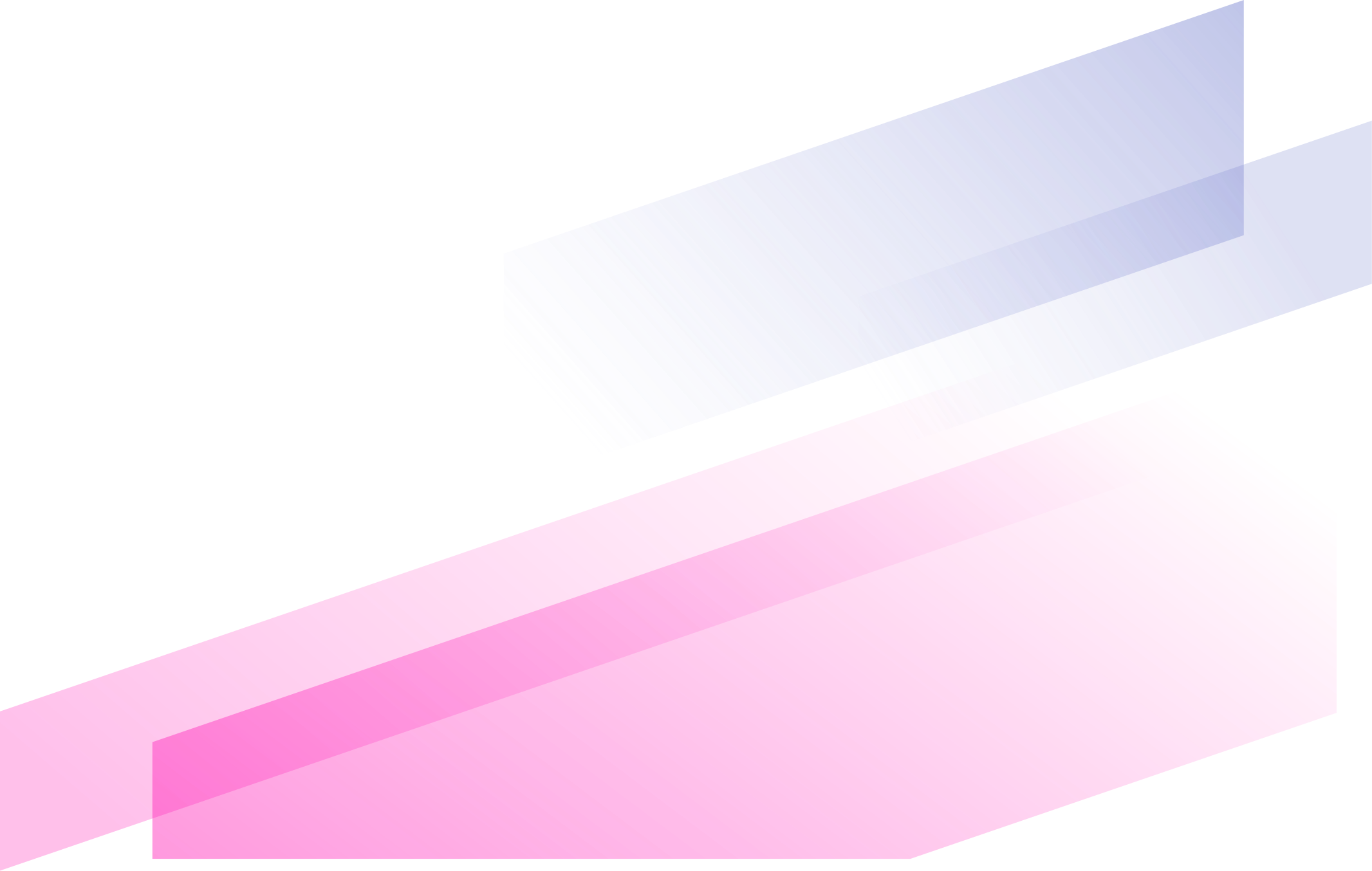
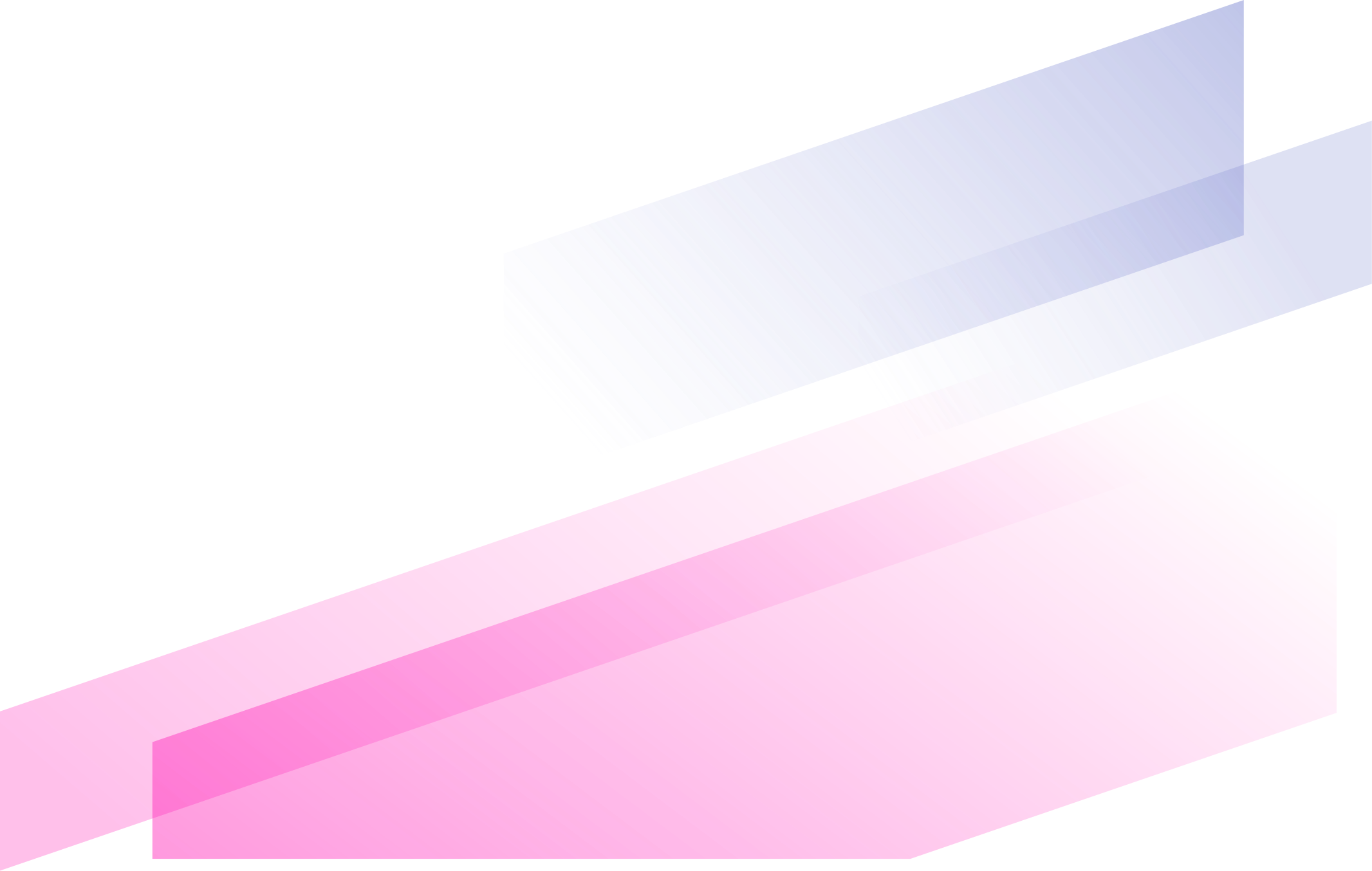
In silico Drug Repurposing to combat COVID-19 based on Pharmacogenomics of Patient Transcriptomic Data.
Potential RNA-dependent RNA polymerase inhibitors as prospective therapeutics against SARS-CoV-2.
A Physiologically-Based Quantitative Systems Pharmacology Model of the Incretin Hormones GLP-1 and GIP and the DPP4 Inhibitor Sitagliptin.
Towards the routine use of in silico screenings for drug discovery using metabolic modelling.
Discovery of Synergistic and Antagonistic Drug Combinations against SARS-CoV-2 In Vitro.
Quantifying genetic effects on disease mediated by assayed gene expression levels.
Immediate Adaptation Analysis Implicates BCL6 as an EGFR-TKI Combination Therapy Target in NSCLC.
A systems approach to infectious disease.
DTiGEMS+: drug-target interaction prediction using graph embedding, graph mining, and similarity-based techniques.
Hepatoprotective effects of Hovenia dulcis seeds against alcoholic liver injury and related mechanisms investigated via network pharmacology.