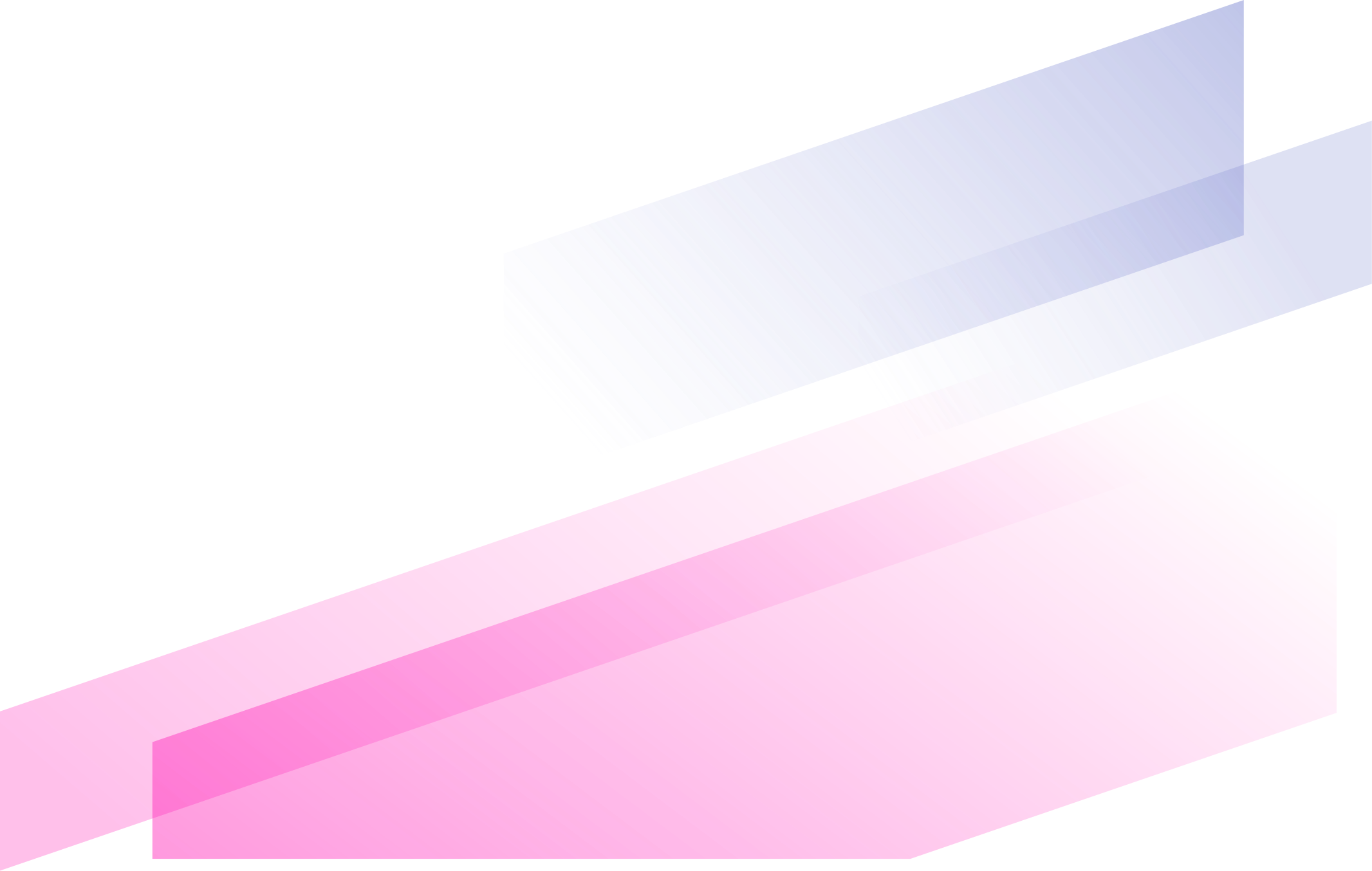
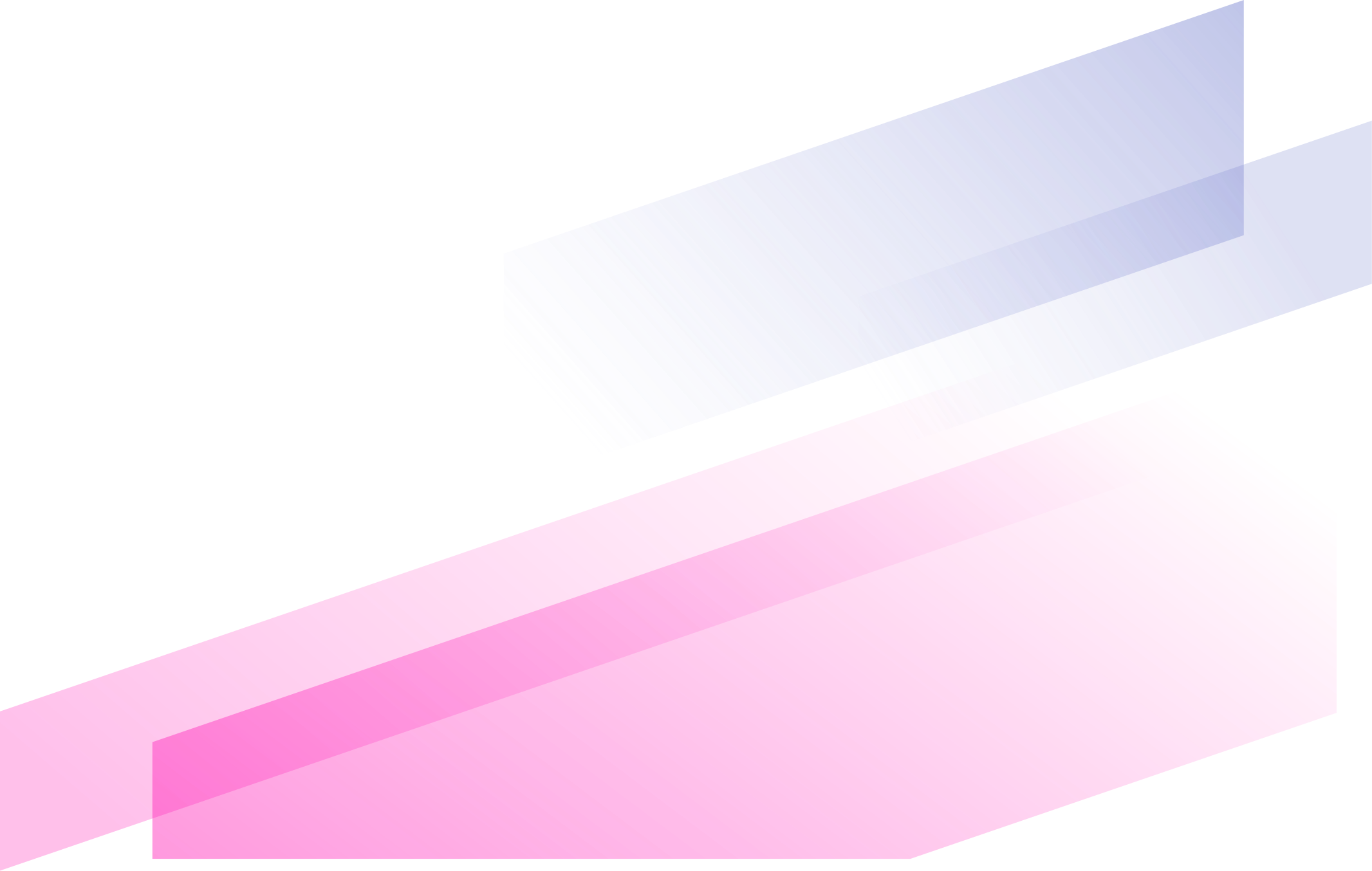
Onset timing of statin-induced musculoskeletal adverse events and concomitant drug-associated shift in onset timing of MAEs.
Using Machine Learning to Predict Synergistic Antimalarial Compound Combinations With Novel Structures.
In silico approach in reveal traditional medicine plants pharmacological material basis.
Docking-based inverse virtual screening: methods, applications, and challenges.
An analysis of aging-related genes derived from the Genotype-Tissue Expression project (GTEx).
DeCoST: A New Approach in Drug Repurposing From Control System Theory.
Exploring the Potential of Direct-To-Consumer Genomic Test Data for Predicting Adverse Drug Events.
Induction of anaplastic lymphoma kinase (ALK) as a novel mechanism of EGFR inhibitor resistance in head and neck squamous cell carcinoma patient-derived models.
Conserved Disease Modules Extracted From Multilayer Heterogeneous Disease and Gene Networks for Understanding Disease Mechanisms and Predicting Disease Treatments.
Predicting protein targets for drug-like compounds using transcriptomics.