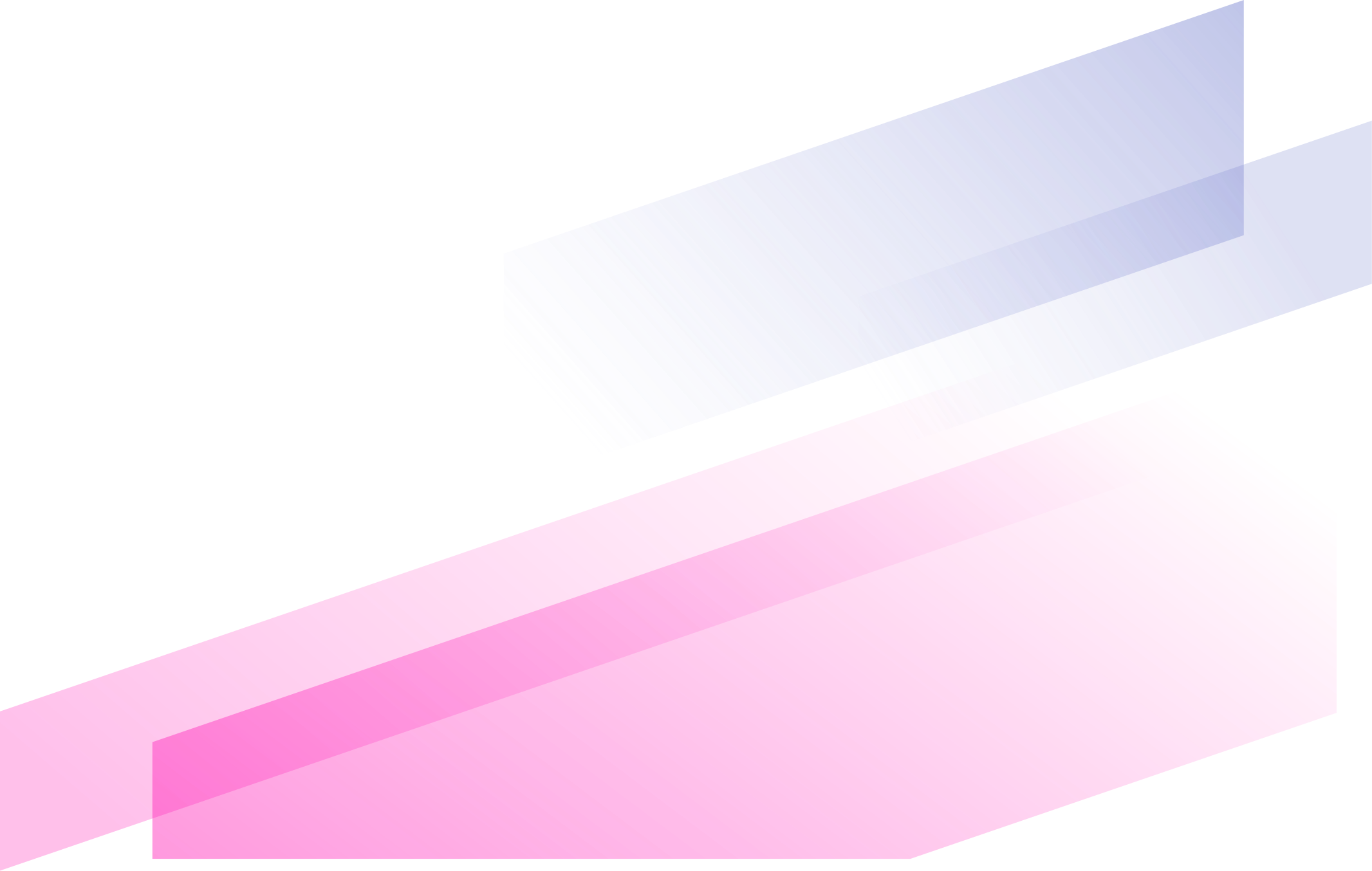
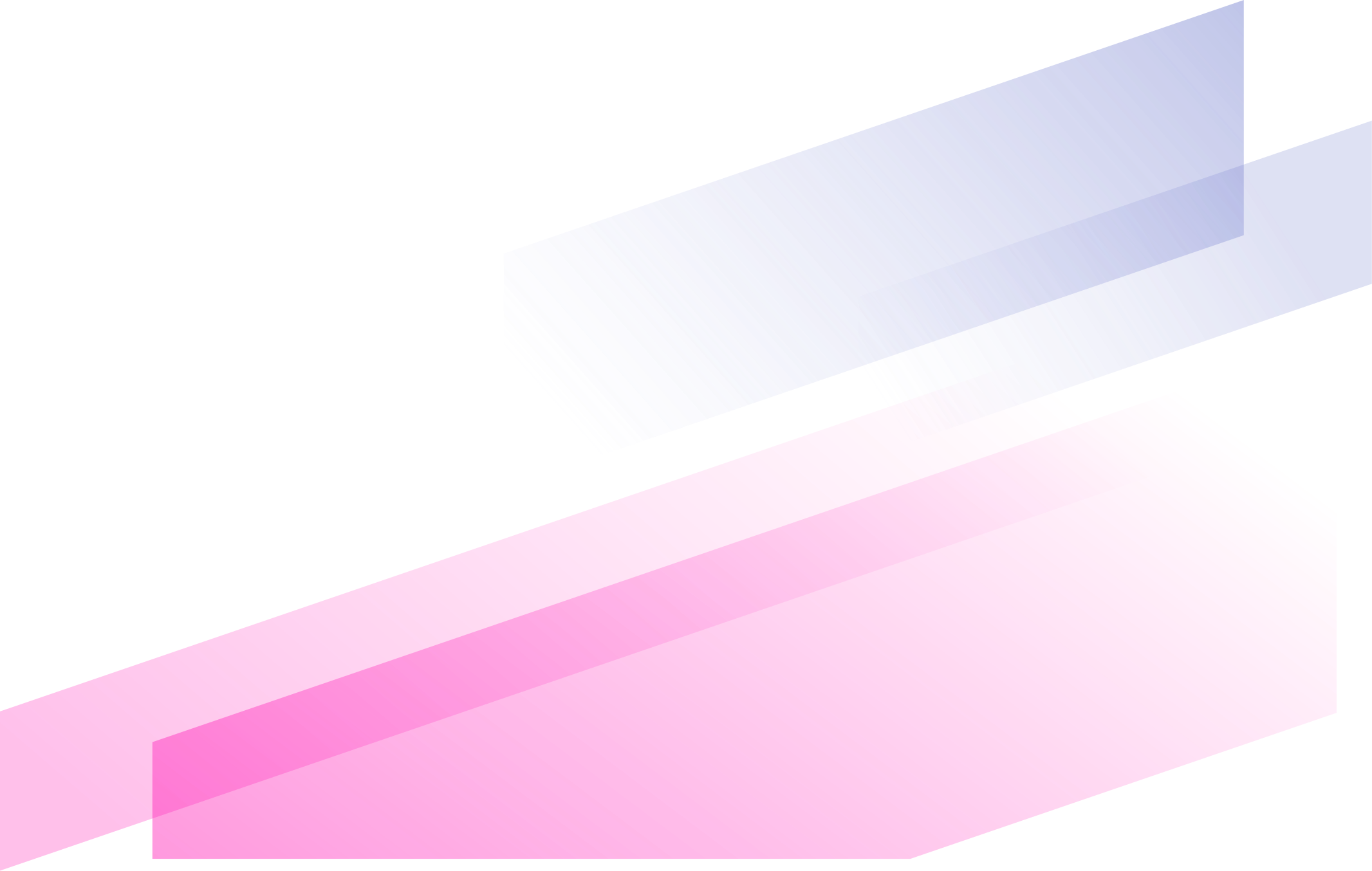
The applications of deep learning algorithms on in silico druggable proteins identification.
CYP2C8*3 and *4 define CYP2C8 phenotype: An approach with the substrate cinitapride.
Network Analysis for Signal Detection in Spontaneous Adverse Event Reporting Database: Application of Network Weighting Normalization to Characterize Cardiovascular Drug Safety.
A network paradigm predicts drug synergistic effects using downstream protein-protein interactions.
The PBPK LeiCNS-PK3.0 framework predicts Nirmatrelvir (but not Remdesivir or Molnupiravir) to achieve effective concentrations against SARS-CoV-2 in human brain cells.
Human health risk assessment of pharmaceuticals in the European Vecht River.
Interpretable deep learning translation of GWAS and multi-omics findings to identify pathobiology and drug repurposing in Alzheimer's disease.
Inhibition of IRAK1 Is an Effective Therapy for Autoimmune Hypophysitis in Mice.
The integration of large-scale public data and network analysis uncovers molecular characteristics of psoriasis.
ES-Screen: A Novel Electrostatics-Driven Method for Drug Discovery Virtual Screening.