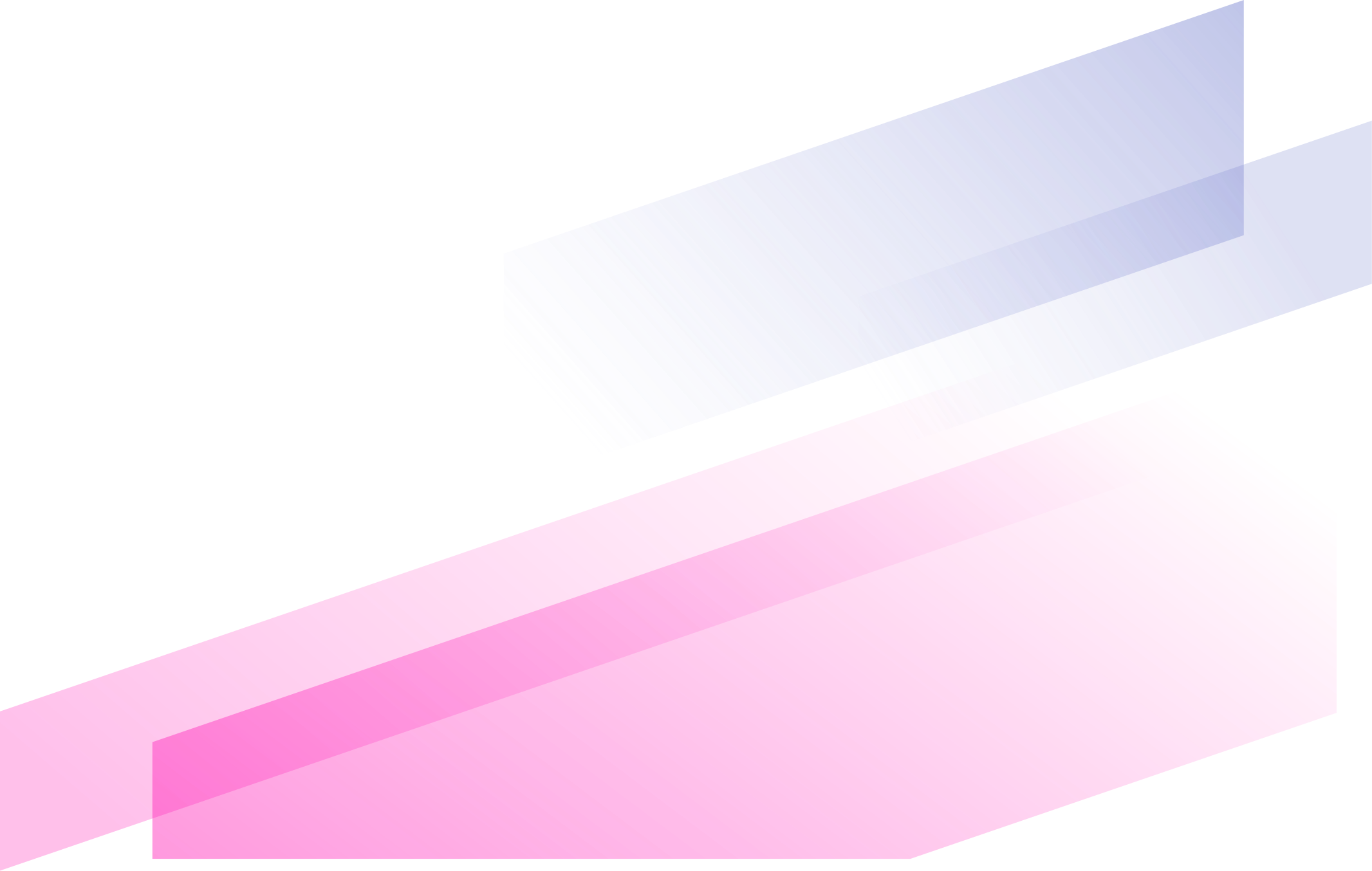
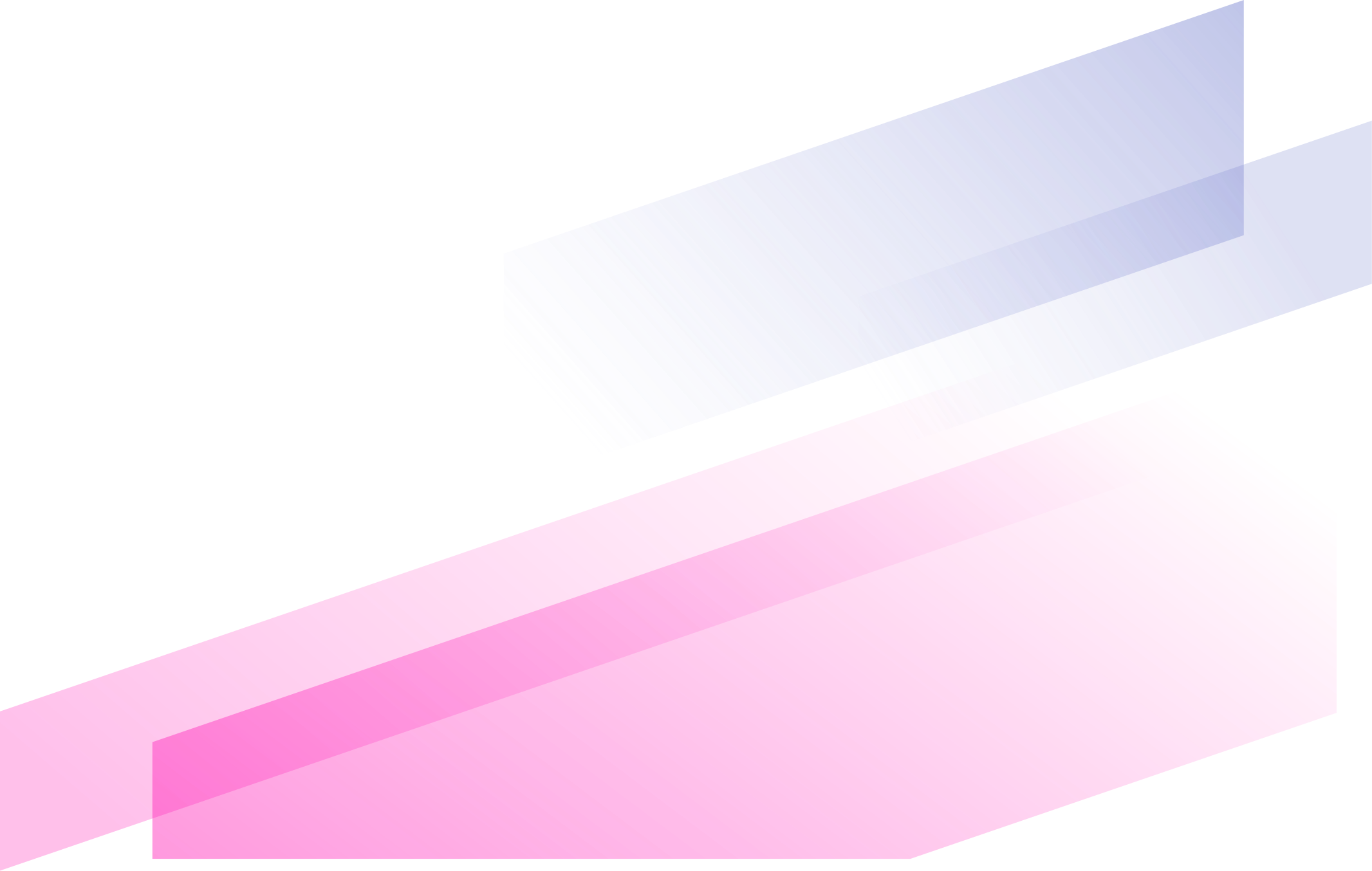
Lung adenocarcinoma-related target gene prediction and drug repositioning.
Investigation of the protective mechanism of leonurine against acute myocardial ischemia by an integrated metabolomics and network pharmacology strategy.
Evolution of Artificial Intelligence-Powered Technologies in Biomedical Research and Healthcare.
Network pharmacology and experimental analysis to reveal the mechanism of Dan-Shen-Yin against endothelial to mesenchymal transition in atherosclerosis.
Mechanism of Peitu Shengjin Formula Shenlingbaizhu Powder in Treating Bronchial Asthma and Allergic Colitis through Different Diseases with Simultaneous Treatment Based on Network Pharmacology and Molecular Docking.
Homology Modeling, de Novo Design of Ligands, and Molecular Docking Identify Potential Inhibitors of Leishmania donovani 24-Sterol Methyltransferase.
INPUT: An intelligent network pharmacology platform unique for traditional Chinese medicine.
Prediction of Synergistic Antibiotic Combinations by Graph Learning.
Combining biomedical knowledge graphs and text to improve predictions for drug-target interactions and drug-indications.
Dan-Shen-Yin Granules Prevent Hypoxia-Induced Pulmonary Hypertension via STAT3/HIF-1alpha/VEGF and FAK/AKT Signaling Pathways.