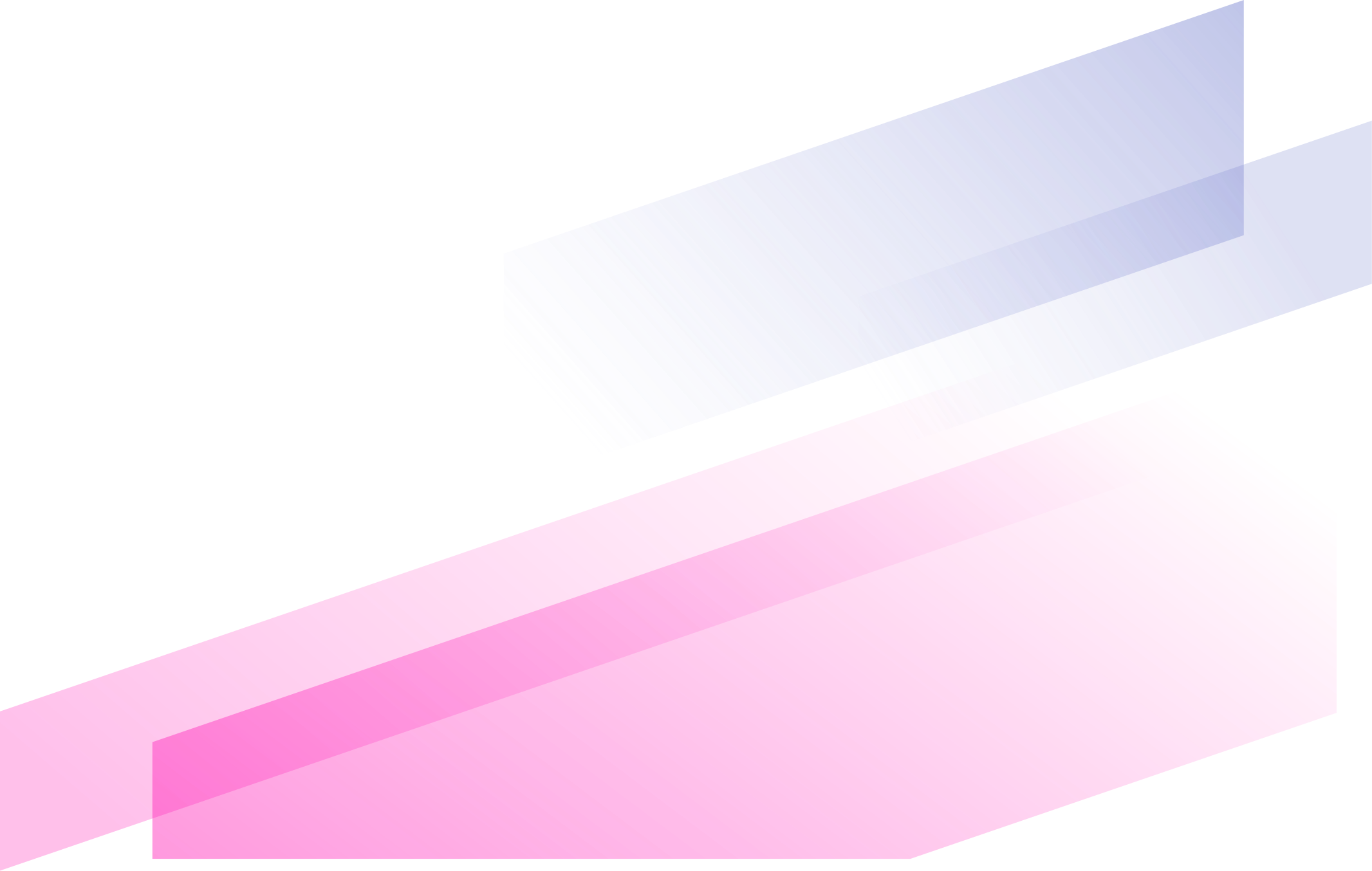
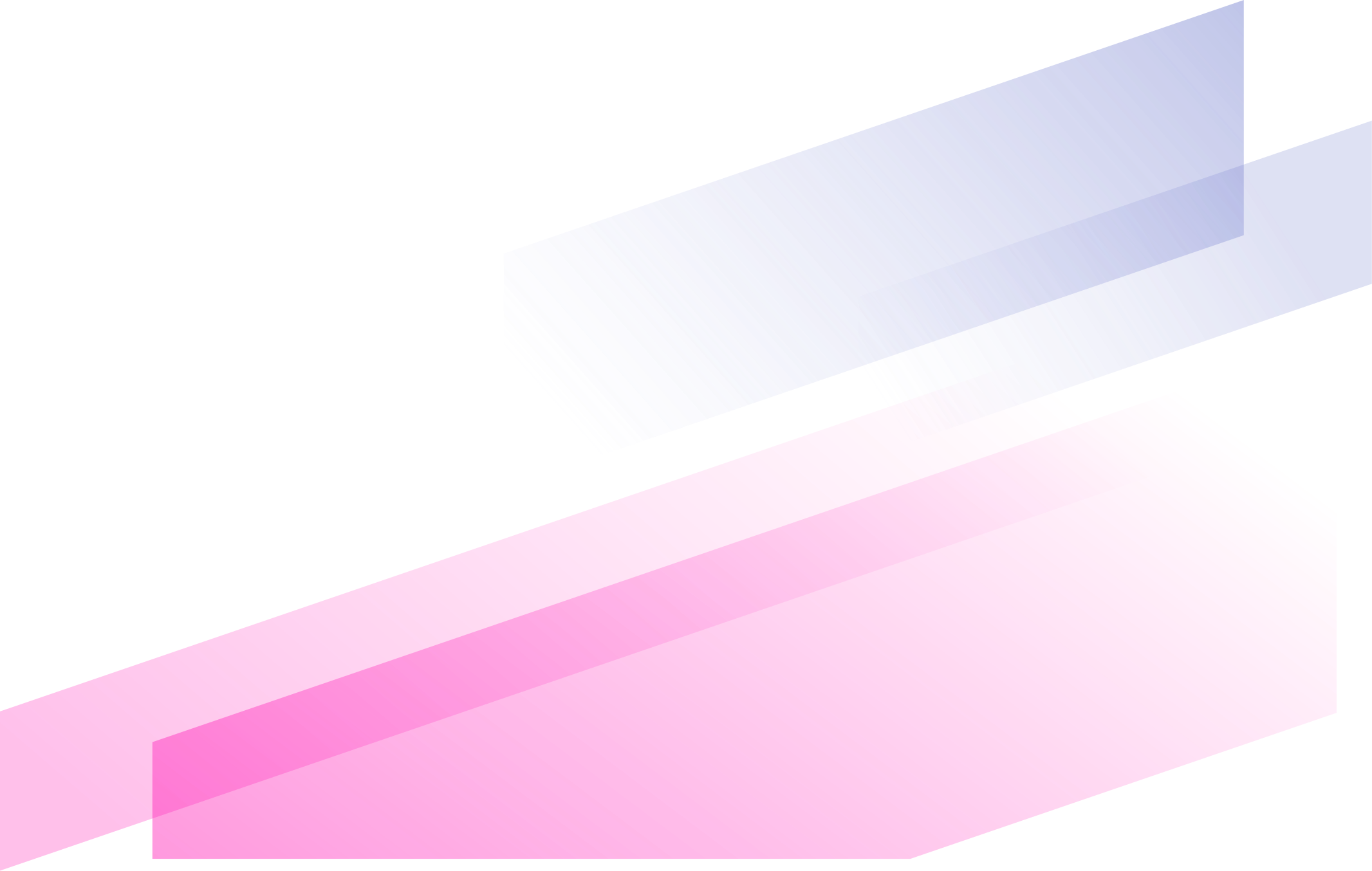
Drug target-gene signatures that predict teratogenicity are enriched for developmentally related genes.
Increased diversity of libraries from libraries: chemoinformatic analysis of bis-diazacyclic libraries.
Combinations of protein-chemical complex structures reveal new targets for established drugs.
Natural products as DNA methyltransferase inhibitors: a computer-aided discovery approach.
Exploring the metabolic network of the epidemic pathogen Burkholderia cenocepacia J2315 via genome-scale reconstruction.
Predicting drug side-effect profiles: a chemical fragment-based approach.
The Translational Medicine Ontology and Knowledge Base: driving personalized medicine by bridging the gap between bench and bedside.
Linked open drug data for pharmaceutical research and development.
Discrimination of approved drugs from experimental drugs by learning methods.
Analysis of in vitro bioactivity data extracted from drug discovery literature and patents: Ranking 1654 human protein targets by assayed compounds and molecular scaffolds.