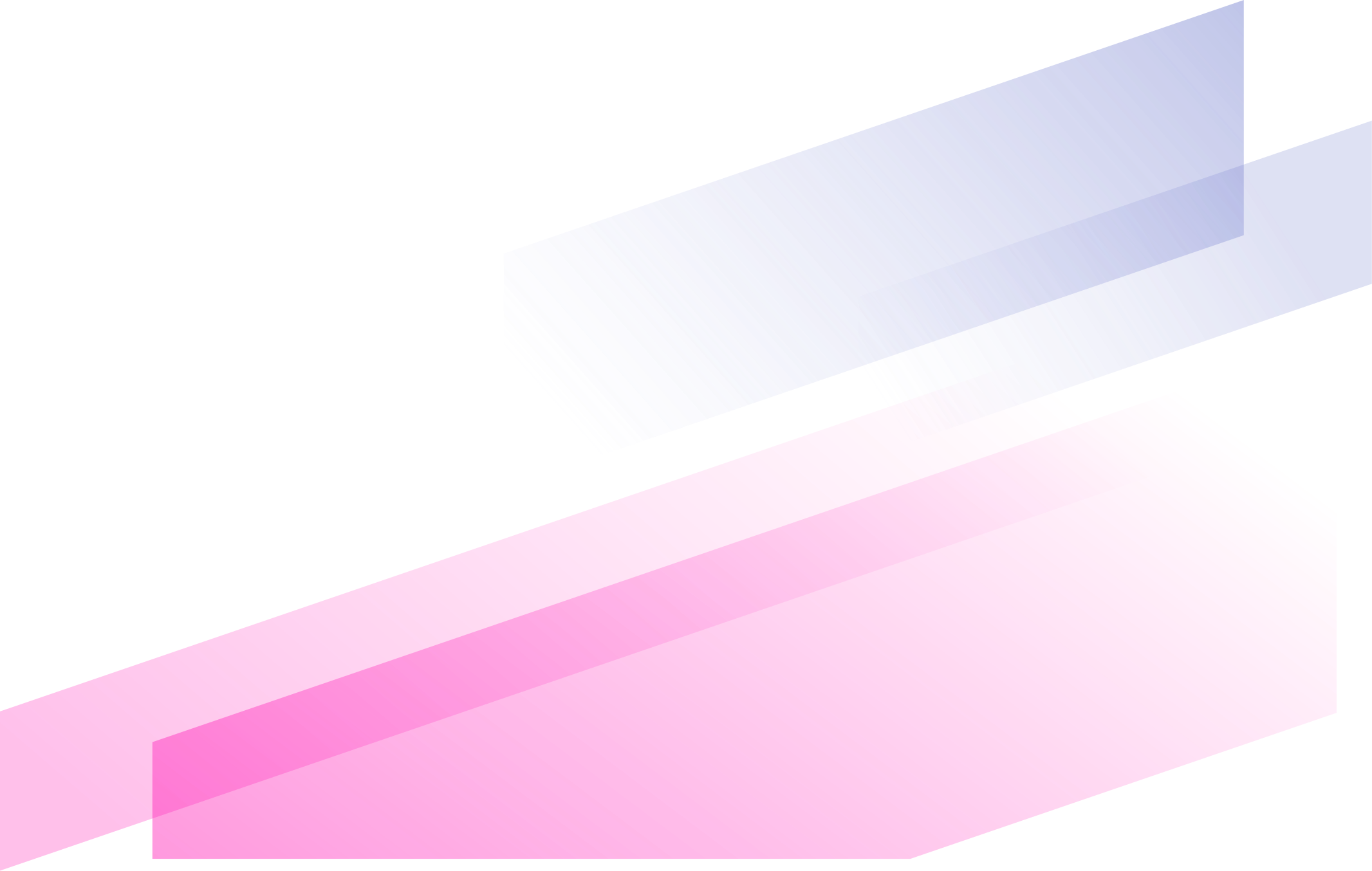
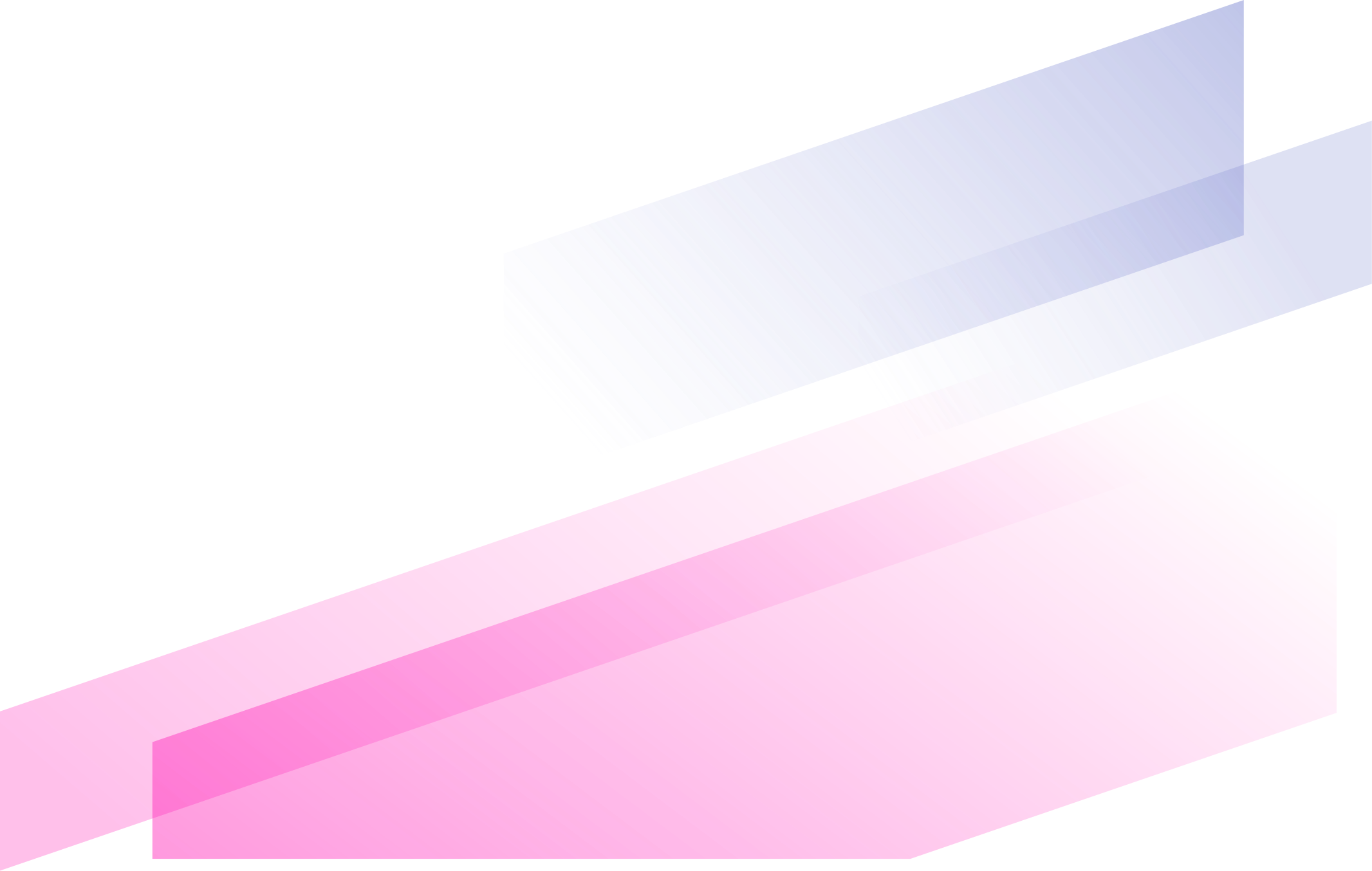
Exploration of databases and methods supporting drug repurposing: a comprehensive survey.
Fenamates as Potential Therapeutics for Neurodegenerative Disorders.
Virtual screening web servers: designing chemical probes and drug candidates in the cyberspace.
Coupled matrix-matrix and coupled tensor-matrix completion methods for predicting drug-target interactions.
Bioinformatics and system biology approach to identify the influences of COVID-19 on cardiovascular and hypertensive comorbidities.
Biological network analysis with deep learning.
Drug repurposing against breast cancer by integrating drug-exposure expression profiles and drug-drug links based on graph neural network.
Identification of disease treatment mechanisms through the multiscale interactome.
Proteomic blood profiling in mild, severe and critical COVID-19 patients.
Prioritizing antiviral drugs against SARS-CoV-2 by integrating viral complete genome sequences and drug chemical structures.