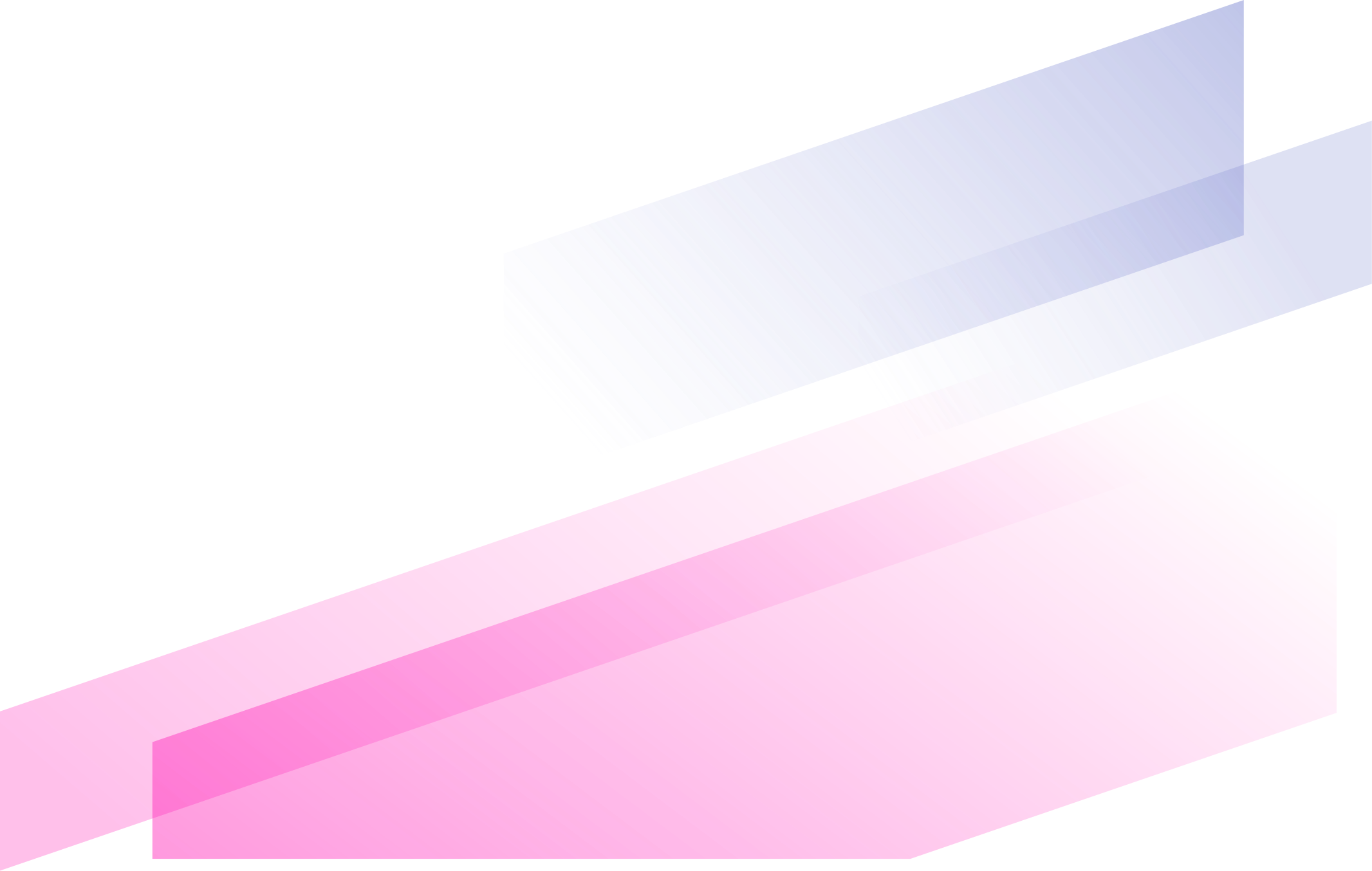
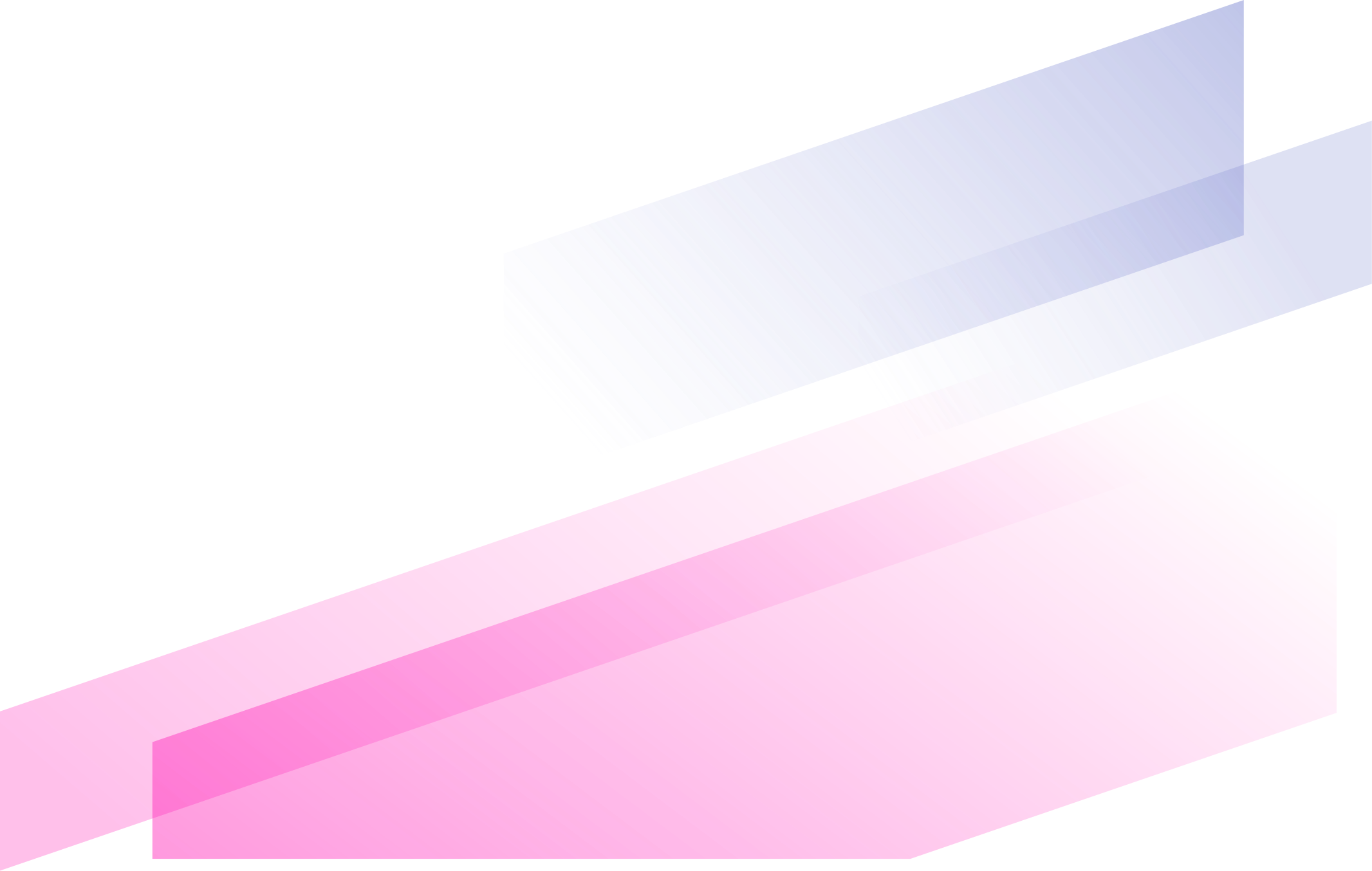
Exploiting the Stemness and Chemoresistance Transcriptome of Ewing Sarcoma to Identify Candidate Therapeutic Targets and Drug-Repurposing Candidates.
DeepMPF: deep learning framework for predicting drug-target interactions based on multi-modal representation with meta-path semantic analysis.
Fragment screening libraries for the identification of protein hot spots and their minimal binding pharmacophores.
Network pharmacology and experimental validation to identify the potential mechanism of Hedyotis diffusa Willd against rheumatoid arthritis.
Scaffold and Structural Diversity of the Secondary Metabolite Space of Medicinal Fungi.
Tenebrio molitor as a Simple and Cheap Preclinical Pharmacokinetic and Toxicity Model.
Guiding Drug Repositioning for Cancers Based on Drug Similarity Networks.
Permeability Assessment of a High-Throughput Mucosal Platform.
Future Antimicrobials: Natural and Functionalized Phenolics.
Synthesize heterogeneous biological knowledge via representation learning for Alzheimer's disease drug repurposing.