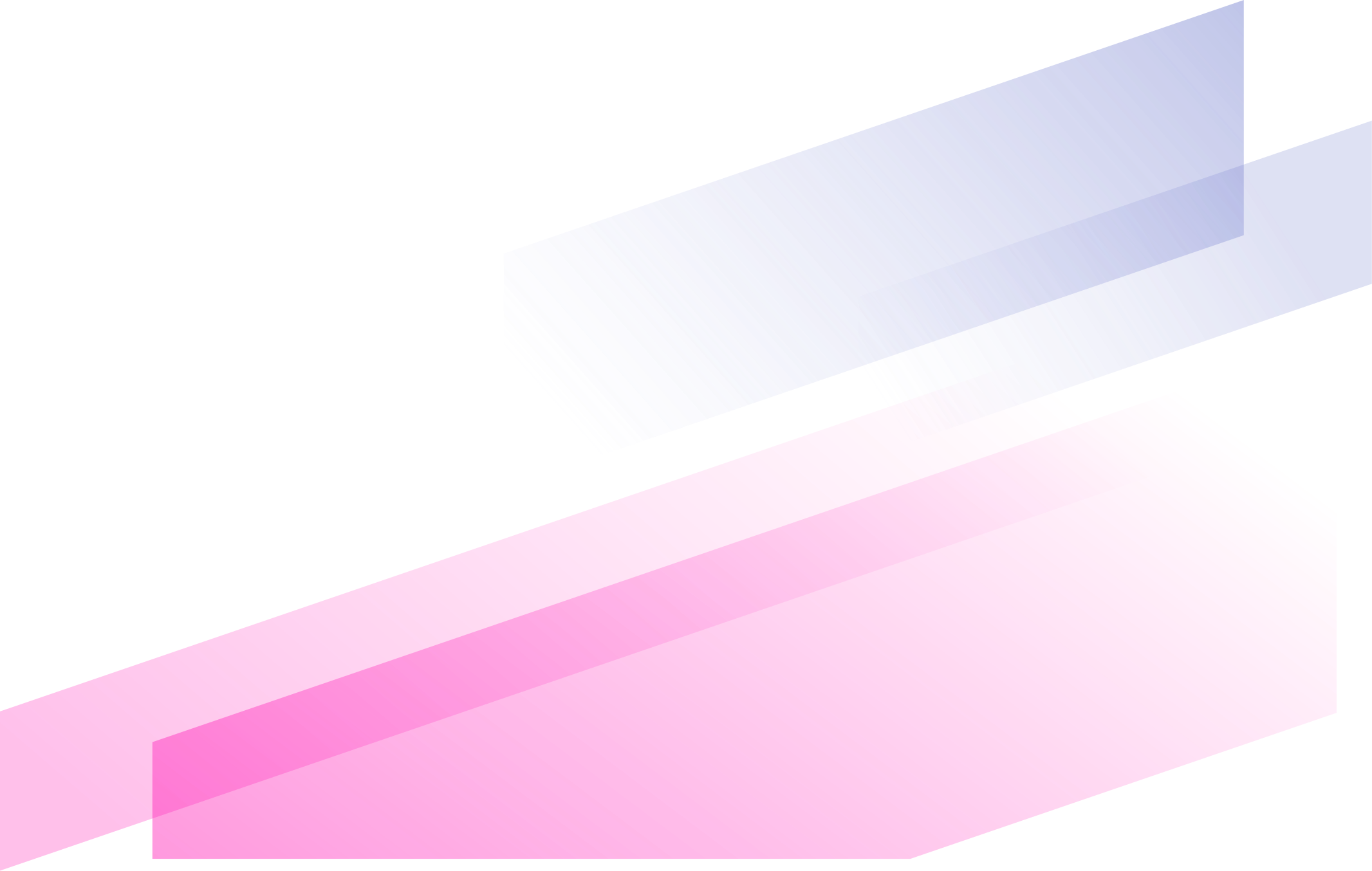
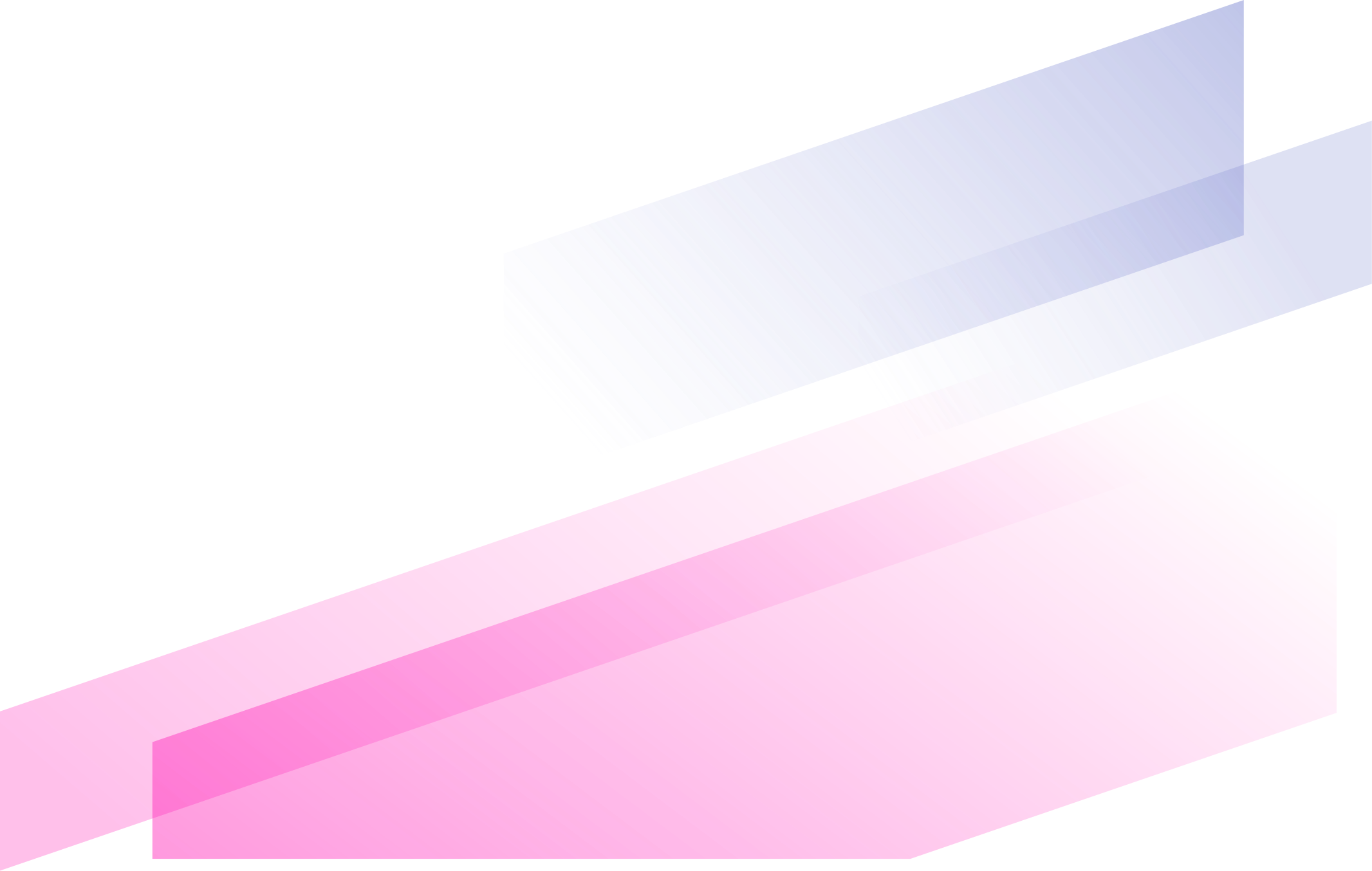
RepTB: a gene ontology based drug repurposing approach for tuberculosis.
The impact of pharmacokinetic gene profiles across human cancers.
VAReporter: variant reporter for cancer research of massive parallel sequencing.
Identification of new EphA4 inhibitors by virtual screening of FDA-approved drugs.
In silico identification of protein targets for chemical neurotoxins using ToxCast in vitro data and read-across within the QSAR toolbox.
Genetic Mechanisms of Asthma and the Implications for Drug Repositioning.
Deep learning improves prediction of drug-drug and drug-food interactions.
Quantitative self-assembly prediction yields targeted nanomedicines.
A systems medicine approach reveals disordered immune system and lipid metabolism in multiple sclerosis patients.
Combining Similarity Searching and Network Analysis for the Identification of Active Compounds.