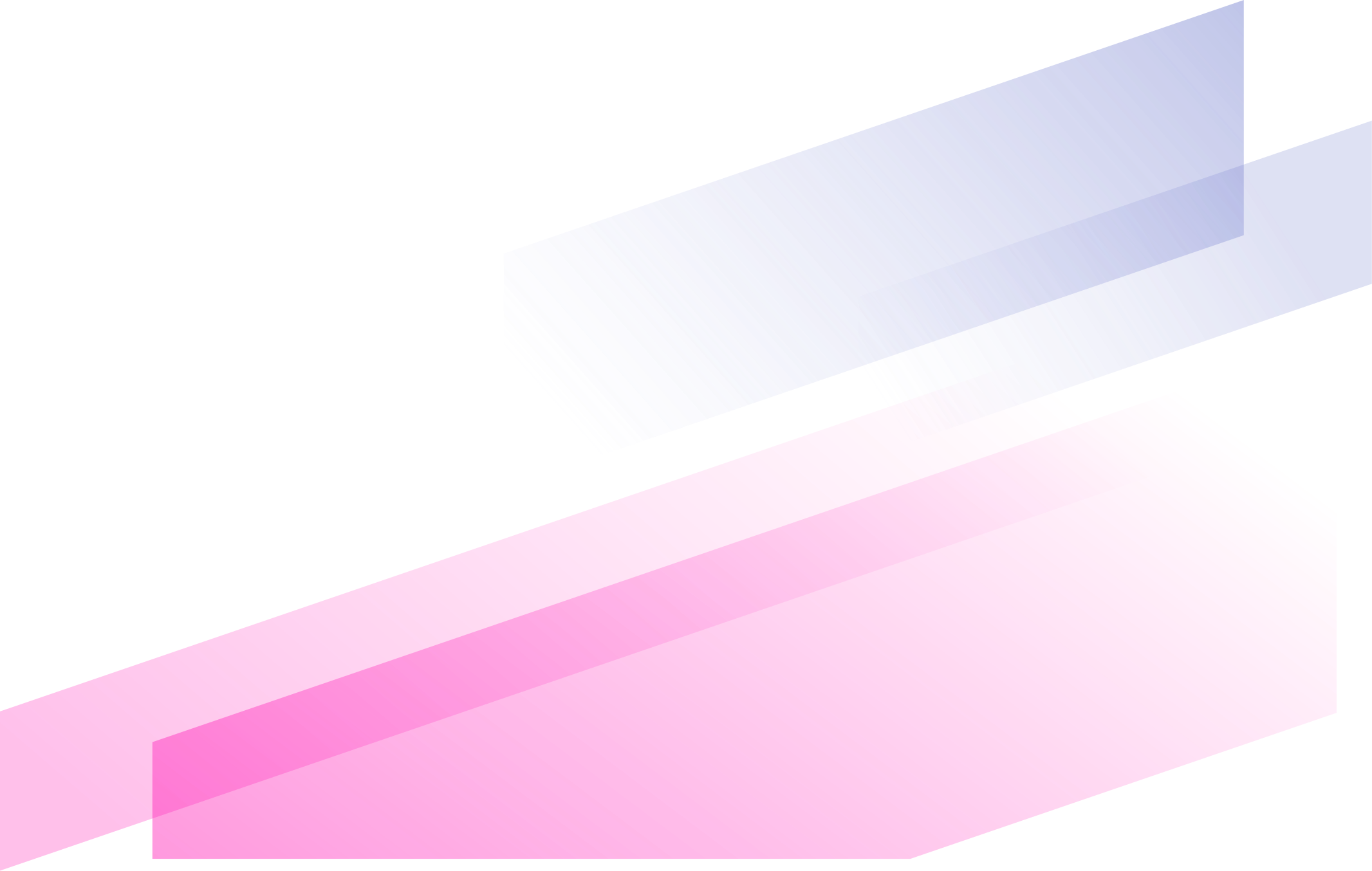
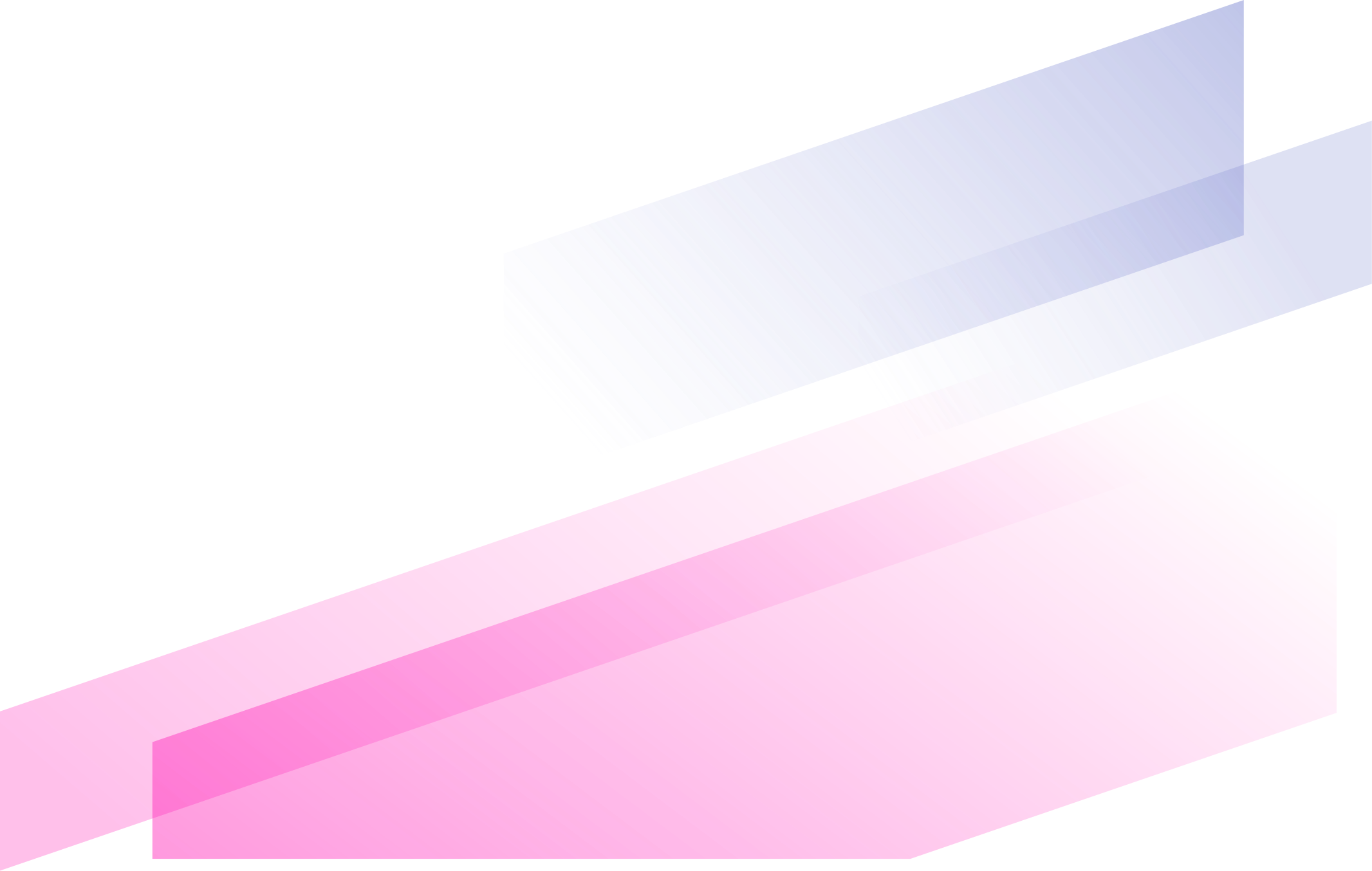
A Comparative Analysis of Drug-Induced Hepatotoxicity in Clinically Relevant Situations.
Monitoring of adherence to headache treatments by means of hair analysis.
Chembench: A Publicly Accessible, Integrated Cheminformatics Portal.
Systematic Identification and Assessment of Therapeutic Targets for Breast Cancer Based on Genome-Wide RNA Interference Transcriptomes.
Human enterovirus 71 protein interaction network prompts antiviral drug repositioning.
Deep Learning to Predict the Formation of Quinone Species in Drug Metabolism.
HAPPI-2: a Comprehensive and High-quality Map of Human Annotated and Predicted Protein Interactions.
Accelerating the semisynthesis of alkaloid-based drugs through metabolic engineering.
Green Synthesis of Halogenated Thiophenes, Selenophenes and Benzo[b]selenophenes Using Sodium Halides as a Source of Electrophilic Halogens.
Systematic Analyses and Prediction of Human Drug Side Effect Associated Proteins from the Perspective of Protein Evolution.