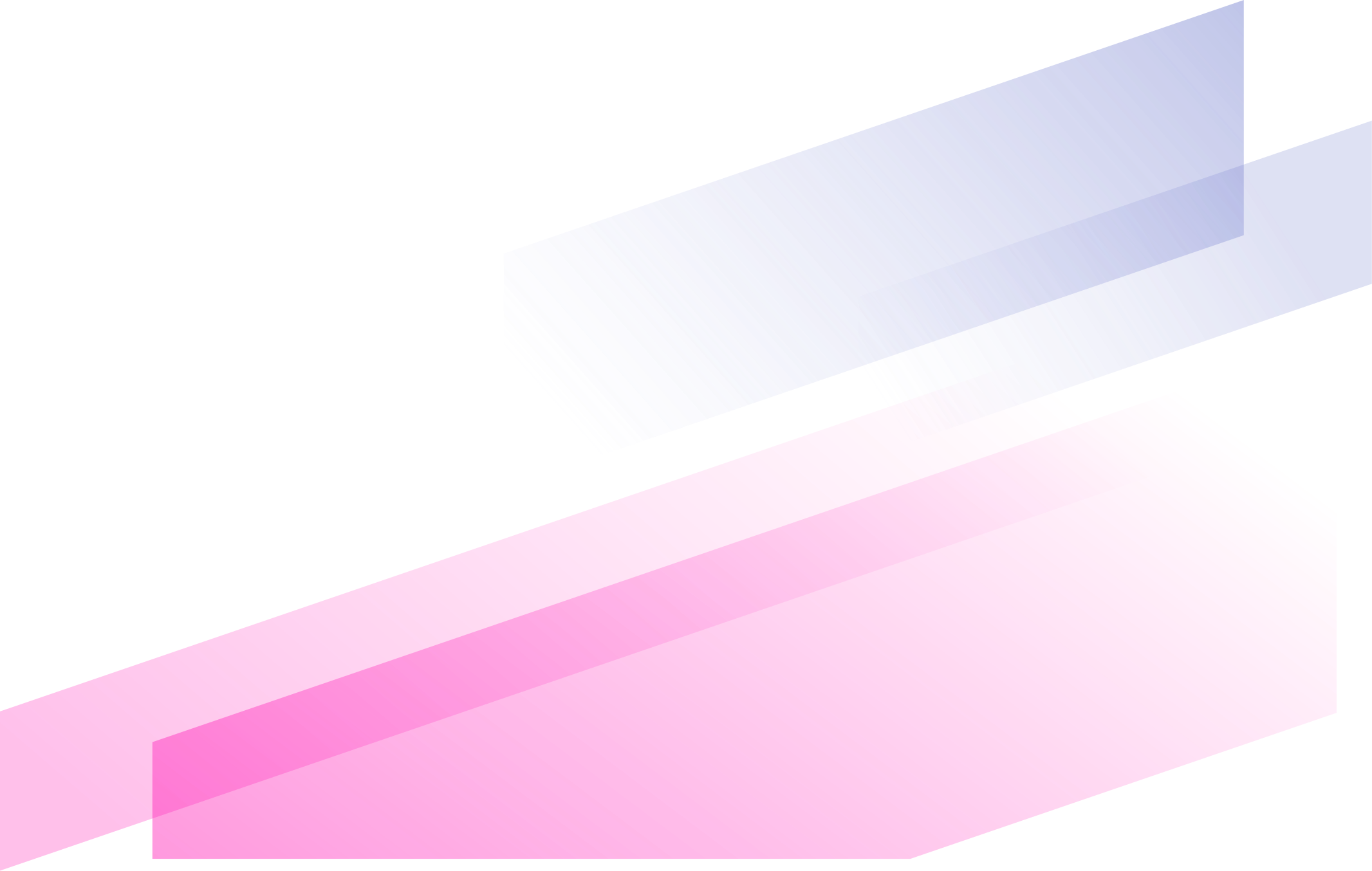
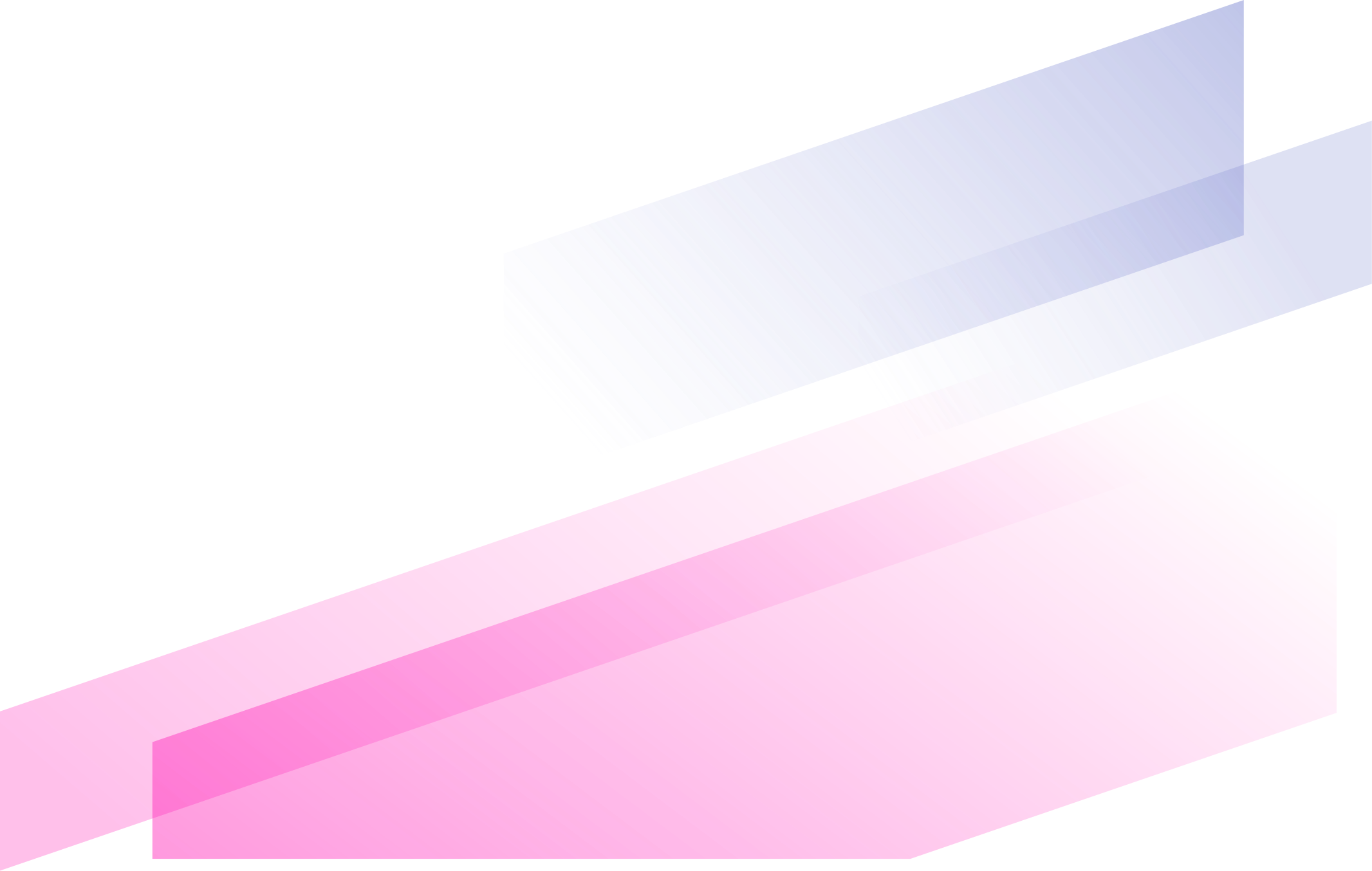
Ifenprodil and Flavopiridol Identified by Genomewide RNA Interference Screening as Effective Drugs To Ameliorate Murine Acute Lung Injury after Influenza A H5N1 Virus Infection.
Identification of STAB1 in Multiple Datasets as a Prognostic Factor for Cytogenetically Normal AML: Mechanism and Drug Indications.
Modulating FOXO3 transcriptional activity by small, DBD-binding molecules.
Systematically Prioritizing Candidates in Genome-Based Drug Repurposing.
RedMed: Extending drug lexicons for social media applications.
Evaluation of the target genes of arsenic trioxide in pancreatic cancer by bioinformatics analysis.
CMTTdb: the cancer molecular targeted therapy database.
Multi-residue ultra-performance liquid chromatography coupled with tandem mass spectrometry method for comprehensive multi-class anthropogenic compounds of emerging concern analysis in a catchment-based exposure-driven study.
Binding site matching in rational drug design: algorithms and applications.
BIOPEP-UWM Database of Bioactive Peptides: Current Opportunities.