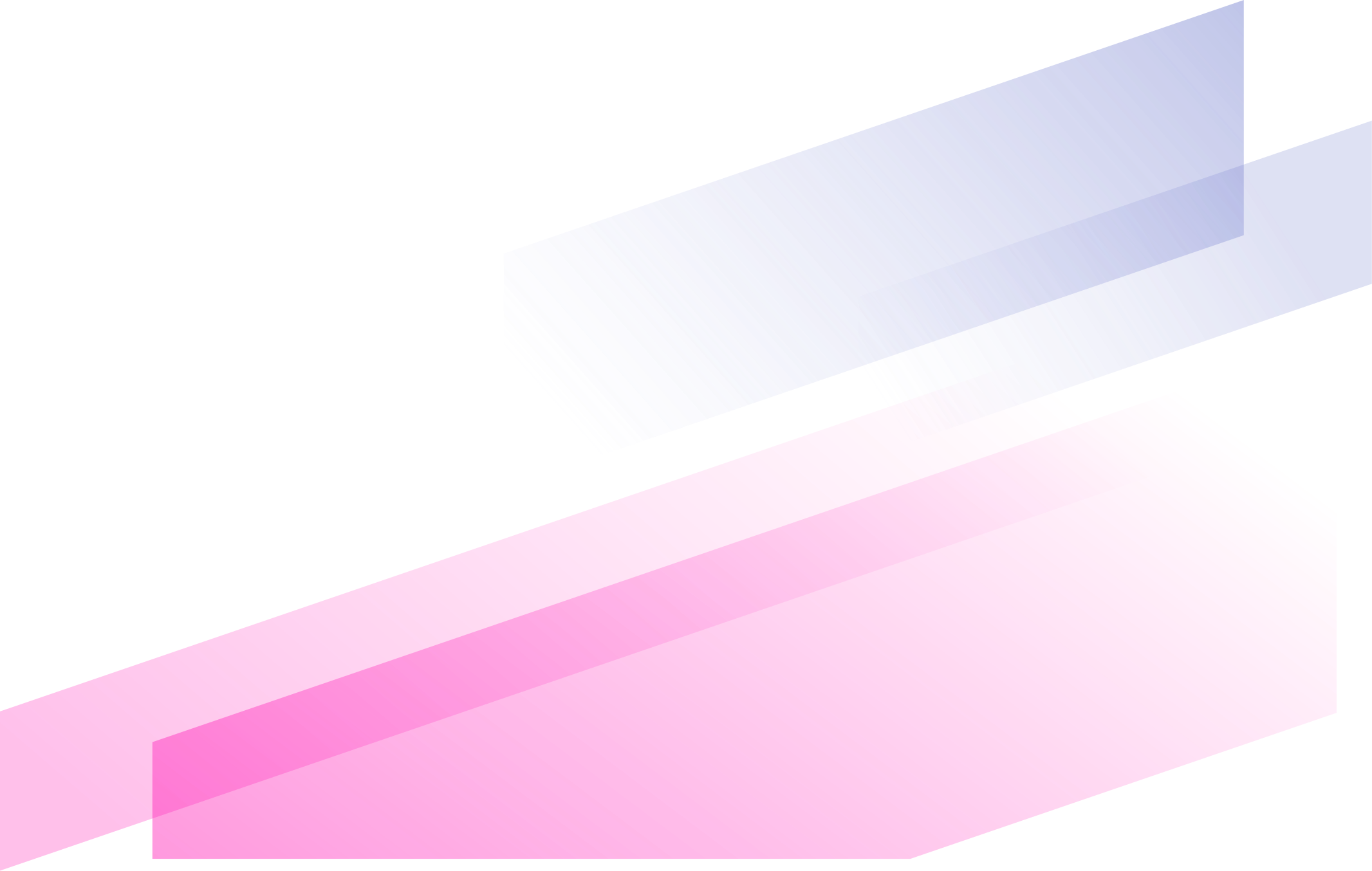
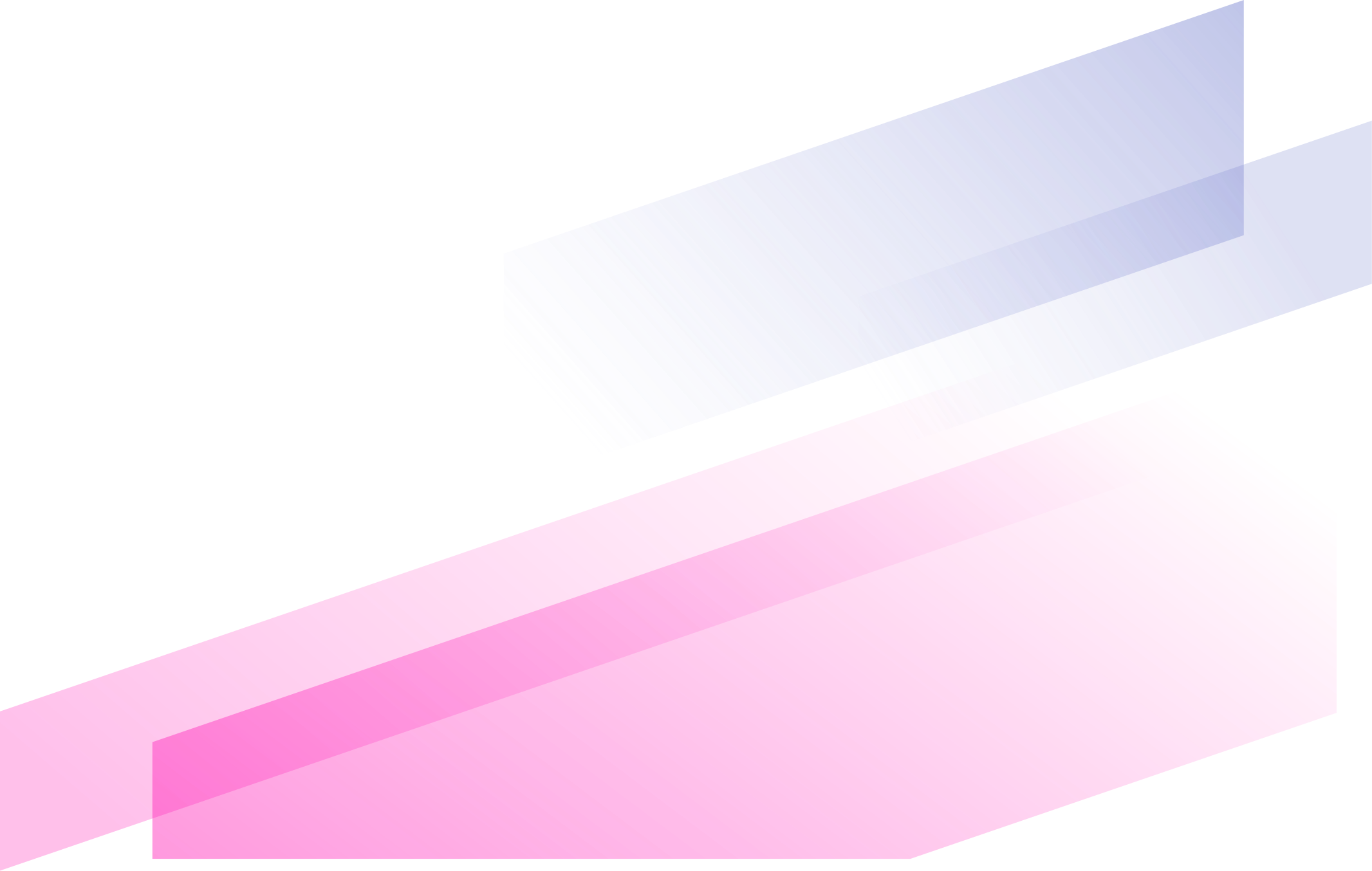
Publications Search
Explore how scientists all over the world use DrugBank in their research.
Showing 4901 to 4904 of 5186 entries
Published in January 2012
Systems biology of kidney diseases.
Abstract: Kidney diseases manifest in progressive loss of renal function, which ultimately leads to complete kidney failure. The mechanisms underlying the origins and progression of kidney diseases are not fully understood. Multiple factors involved in the pathogenesis of kidney diseases have made the traditional candidate gene approach of limited value toward full understanding of the molecular mechanisms of these diseases. A systems biology approach that integrates computational modeling with large-scale data gathering of the molecular changes could be useful in identifying the multiple interacting genes and their products that drive kidney diseases. Advances in biotechnology now make it possible to gather large data sets to characterize the role of the genome, epigenome, transcriptome, proteome, and metabolome in kidney diseases. When combined with computational analyses, these experimental approaches will provide a comprehensive understanding of the underlying biological processes. Multiscale analysis that connects the molecular interactions and cell biology of different kidney cells to renal physiology and pathology can be utilized to identify modules of biological and clinical importance that are perturbed in disease processes. This integration of experimental approaches and computational modeling is expected to generate new knowledge that can help to identify marker sets to guide the diagnosis, monitor disease progression, and identify new therapeutic targets.
Published on January 24, 2012
Quantifying the chemical beauty of drugs.
Abstract: Drug-likeness is a key consideration when selecting compounds during the early stages of drug discovery. However, evaluation of drug-likeness in absolute terms does not reflect adequately the whole spectrum of compound quality. More worryingly, widely used rules may inadvertently foster undesirable molecular property inflation as they permit the encroachment of rule-compliant compounds towards their boundaries. We propose a measure of drug-likeness based on the concept of desirability called the quantitative estimate of drug-likeness (QED). The empirical rationale of QED reflects the underlying distribution of molecular properties. QED is intuitive, transparent, straightforward to implement in many practical settings and allows compounds to be ranked by their relative merit. We extended the utility of QED by applying it to the problem of molecular target druggability assessment by prioritizing a large set of published bioactive compounds. The measure may also capture the abstract notion of aesthetics in medicinal chemistry.
Published on January 20, 2012
Predicting the in vivo mechanism of action for drug leads using NMR metabolomics.
Abstract: New strategies are needed to circumvent increasing outbreaks of resistant strains of pathogens and to expand the dwindling supply of effective antimicrobials. A common impediment to drug development is the lack of an easy approach to determine the in vivo mechanism of action and efficacy of novel drug leads. Toward this end, we describe an unbiased approach to predict in vivo mechanisms of action from NMR metabolomics data. Mycobacterium smegmatis, a non-pathogenic model organism for Mycobacterium tuberculosis, was treated with 12 known drugs and 3 chemical leads identified from a cell-based assay. NMR analysis of drug-induced changes to the M. smegmatis metabolome resulted in distinct clustering patterns correlating with in vivo drug activity. The clustering of novel chemical leads relative to known drugs provides a mean to identify a protein target or predict in vivo activity.
Published on January 19, 2012
Identifying co-targets to fight drug resistance based on a random walk model.
Abstract: BACKGROUND: Drug resistance has now posed more severe and emergent threats to human health and infectious disease treatment. However, wet-lab approaches alone to counter drug resistance have so far still achieved limited success due to less knowledge about the underlying mechanisms of drug resistance. Our approach apply a heuristic search algorithm in order to extract active network under drug treatment and use a random walk model to identify potential co-targets for effective antibacterial drugs. RESULTS: We use interactome network of Mycobacterium tuberculosis and gene expression data which are treated with two kinds of antibiotic, Isoniazid and Ethionamide as our test data. Our analysis shows that the active drug-treated networks are associated with the trigger of fatty acid metabolism and synthesis and nicotinamide adenine dinucleotide (NADH)-related processes and those results are consistent with the recent experimental findings. Efflux pumps processes appear to be the major mechanisms of resistance but SOS response is significantly up-regulation under Isoniazid treatment. We also successfully identify the potential co-targets with literature confirmed evidences which are related to the glycine-rich membrane, adenosine triphosphate energy and cell wall processes. CONCLUSIONS: With gene expression and interactome data supported, our study points out possible pathways leading to the emergence of drug resistance under drug treatment. We develop a computational workflow for giving new insights to bacterial drug resistance which can be gained by a systematic and global analysis of the bacterial regulation network. Our study also discovers the potential co-targets with good properties in biological and graph theory aspects to overcome the problem of drug resistance.
Published on January 18, 2012
Acetylcholinesterase inhibitors for schizophrenia.
Abstract: BACKGROUND: Antipsychotic medication remains the mainstay of treatment for schizophrenia and has been in use for a long time. As evidenced by ongoing research and partial effectiveness of the antipsychotics on cognitive and negative symptoms, the search is on for drugs that may improve these domains of functioning for someone suffering from schizophrenia. Acetylcholinesterase inhibitors have long been in use for treating cognitive symptoms of dementia. OBJECTIVES: The aim of the review was to evaluate the clinical effects, safety and cost effectiveness of acetylcholinesterase inhibitors for treating people with schizophrenia SEARCH METHODS: We searched the Cochrane Schizophrenia Group's Register (February 2009), and inspected the references of all identified studies for further trials. SELECTION CRITERIA: We included all clinical randomised trials comparing acetylcholinesterase inhibitors with antipsychotics or placebo either alone, or in combination, for schizophrenia and schizophrenia-like psychoses. DATA COLLECTION AND ANALYSIS: We extracted data independently. For dichotomous data, we calculated risk ratios (RR) and their 95% confidence intervals (CI) on an intention-to-treat (ITT) basis based on a random-effects model. For continuous data, we calculated mean differences (MD), again based on a random-effects model. MAIN RESULTS: The acetylcholinesterase inhibitor plus antipsychotic showed benefit over antipsychotic and placebo in the following outcomes.1. Mental state - PANSS negative symptoms average end point score (2 RCTs, n = 31, MD -1.69 95% CI -2.80 to -0.57), PANSS General Psychopathology average end point score (2 RCTs, n = 31, MD -3.86 95% CI -5.40 to -2.32), and improvement in depressive symptoms showed at least by one short-term study as measured by CDSS scale (data skewed).2. Cognitive domains - attention, (1 RCT, n = 73, MD 1.20 95% CI 0.14 to 2.26), visual memory (2 RCTs, n = 48 , MD 1.90 95% CI 0.52 to 3.28), verbal memory and language (3 RCTs, n = 42, MD 3.46 95% CI 0.67 to 6.26) and executive functioning (1 RCT, n = 24, MD 17.10 95% CI 0.70 to 33.50).3. Tolerability - EPSE: AIMS, (1 RCT, n = 35, MD 1.50 95% CI 1.04 to 1.96).No difference was noted between the two arms in other outcomes. The overall rate of participants leaving studies early was low (13.6 %) and showed no clear difference between the two groups. AUTHORS' CONCLUSIONS: The results seem to favour the use of acetylcholinesterase inhibitors in combination with antipsychotics on a few domains of mental state and cognition, but because of the various limitations in the studies as mentioned in the main text, the evidence is weak. This review highlights the need for large, independent, well designed, conducted and reported pragmatic randomised studies.
Published on January 15, 2012
Whole-genome sequence of Schistosoma haematobium.
Abstract: Schistosomiasis is a neglected tropical disease caused by blood flukes (genus Schistosoma; schistosomes) and affecting 200 million people worldwide. No vaccines are available, and treatment relies on one drug, praziquantel. Schistosoma haematobium has come into the spotlight as a major cause of urogenital disease, as an agent linked to bladder cancer and as a predisposing factor for HIV/AIDS. The parasite is transmitted to humans from freshwater snails. Worms dwell in blood vessels and release eggs that become embedded in the bladder wall to elicit chronic immune-mediated disease and induce squamous cell carcinoma. Here we sequenced the 385-Mb genome of S. haematobium using Illumina-based technology at 74-fold coverage and compared it to sequences from related parasites. We included genome annotation based on function, gene ontology, networking and pathway mapping. This genome now provides an unprecedented resource for many fundamental research areas and shows great promise for the design of new disease interventions.
Published on January 13, 2012
Modulating protein-protein interactions with small molecules: the importance of binding hotspots.
Abstract: The modulation of protein-protein interactions (PPIs) by small drug-like molecules is a relatively new area of research and has opened up new opportunities in drug discovery. However, the progress made in this area is limited to a handful of known cases of small molecules that target specific diseases. With the increasing availability of protein structure complexes, it is highly important to devise strategies exploiting homologous structure space on a large scale for discovering putative PPIs that could be attractive drug targets. Here, we propose a scheme that allows performing large-scale screening of all protein complexes and finding putative small-molecule and/or peptide binding sites overlapping with protein-protein binding sites (so-called "multibinding sites"). We find more than 600 nonredundant proteins from 60 protein families with multibinding sites. Moreover, we show that the multibinding sites are mostly observed in transient complexes, largely overlap with the binding hotspots and are more evolutionarily conserved than other interface sites. We investigate possible mechanisms of how small molecules may modulate protein-protein binding and discuss examples of new candidates for drug design.
Published on January 13, 2012
A network flow approach to predict drug targets from microarray data, disease genes and interactome network - case study on prostate cancer.
Abstract: BACKGROUND: Systematic approach for drug discovery is an emerging discipline in systems biology research area. It aims at integrating interaction data and experimental data to elucidate diseases and also raises new issues in drug discovery for cancer treatment. However, drug target discovery is still at a trial-and-error experimental stage and it is a challenging task to develop a prediction model that can systematically detect possible drug targets to deal with complex diseases. METHODS: We integrate gene expression, disease genes and interaction networks to identify the effective drug targets which have a strong influence on disease genes using network flow approach. In the experiments, we adopt the microarray dataset containing 62 prostate cancer samples and 41 normal samples, 108 known prostate cancer genes and 322 approved drug targets treated in human extracted from DrugBank database to be candidate proteins as our test data. Using our method, we prioritize the candidate proteins and validate them to the known prostate cancer drug targets. RESULTS: We successfully identify potential drug targets which are strongly related to the well known drugs for prostate cancer treatment and also discover more potential drug targets which raise the attention to biologists at present. We denote that it is hard to discover drug targets based only on differential expression changes due to the fact that those genes used to be drug targets may not always have significant expression changes. Comparing to previous methods that depend on the network topology attributes, they turn out that the genes having potential as drug targets are weakly correlated to critical points in a network. In comparison with previous methods, our results have highest mean average precision and also rank the position of the truly drug targets higher. It thereby verifies the effectiveness of our method. CONCLUSIONS: Our method does not know the real ideal routes in the disease network but it tries to find the feasible flow to give a strong influence to the disease genes through possible paths. We successfully formulate the identification of drug target prediction as a maximum flow problem on biological networks and discover potential drug targets in an accurate manner.
Published on January 10, 2012
Serendipity in anticancer drug discovery.
Abstract: It was found that the discovery of 5.8% (84/1437) of all drugs on the market involved serendipity. Of these drugs, 31 (2.2%) were discovered following an incident in the laboratory and 53 (3.7%) were discovered in a clinical setting. In addition, 263 (18.3%) of the pharmaceuticals in clinical use today are chemical derivatives of the drugs discovered with the aid of serendipity. Therefore, in total, 24.1% (347/1437) of marketed drugs can be directly traced to serendipitous events confirming the importance of this elusive phenomenon. In the case of anticancer drugs, 35.2% (31/88) can be attributed to a serendipitous event, which is somewhat larger than for all drugs. The therapeutic field that has benefited the most from serendipity are central nervous system active drugs reflecting the difficulty in designing compounds to pass the blood-brain-barrier and the lack of laboratory-based assays for many of the diseases of the mind.
Published on January 6, 2012
Guidelines for information about therapy experiments: a proposal on best practice for recording experimental data on cancer therapy.
Abstract: BACKGROUND: Biology, biomedicine and healthcare have become data-driven enterprises, where scientists and clinicians need to generate, access, validate, interpret and integrate different kinds of experimental and patient-related data. Thus, recording and reporting of data in a systematic and unambiguous fashion is crucial to allow aggregation and re-use of data. This paper reviews the benefits of existing biomedical data standards and focuses on key elements to record experiments for therapy development. Specifically, we describe the experiments performed in molecular, cellular, animal and clinical models. We also provide an example set of elements for a therapy tested in a phase I clinical trial. FINDINGS: We introduce the Guidelines for Information About Therapy Experiments (GIATE), a minimum information checklist creating a consistent framework to transparently report the purpose, methods and results of the therapeutic experiments. A discussion on the scope, design and structure of the guidelines is presented, together with a description of the intended audience. We also present complementary resources such as a classification scheme, and two alternative ways of creating GIATE information: an electronic lab notebook and a simple spreadsheet-based format. Finally, we use GIATE to record the details of the phase I clinical trial of CHT-25 for patients with refractory lymphomas. The benefits of using GIATE for this experiment are discussed. CONCLUSIONS: While data standards are being developed to facilitate data sharing and integration in various aspects of experimental medicine, such as genomics and clinical data, no previous work focused on therapy development. We propose a checklist for therapy experiments and demonstrate its use in the 131Iodine labeled CHT-25 chimeric antibody cancer therapy. As future work, we will expand the set of GIATE tools to continue to encourage its use by cancer researchers, and we will engineer an ontology to annotate GIATE elements and facilitate unambiguous interpretation and data integration.
Showing 4901 to 4904 of 5186 entries