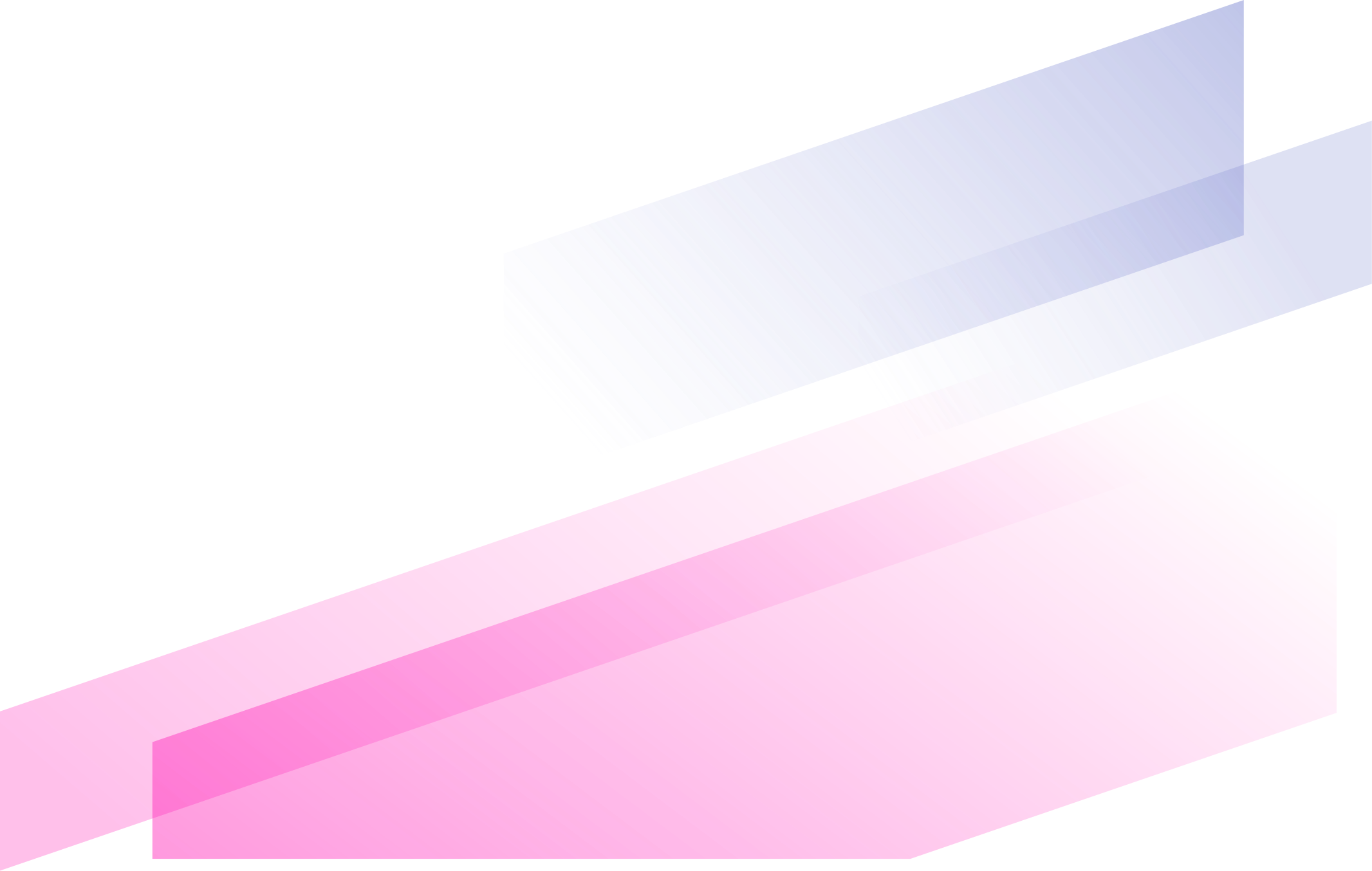
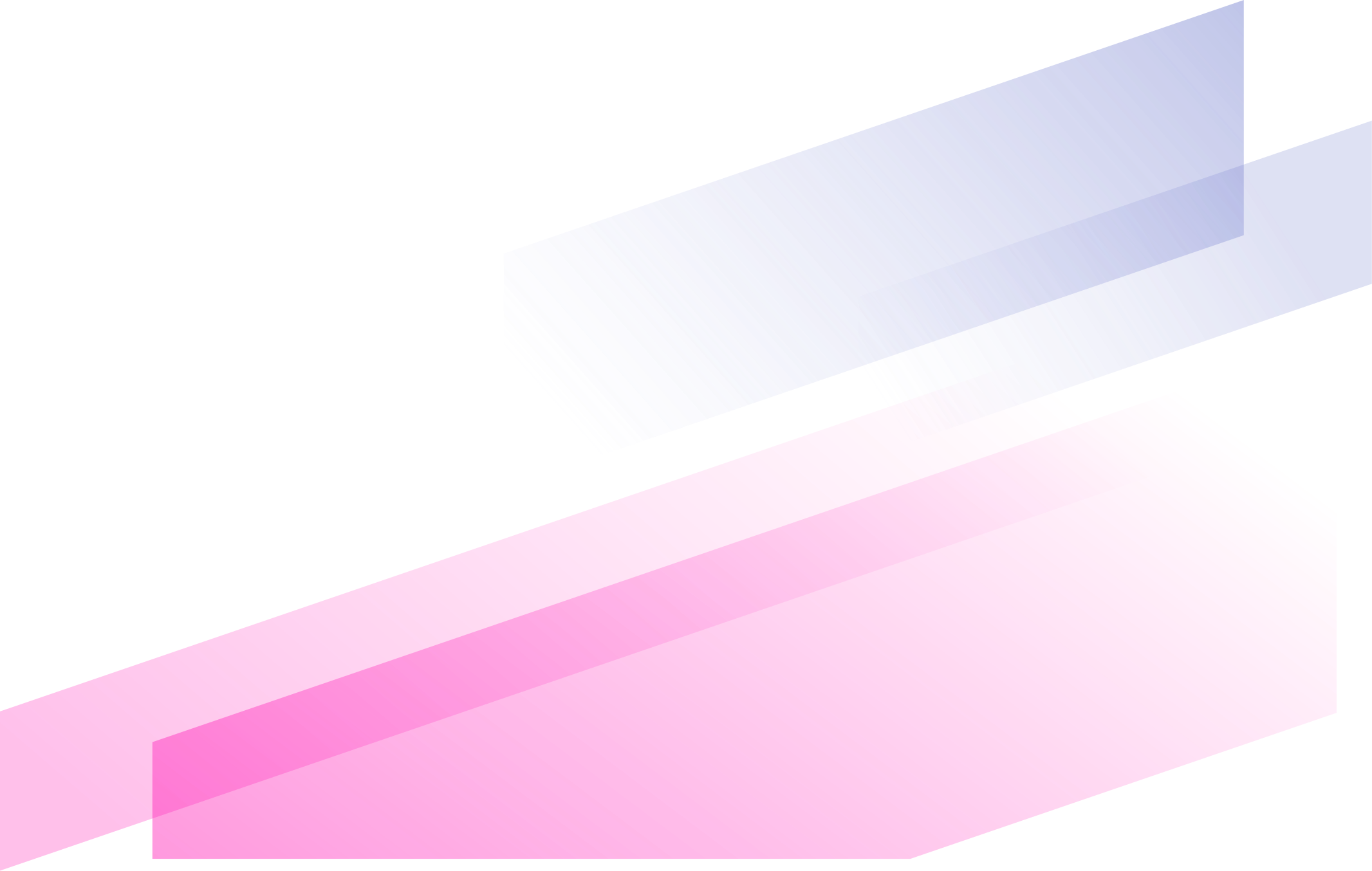
In-silico drug repurposing and molecular dynamics puzzled out potential SARS-CoV-2 main protease inhibitors.
Mechanisms of Spica Prunellae against thyroid-associated Ophthalmopathy based on network pharmacology and molecular docking.
A deep learning framework for high-throughput mechanism-driven phenotype compound screening.
ACE2 Co-evolutionary Pattern Suggests Targets for Pharmaceutical Intervention in the COVID-19 Pandemic.
Exploring Drug Treatment Patterns Based on the Action of Drug and Multilayer Network Model.
Synthetic repurposing of drugs against hypertension: a datamining method based on association rules and a novel discrete algorithm.
Lessons from Exploring Chemical Space and Chemical Diversity of Propolis Components.
New insights on human essential genes based on integrated analysis and the construction of the HEGIAP web-based platform.
Integrative pharmacological mechanism of vitamin C combined with glycyrrhizic acid against COVID-19: findings of bioinformatics analyses.
Evolutionary chemical binding similarity approach integrated with 3D-QSAR method for effective virtual screening.