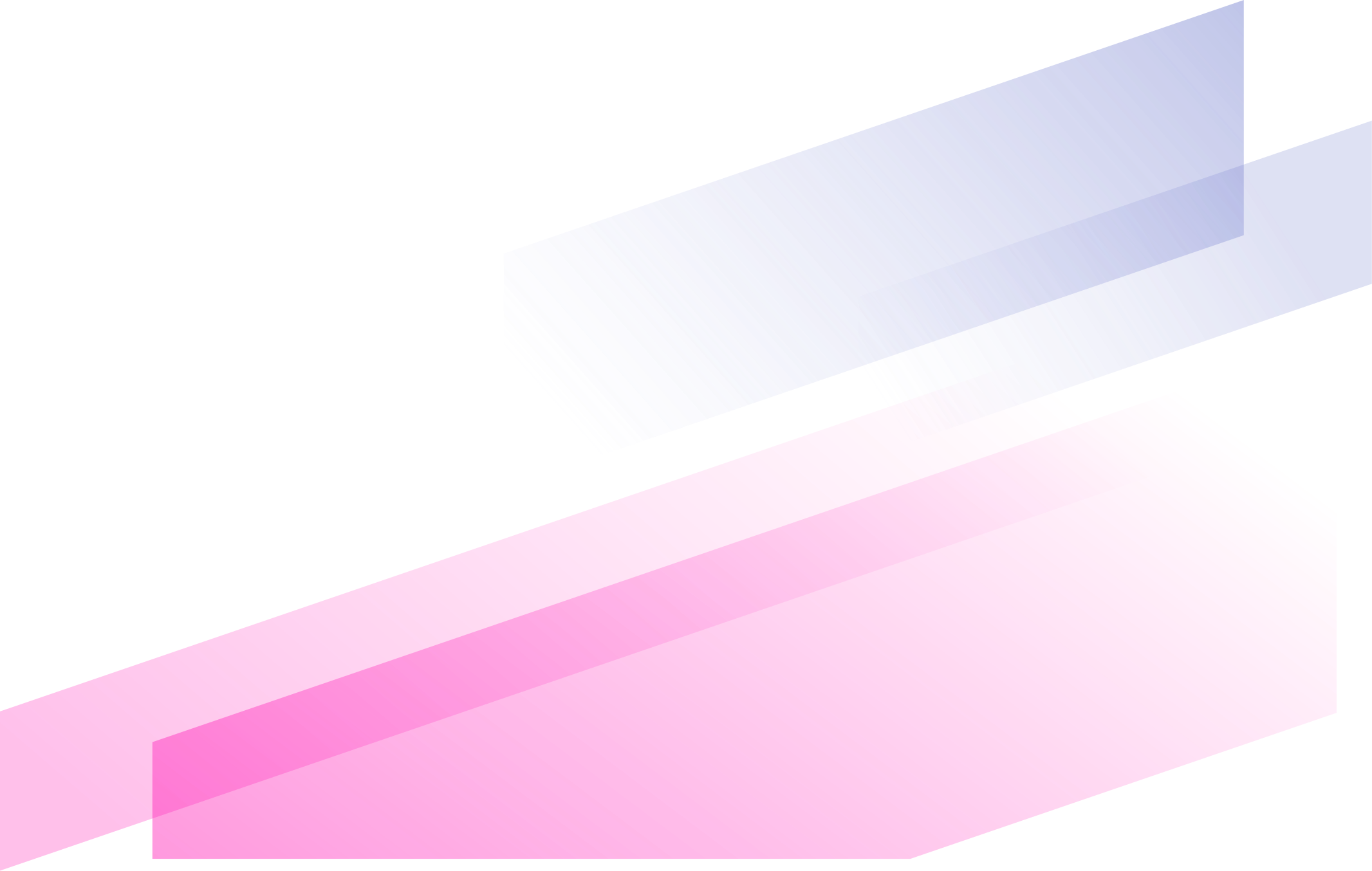
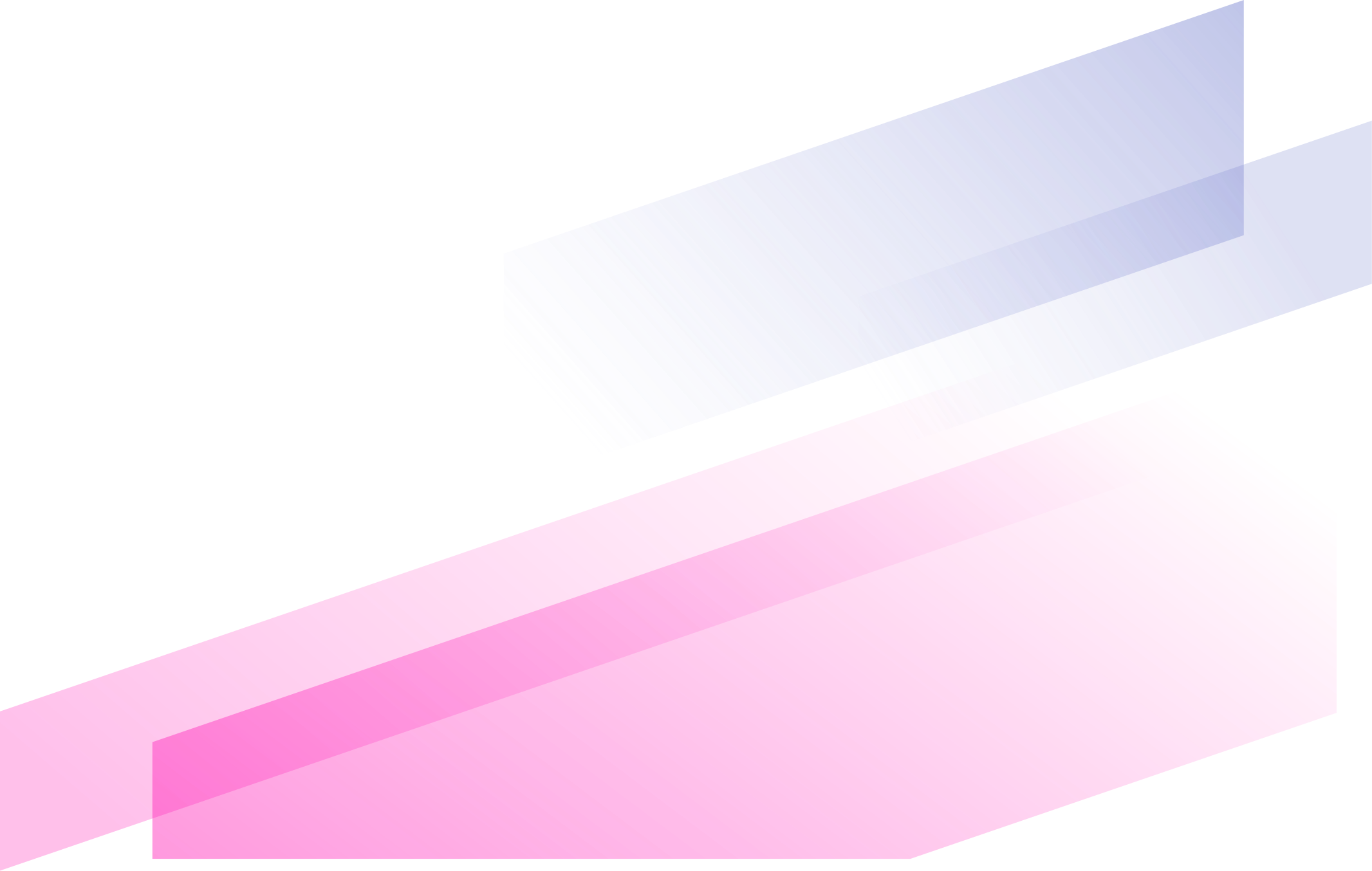
Initial characterization of the human central proteome.
Drug-drug relationship based on target information: application to drug target identification.
Translating clinical findings into knowledge in drug safety evaluation--drug induced liver injury prediction system (DILIps).
From pharmacogenomic knowledge acquisition to clinical applications: the PharmGKB as a clinical pharmacogenomic biomarker resource.
Can heparins stimulate bone cancer stem cells and interfere with tumorigenesis?
In silico approach to screen compounds active against parasitic nematodes of major socio-economic importance.
Drug repositioning using disease associated biological processes and network analysis of drug targets.
Dominating biological networks.
Drug-target network in myocardial infarction reveals multiple side effects of unrelated drugs.
Exploring schizophrenia drug-gene interactions through molecular network and pathway modeling.