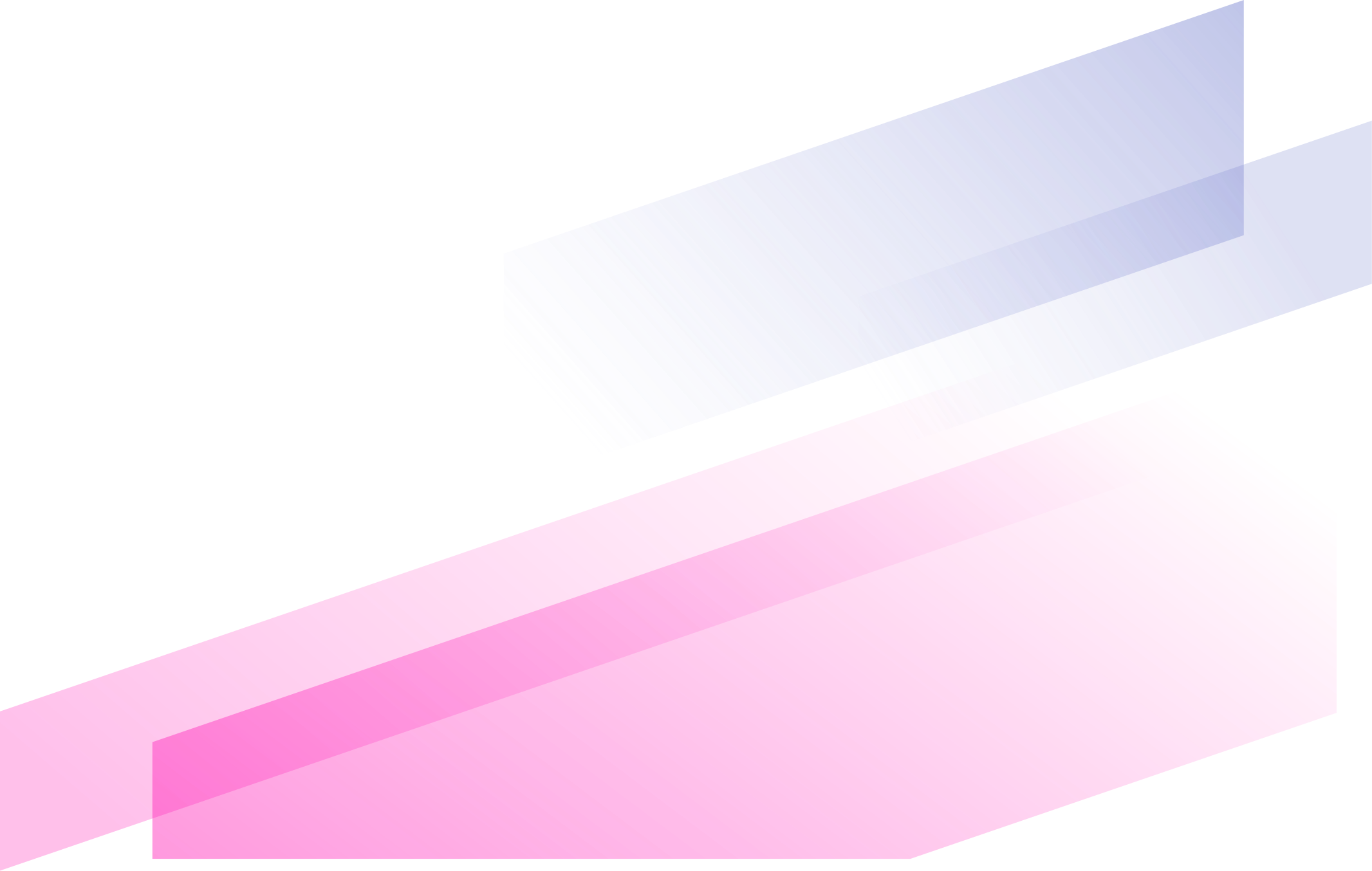
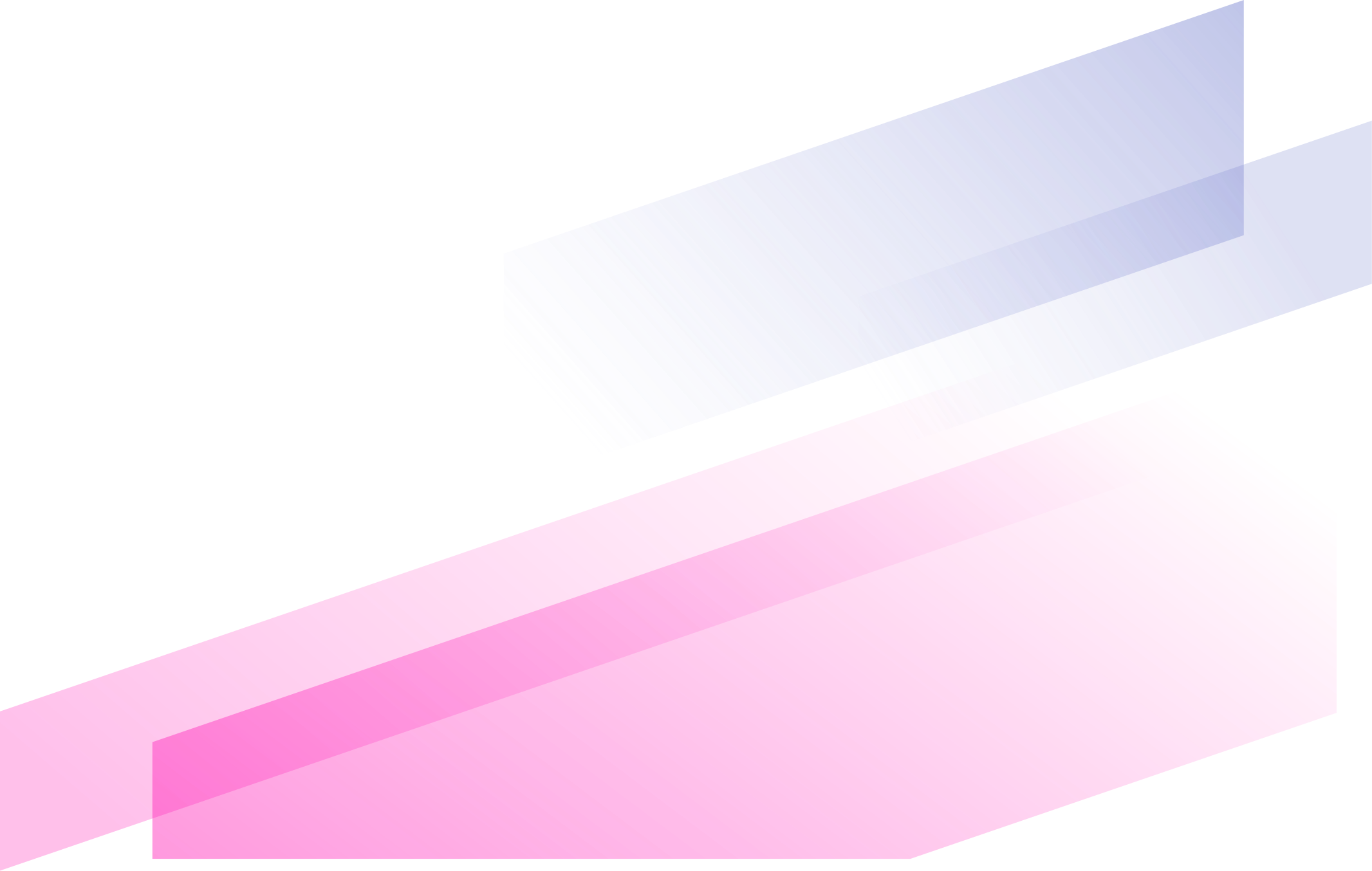
Structure elucidation and in silico docking studies of a novel furopyrimidine antibiotics synthesized by endolithic bacterium Actinomadura sp. AL2.
A network integration approach for drug-target interaction prediction and computational drug repositioning from heterogeneous information.
Critical Roles of Dual-Specificity Phosphatases in Neuronal Proteostasis and Neurological Diseases.
MediSyn: uncertainty-aware visualization of multiple biomedical datasets to support drug treatment selection.
In silico prediction of drug-target interaction networks based on drug chemical structure and protein sequences.
Molecular fingerprinting of principal neurons in the rodent hippocampus: A neuroinformatics approach.
Comparative Pharmacokinetic Study for Linezolid and Two Novel Antibacterial Oxazolidinone Derivatives in Rabbits: Can Differences in the Pharmacokinetic Properties Explain the Discrepancies between Their In Vivo and In Vitro Antibacterial Activities?
Molecular modeling and structure-based drug discovery approach reveals protein kinases as off-targets for novel anticancer drug RH1.
Controlling Directed Protein Interaction Networks in Cancer.
Extracting Drug-Drug Interactions with Word and Character-Level Recurrent Neural Networks.