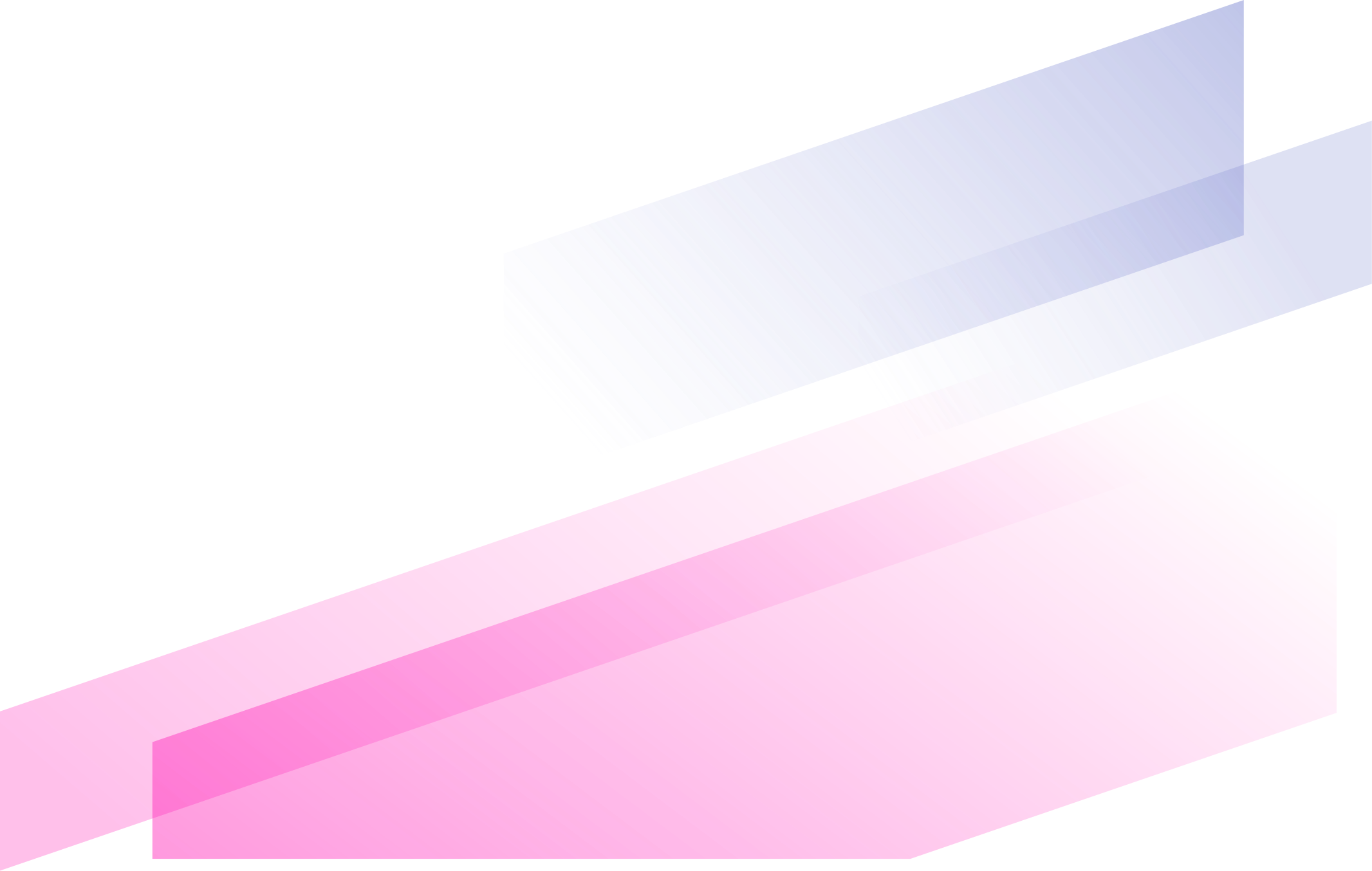
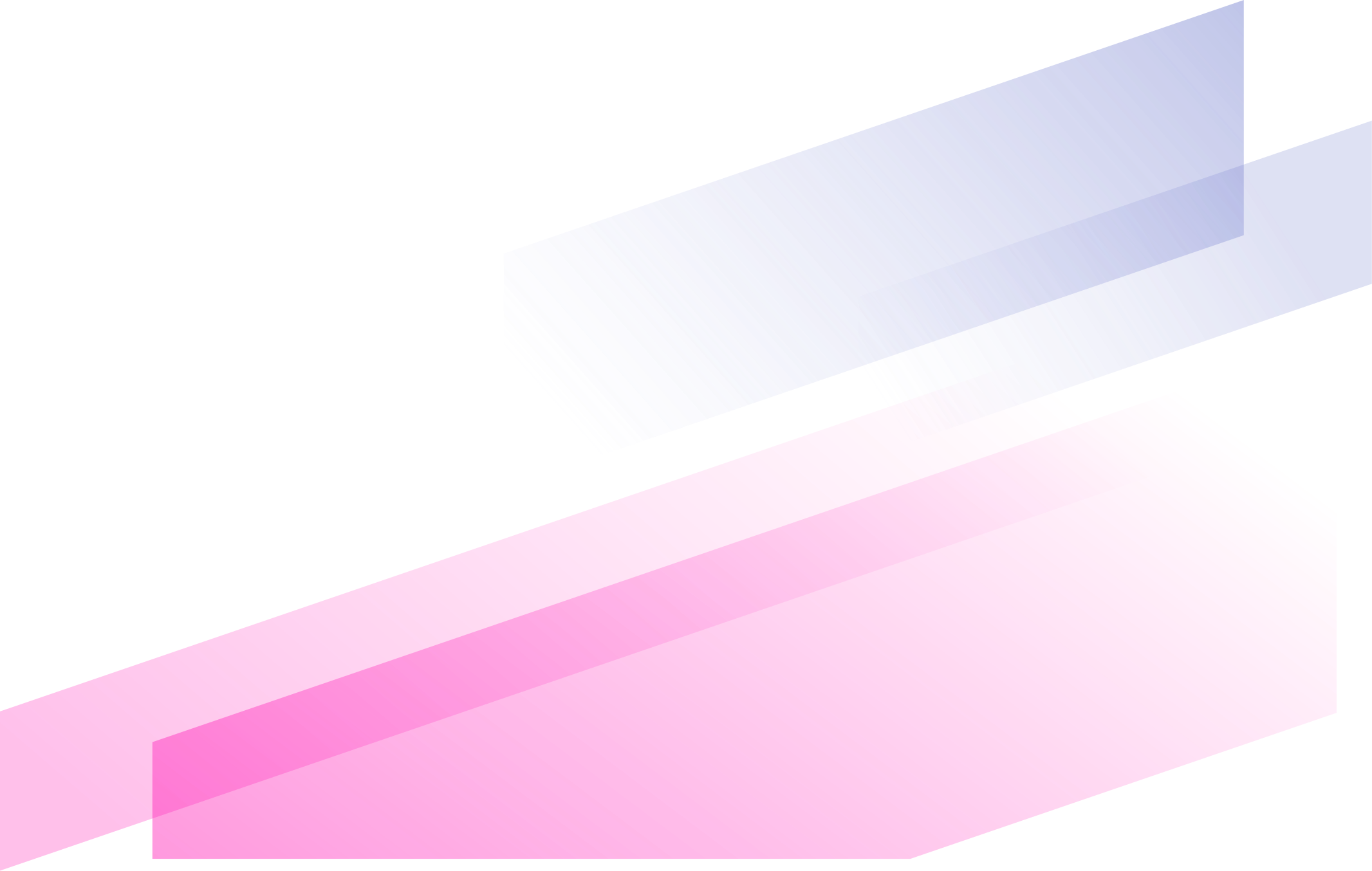
Community-driven roadmap for integrated disease maps.
Beyond the Flavour: The Potential Druggability of Chemosensory G Protein-Coupled Receptors.
Selective Estrogen Receptor Modulators Enhance CNS Remyelination Independent of Estrogen Receptors.
Adverse Event Circumstances and the Case of Drug Interactions.
Impact of Polypharmacy on Candidate Biomarker miRNomes for the Diagnosis of Fibromyalgia and Myalgic Encephalomyelitis/Chronic Fatigue Syndrome: Striking Back on Treatments.
A Platform of Synthetic Lethal Gene Interaction Networks Reveals that the GNAQ Uveal Melanoma Oncogene Controls the Hippo Pathway through FAK.
Long Noncoding RNA and Protein Interactions: From Experimental Results to Computational Models Based on Network Methods.
Study of serious adverse drug reactions using FDA-approved drug labeling and MedDRA.
KMR: knowledge-oriented medicine representation learning for drug-drug interaction and similarity computation.
Network-based prediction of drug combinations.