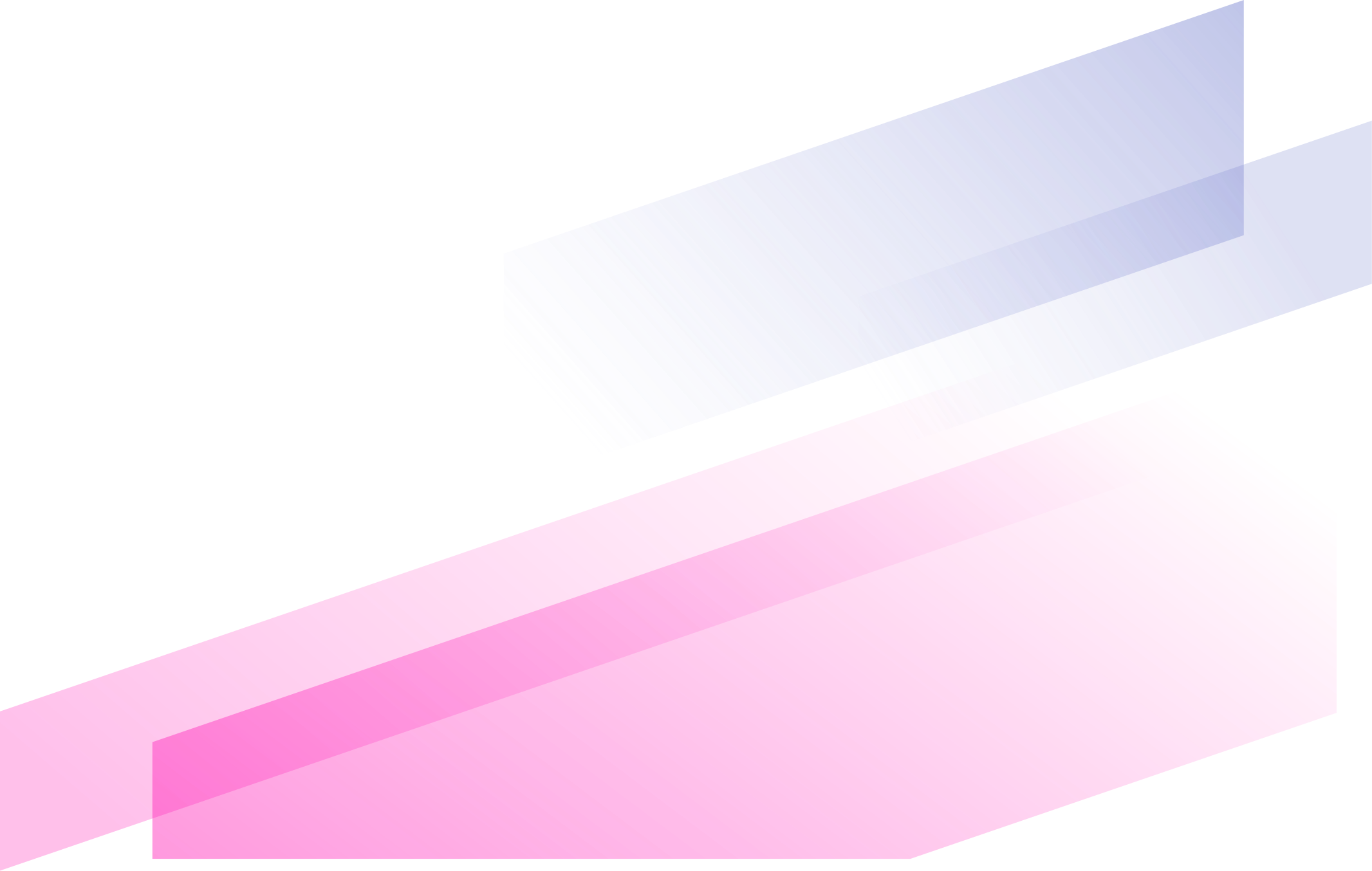
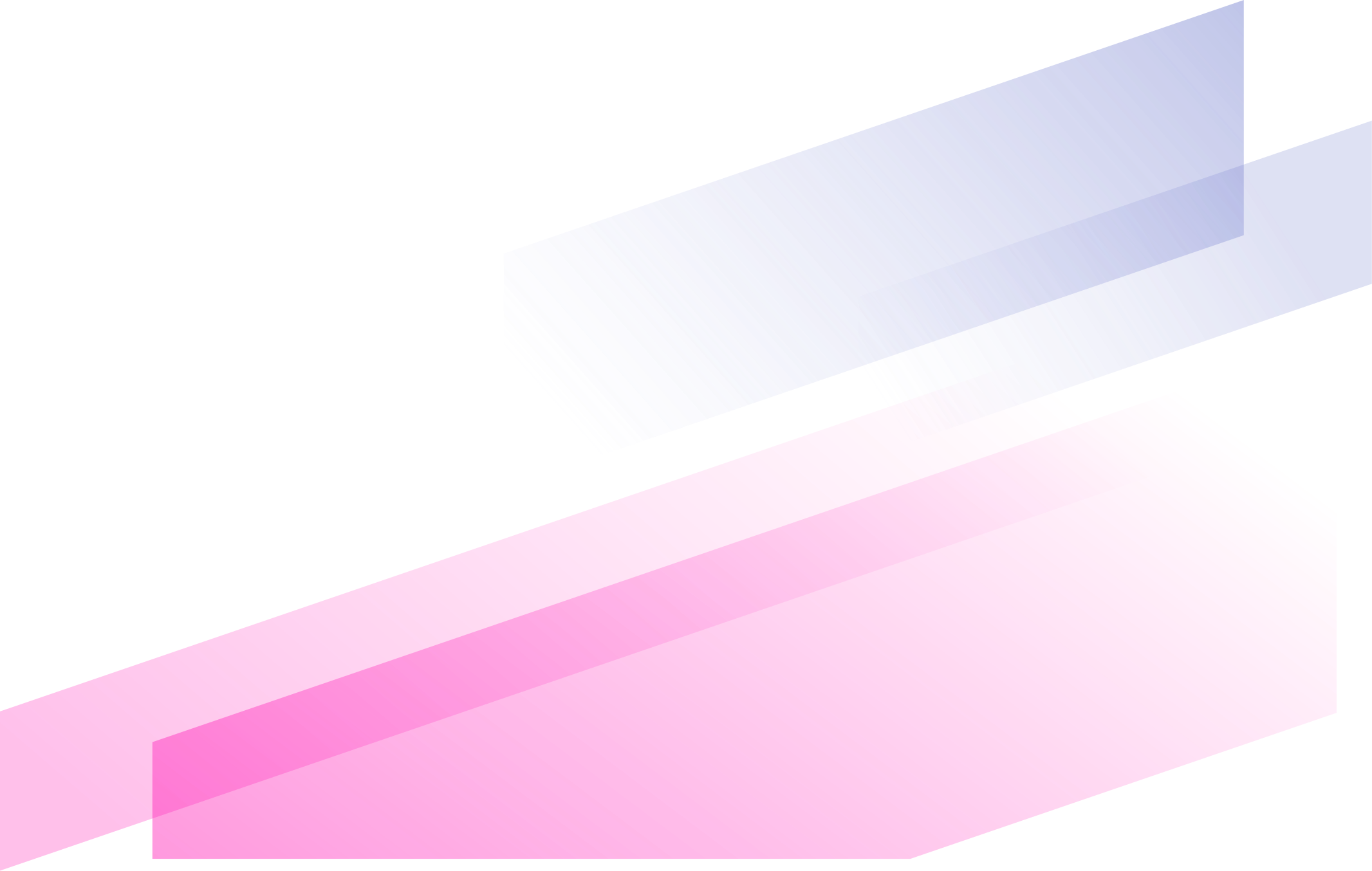
The COVID-19 Drug and Gene Set Library.
Interplay between Cellular Metabolism and the DNA Damage Response in Cancer.
Learning Representations to Predict Intermolecular Interactions on Large-Scale Heterogeneous Molecular Association Network.
Repurpose Open Data to Discover Therapeutics for COVID-19 Using Deep Learning.
Computational drug repurposing for the identification of SARS-CoV-2 main protease inhibitors.
Drug-target interactions prediction using marginalized denoising model on heterogeneous networks.
A review of computational drug repositioning: strategies, approaches, opportunities, challenges, and directions.
Filovirus Antiviral Activity of Cationic Amphiphilic Drugs Is Associated with Lipophilicity and Ability To Induce Phospholipidosis.
From Riluzole to Dexpramipexole via Substituted-Benzothiazole Derivatives for Amyotrophic Lateral Sclerosis Disease Treatment: Case Studies.
MIPDH: A Novel Computational Model for Predicting microRNA-mRNA Interactions by DeepWalk on a Heterogeneous Network.