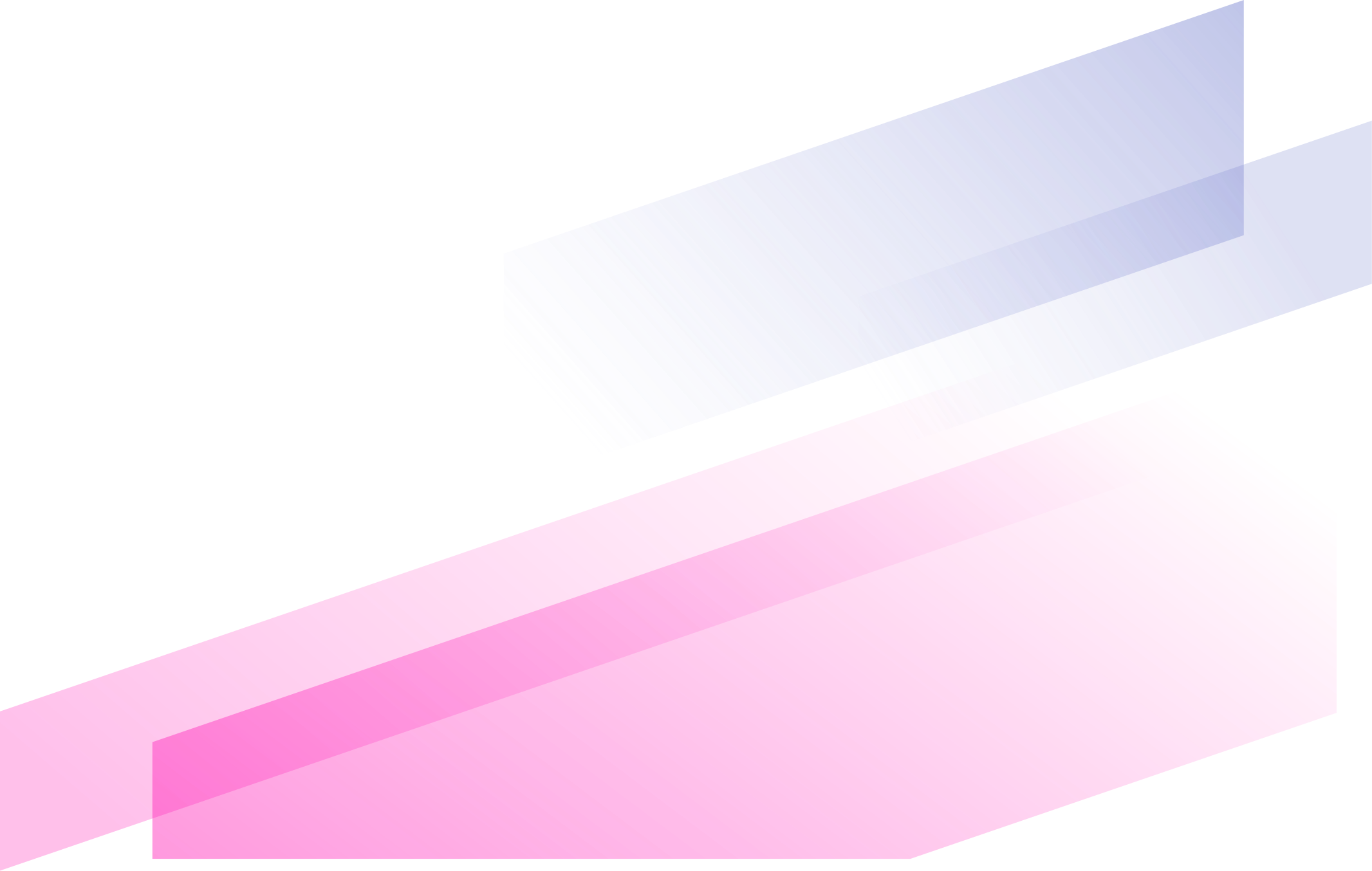
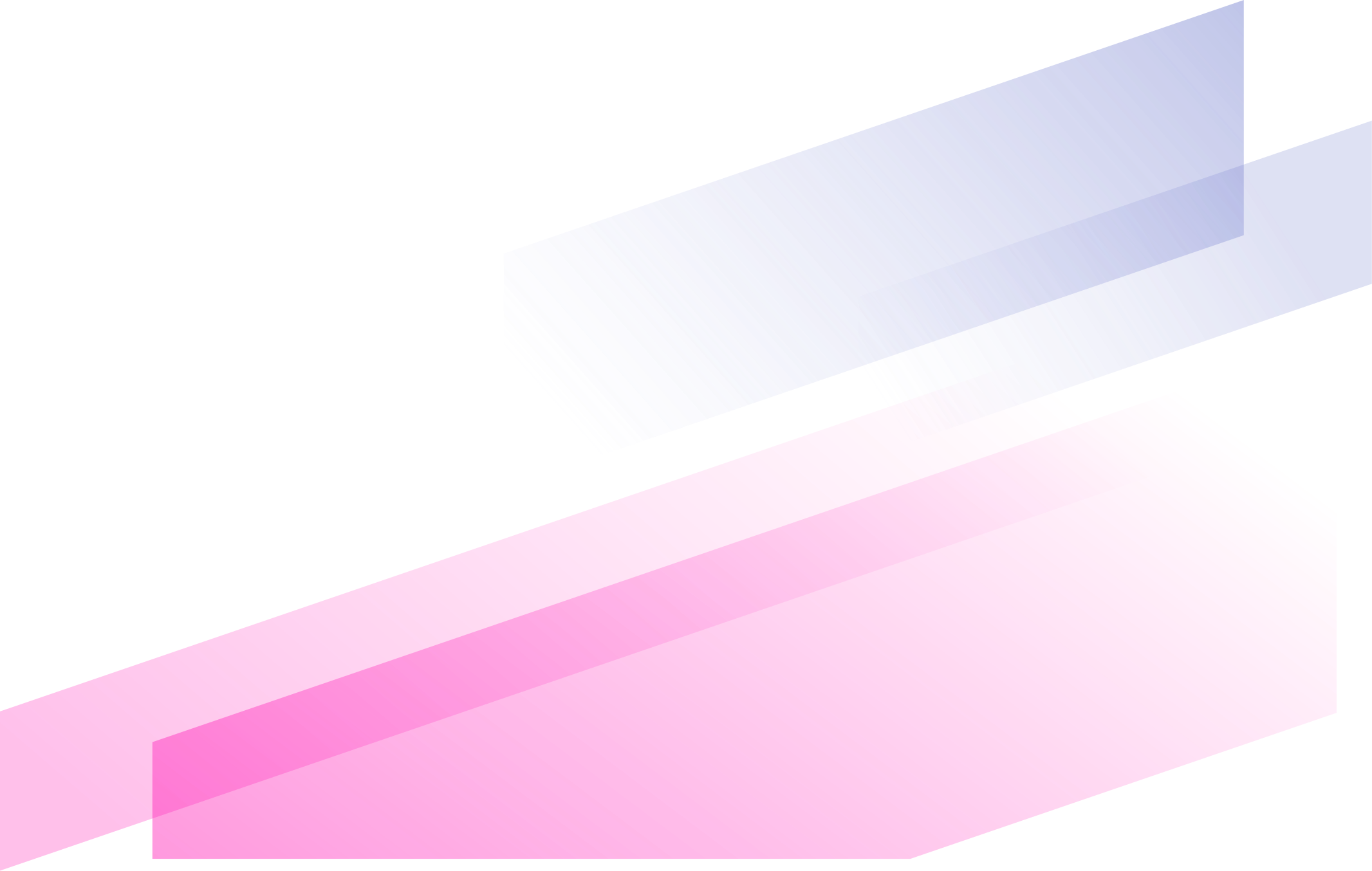
Drug Repurposing of Metabolic Agents in Malignant Glioma.
Sertraline, chlorprothixene, and chlorpromazine characteristically interact with the REST-binding site of the corepressor mSin3, showing medulloblastoma cell growth inhibitory activities.
A database of tissue-specific rhythmically expressed human genes has potential applications in circadian medicine.
Proteomics, Post-translational Modifications, and Integrative Analyses Reveal Molecular Heterogeneity within Medulloblastoma Subgroups.
Identification by virtual screening and functional characterisation of novel positive and negative allosteric modulators of the alpha7 nicotinic acetylcholine receptor.
Prediction of Novel Drugs and Diseases for Hepatocellular Carcinoma Based on Multi-Source Simulated Annealing Based Random Walk.
A systematic assessment of the availability and clinical drug information coverage of machine-readable clinical drug data sources for building knowledge translation products.
Machine Learning for Drug-Target Interaction Prediction.
Identification of drug target candidates of the swine pathogen Actinobacillus pleuropneumoniae by construction of protein-protein interaction network.
PregOMICS-Leveraging systems biology and bioinformatics for drug repurposing in maternal-child health.