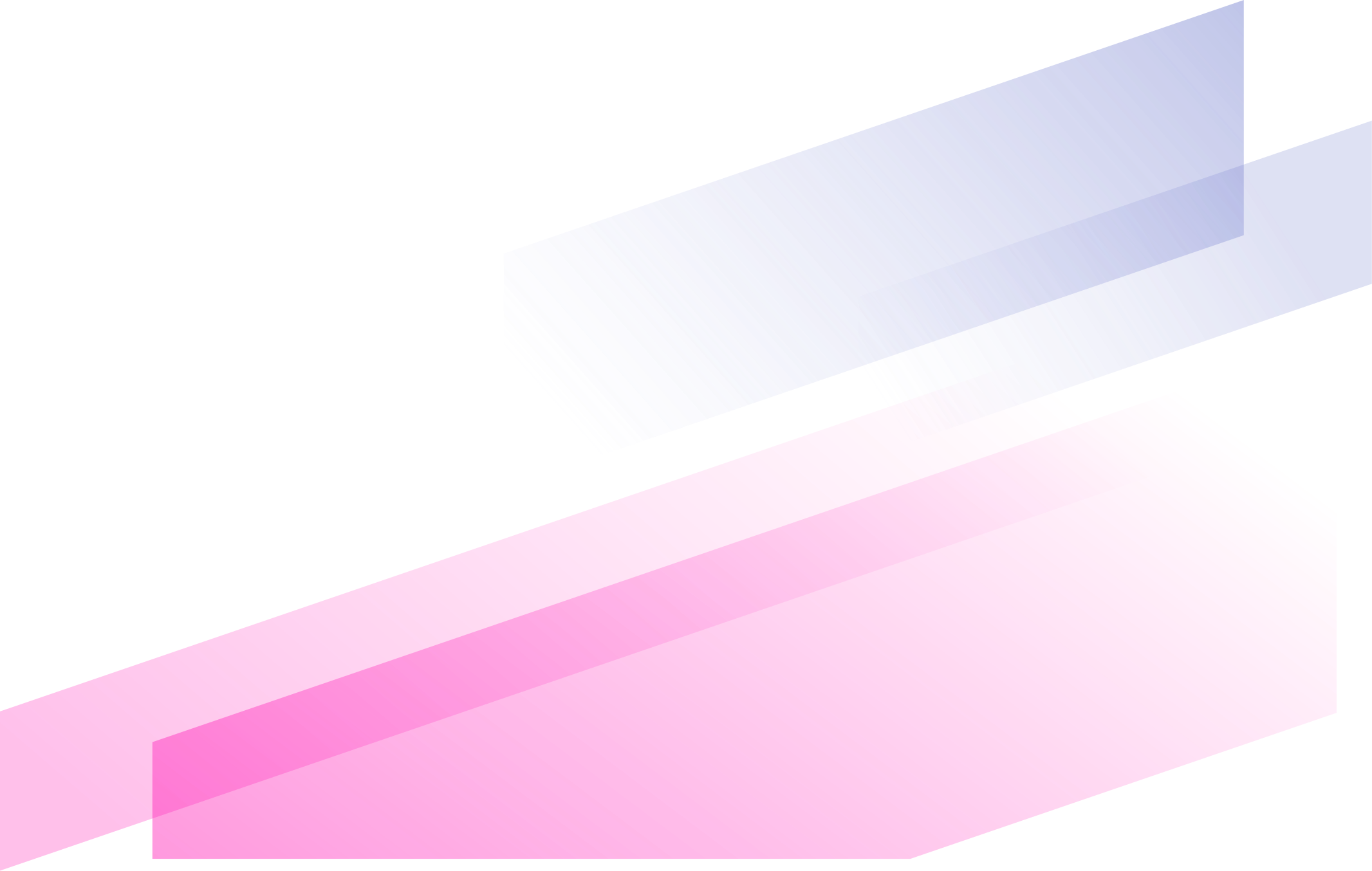
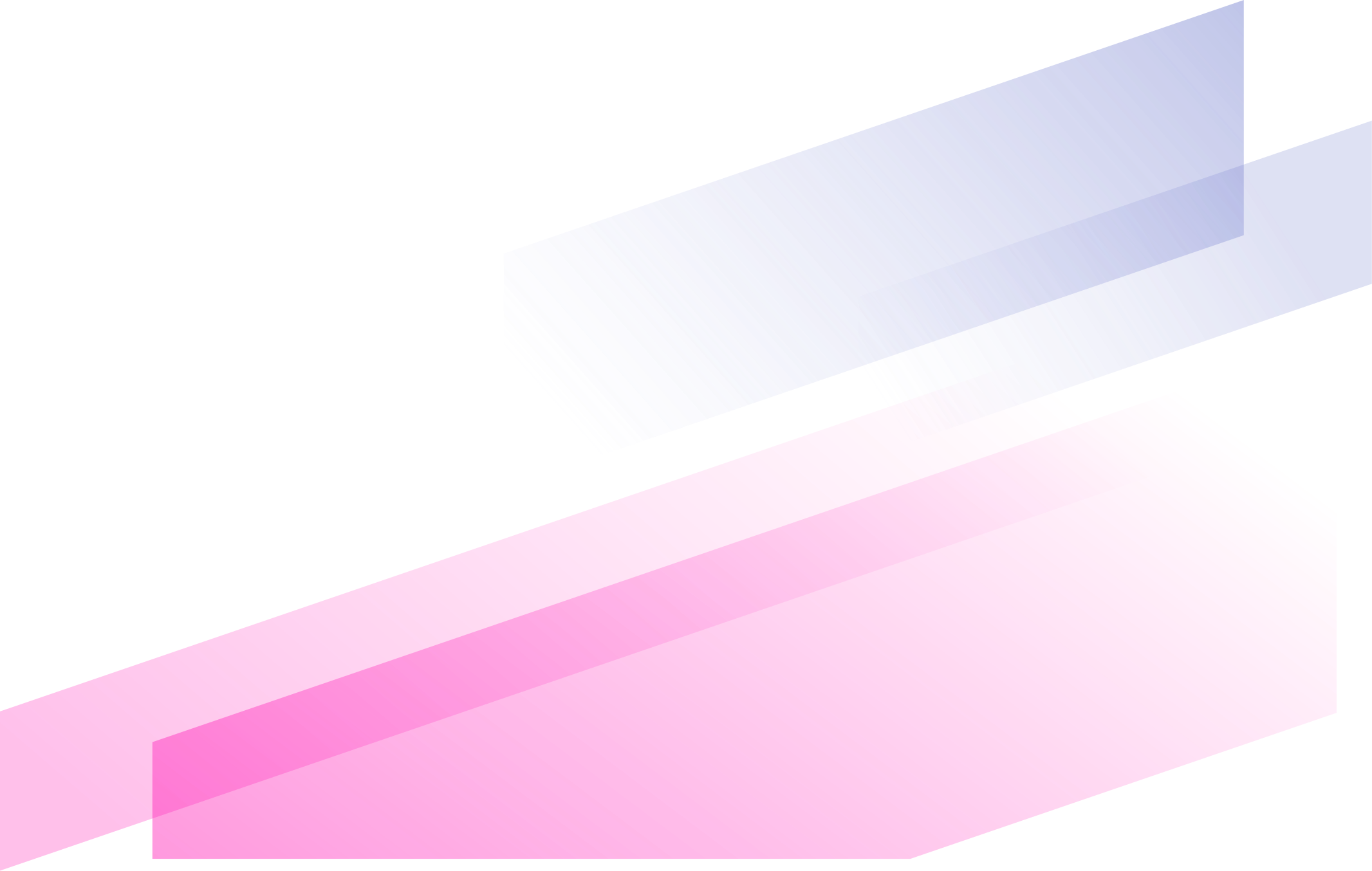
Global landscape of mouse and human cytokine transcriptional regulation.
A new chemoinformatics approach with improved strategies for effective predictions of potential drugs.
A Massively Parallel Selection of Small Molecule-RNA Motif Binding Partners Informs Design of an Antiviral from Sequence.
Context-enriched interactome powered by proteomics helps the identification of novel regulators of macrophage activation.
Screening of cytochrome P450 3A4 inhibitors via in silico and in vitro approaches.
Humanization of Antibodies using a Statistical Inference Approach.
A chronological pharmacovigilance network analytics approach for predicting adverse drug events.
Crystal structure of ethyl 4-[4-(di-methyl-amino)-phen-yl]-2,7,7-trimethyl-5-oxo-1,4,5,6,7,8-hexa-hydro-quin oline-3-carboxyl-ate.
Conformational Sampling of Macrocyclic Drugs in Different Environments: Can We Find the Relevant Conformations?
Inferences of individual drug responses across diverse cancer types using a novel competing endogenous RNA network.