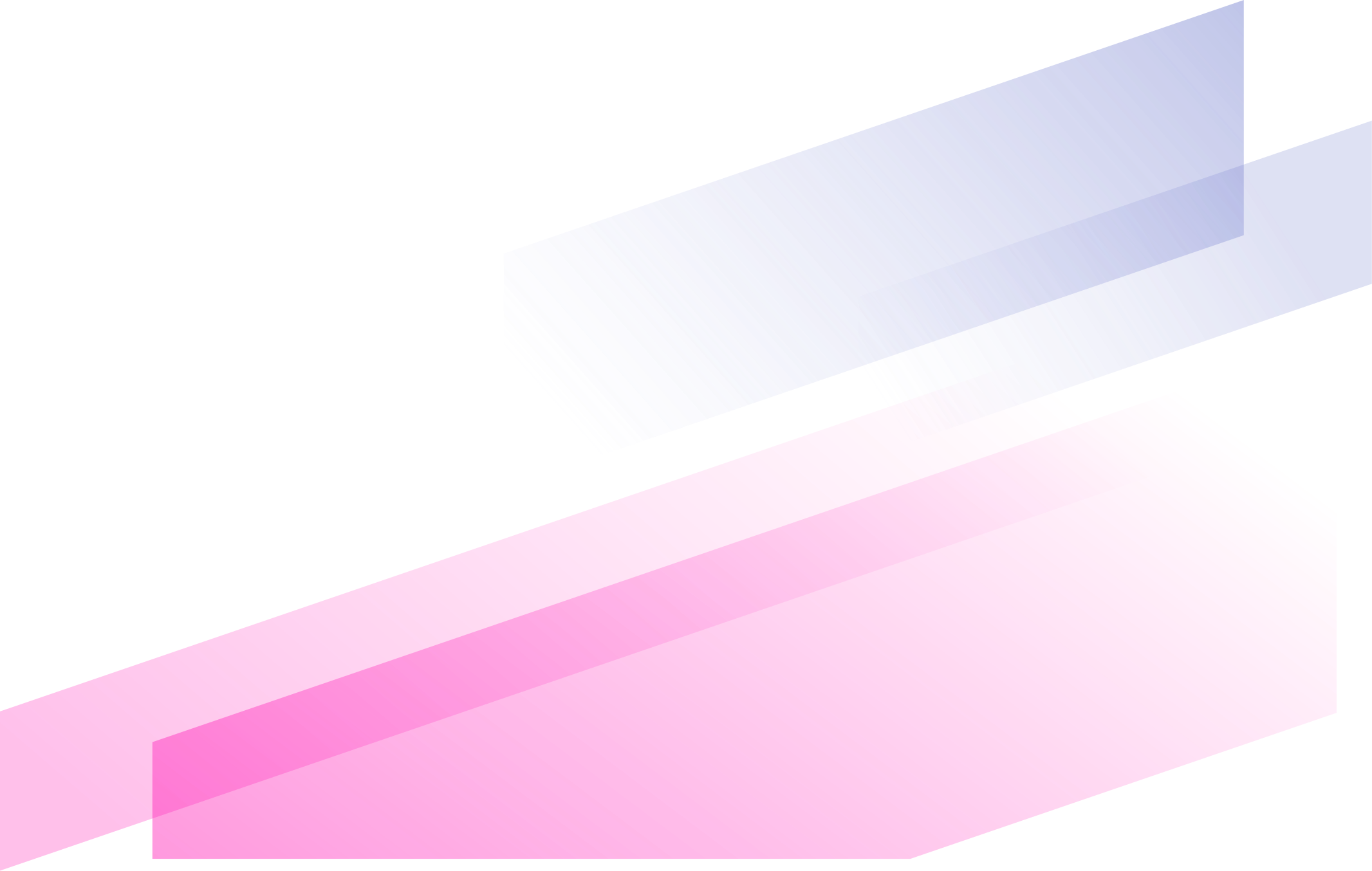
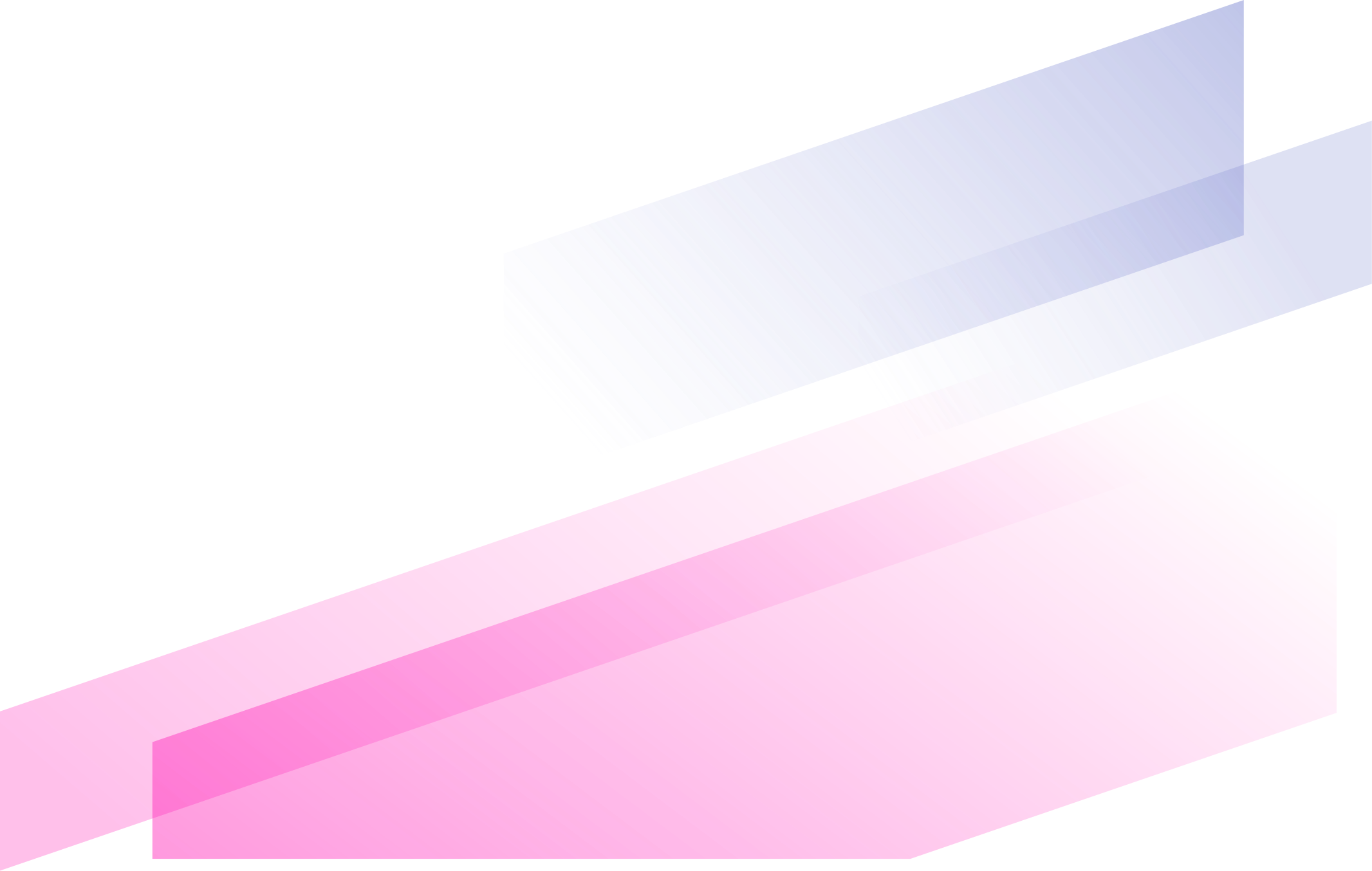
PDBsum: Structural summaries of PDB entries.
Epigenomics alternations and dynamic transcriptional changes in responses to 5-fluorouracil stimulation reveal mechanisms of acquired drug resistance of colorectal cancer cells.
Clinical information extraction applications: A literature review.
Precursor-directed combinatorial biosynthesis of cephalosporin analogue by endolithic actinobacterium Streptomyces sp. AL51 by utilizing thiophene derivative.
Adverse drug reactions triggered by the common HLA-B*57:01 variant: virtual screening of DrugBank using 3D molecular docking.
Discovery of VEGFR2 inhibitors by integrating naive Bayesian classification, molecular docking and drug screening approaches.
Beyond genomics: understanding exposotypes through metabolomics.
In silico profiling of systemic effects of drugs to predict unexpected interactions.
Deep learning of mutation-gene-drug relations from the literature.
Gene annotation bias impedes biomedical research.