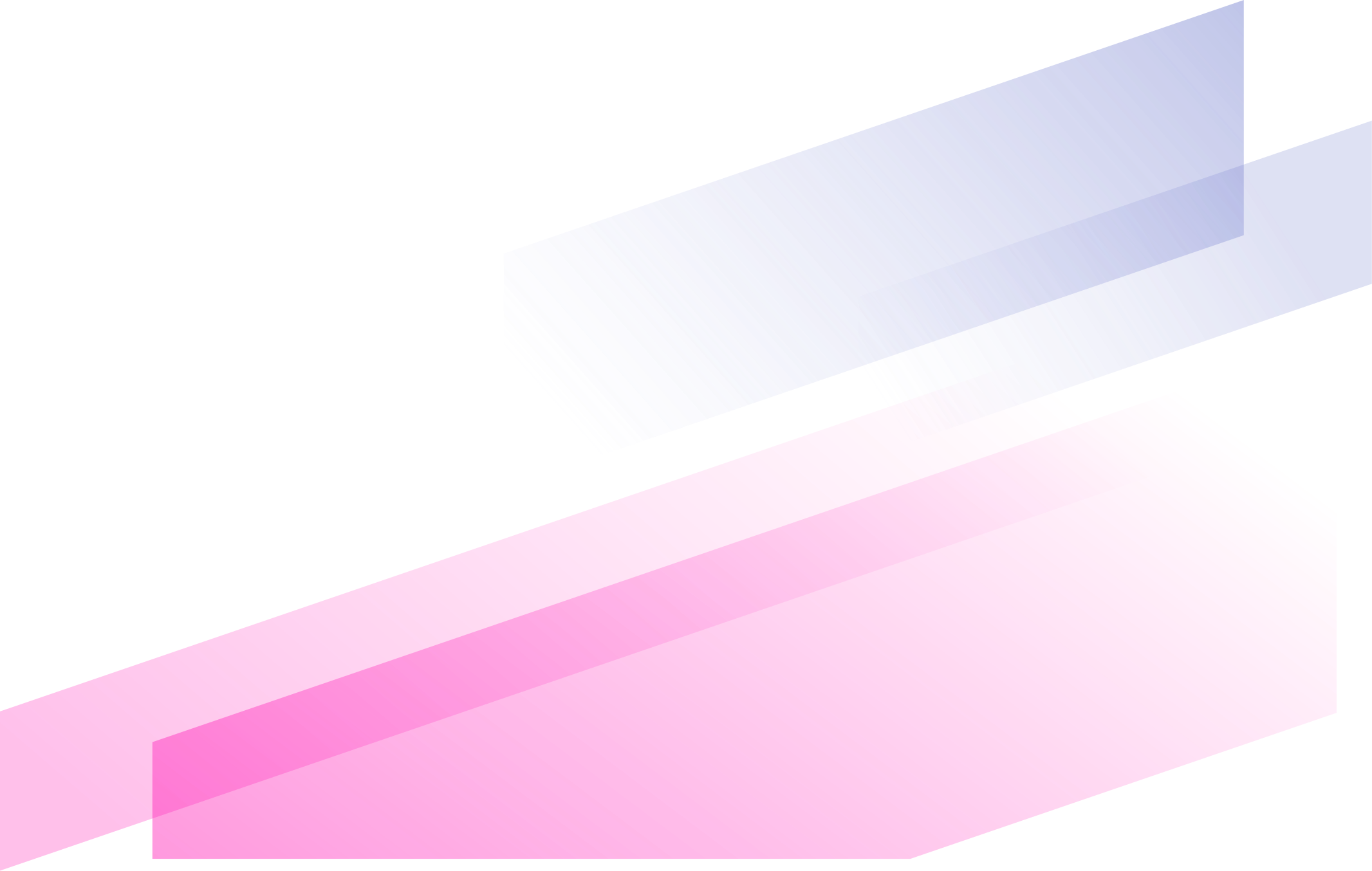
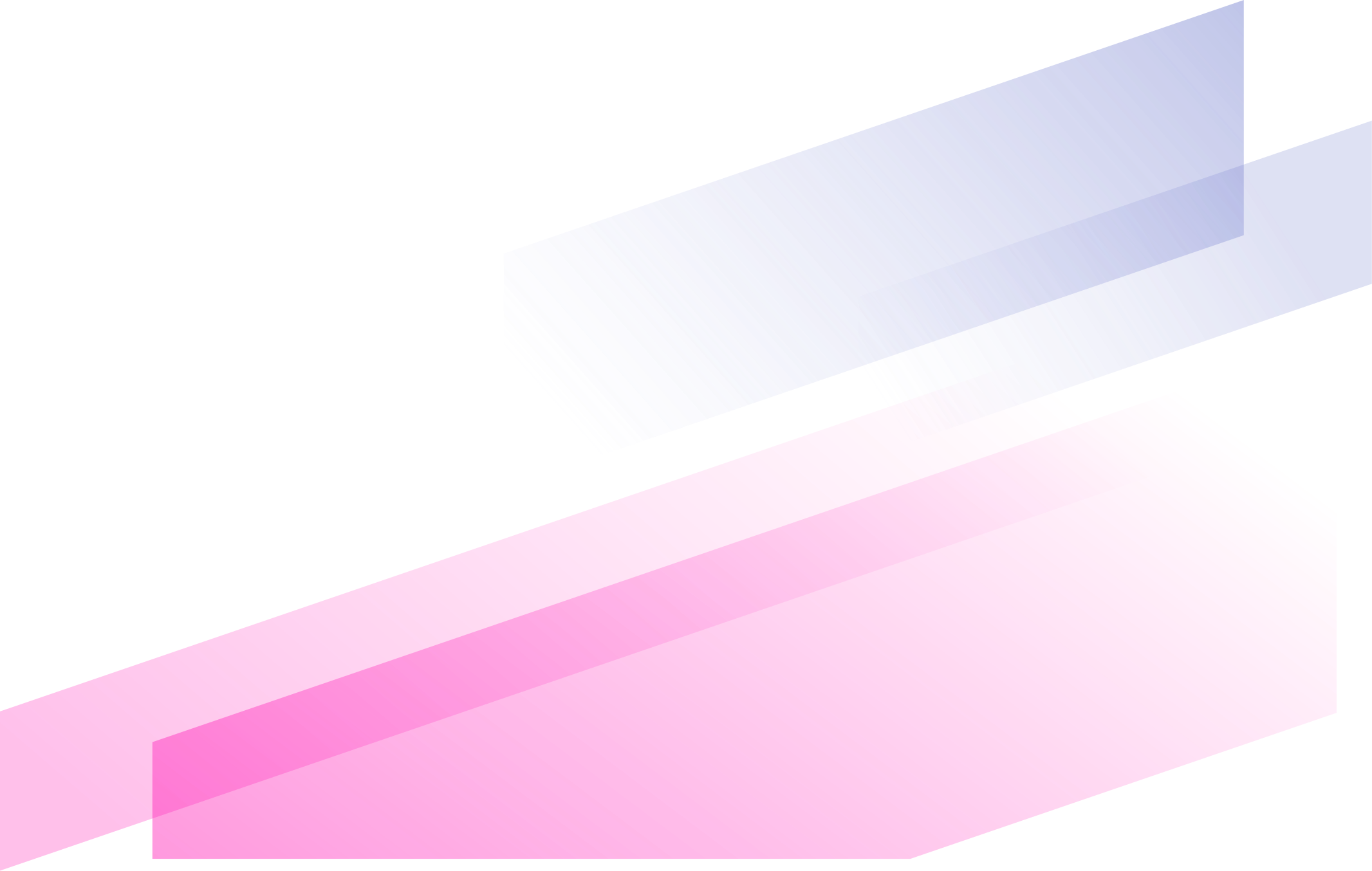
Looking beyond the hype: Applied AI and machine learning in translational medicine.
Auto-Generated Physiological Chain Data for an Ontological Framework for Pharmacology and Mechanism of Action to Determine Suspected Drugs in Cases of Dysuria.
Extracellular binding of indinavir to matrix metalloproteinase-2 and the alpha-7-nicotinic acetylcholine receptor: implications for use in cancer treatment.
alpha-Branched amines by catalytic 1,1-addition of C-H bonds and aminating agents to terminal alkenes.
Non-sedating antihistamines block G-protein-gated inwardly rectifying K(+) channels.
Executable pathway analysis using ensemble discrete-state modeling for large-scale data.
Skin Doctor: Machine Learning Models for Skin Sensitization Prediction that Provide Estimates and Indicators of Prediction Reliability.
Evaluating the consistency of large-scale pharmacogenomic studies.
Recent applications of deep learning and machine intelligence on in silico drug discovery: methods, tools and databases.
Network-based method for drug target discovery at the isoform level.