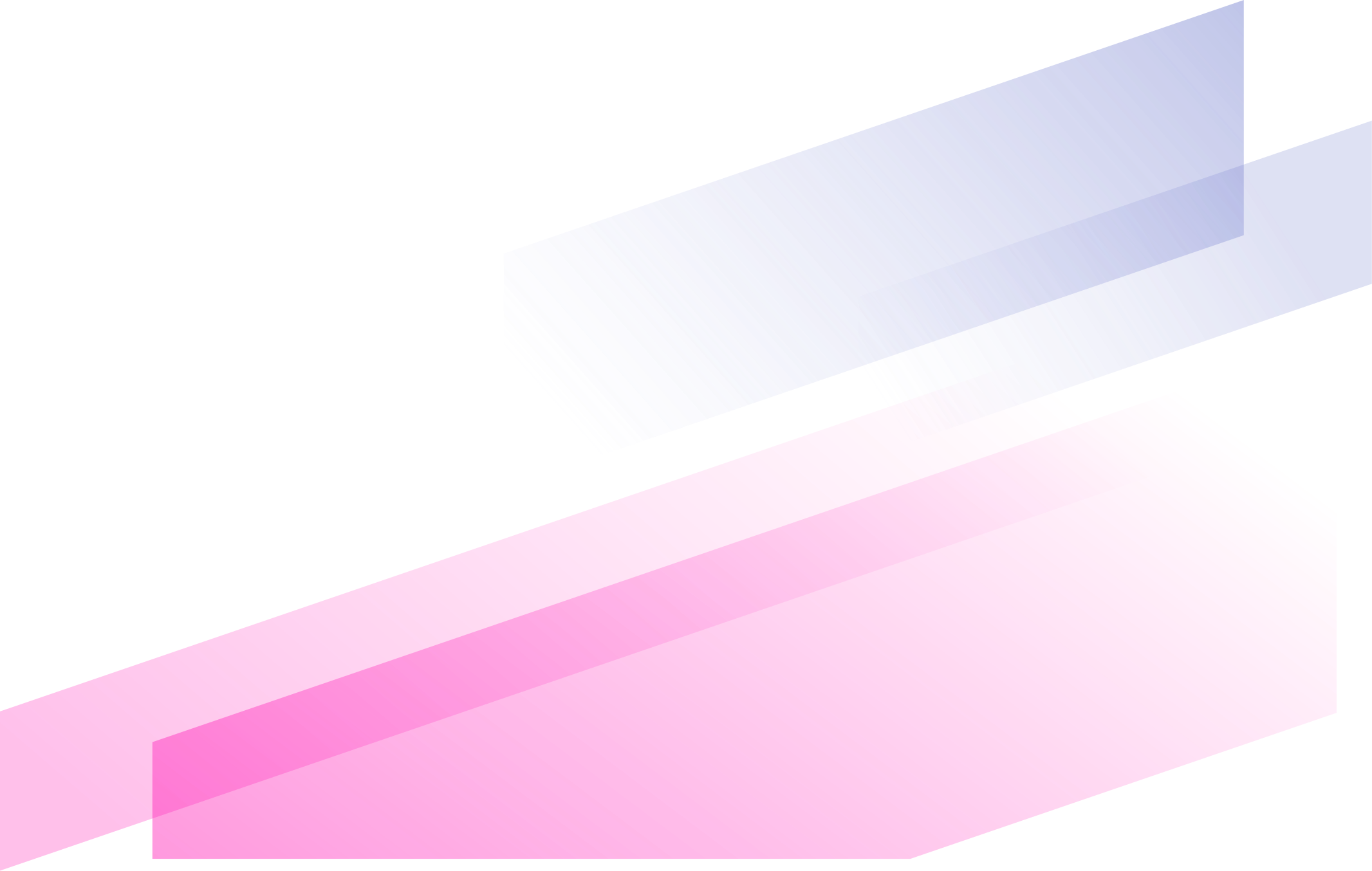
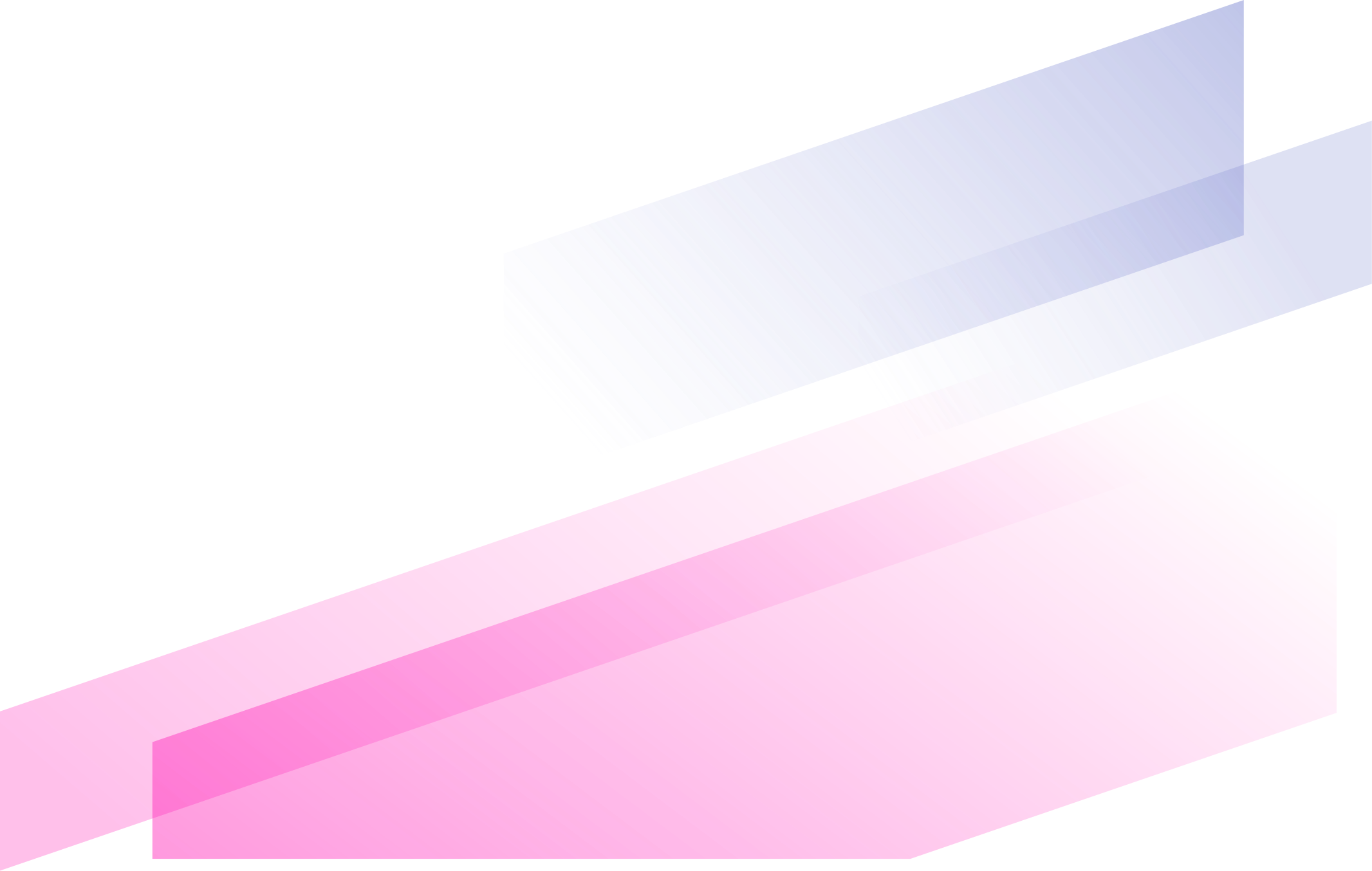
SARS-CoV-2 Non-structural protein 1(NSP1) mutation virulence and natural selection: Evolutionary trends in the six continents.
Proteogenomic characterization of MiT family translocation renal cell carcinoma.
A weighted non-negative matrix factorization approach to predict potential associations between drug and disease.
Introducing ligand GA, a genetic algorithm molecular tool for automated protein inhibitor design.
Molecular mechanism of QH-BJ drug pair in the treatment of systemic lupus erythematosus based on network pharmacology and molecular docking.
A network pharmacology study of mechanism and efficacy of Jiawei Huanglian-Wendan decoction in polycystic ovary syndrome with insulin resistance.
Inhibition of mutant RAS-RAF interaction by mimicking structural and dynamic properties of phosphorylated RAS.
RLFDDA: a meta-path based graph representation learning model for drug-disease association prediction.
Bioinformatics roadmap for therapy selection in cancer genomics.
Artificial intelligence and machine learning in pain research: a data scientometric analysis.