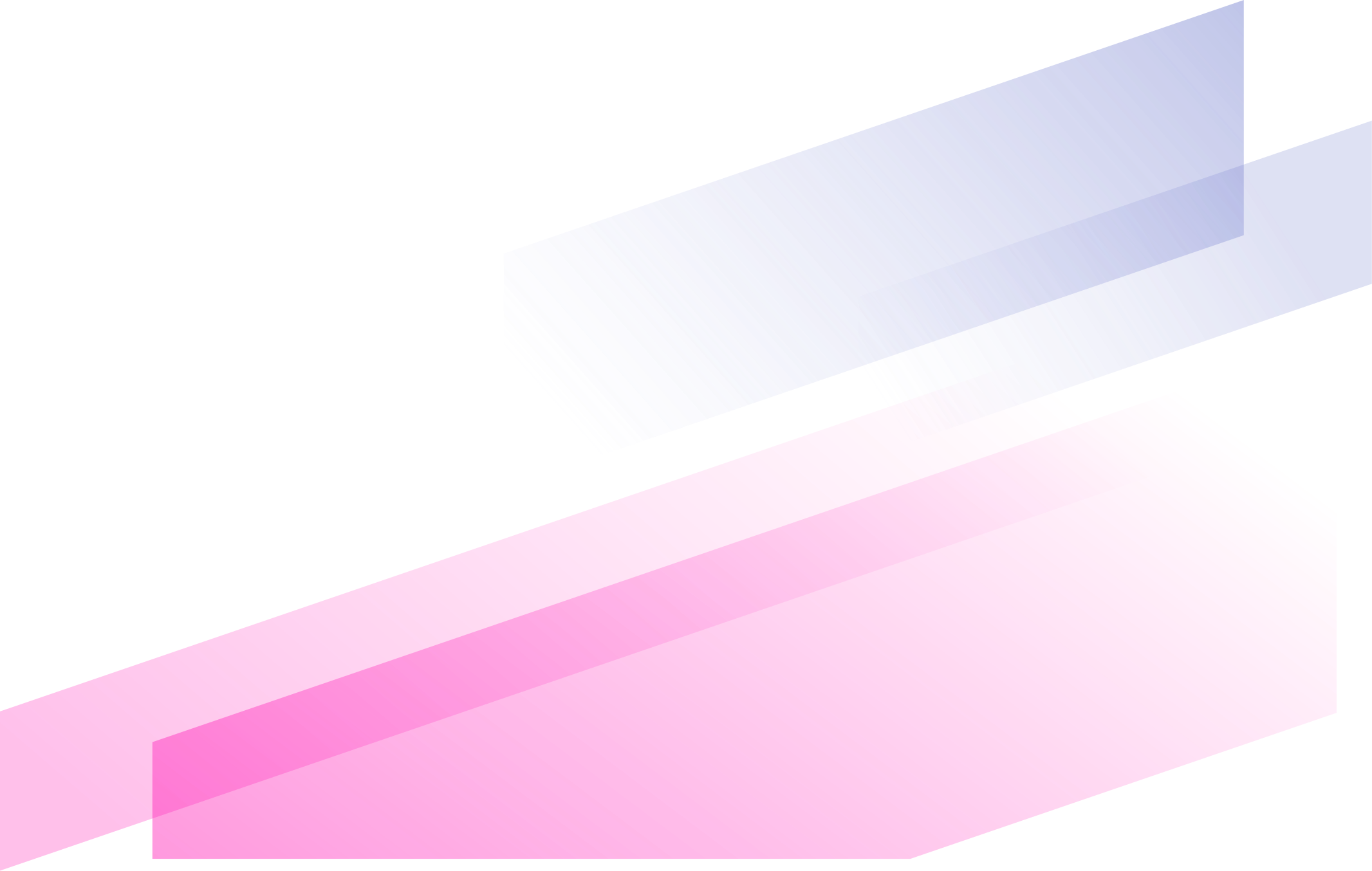
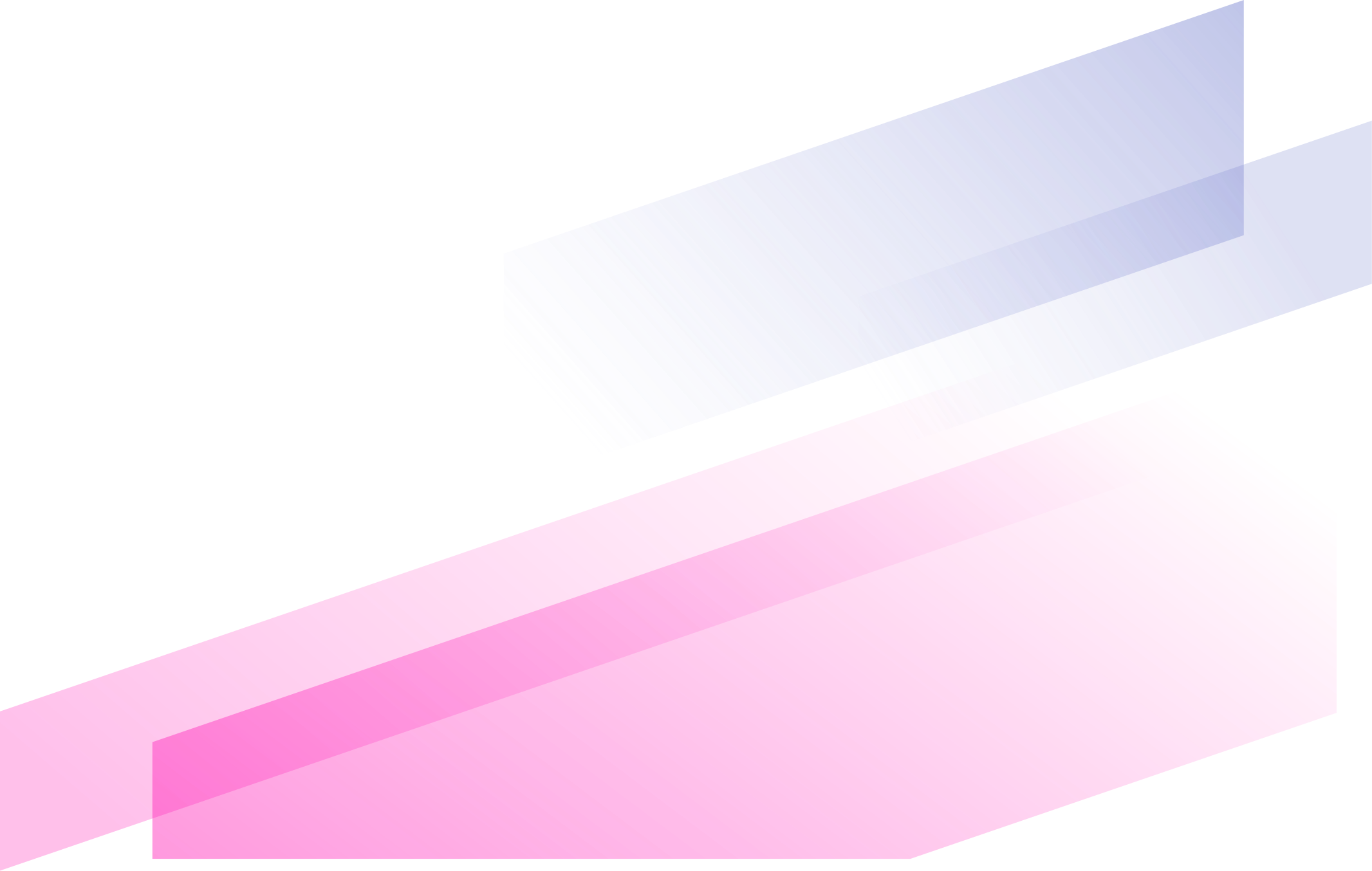
The Chemical Validation and Standardization Platform (CVSP): large-scale automated validation of chemical structure datasets.
Quercetin Influences Quorum Sensing in Food Borne Bacteria: In-Vitro and In-Silico Evidence.
Optimizing drug-target interaction prediction based on random walk on heterogeneous networks.
Cancer based pharmacogenomics network supported with scientific evidences: from the view of drug repurposing.
Computational drug repositioning for peripheral arterial disease: prediction of anti-inflammatory and pro-angiogenic therapeutics.
Assessing the Need of Discourse-Level Analysis in Identifying Evidence of Drug-Disease Relations in Scientific Literature.
A functional biological network centered on XRCC3: a new possible marker of chemoradiotherapy resistance in rectal cancer patients.
Potential Compounds for Oral Cancer Treatment: Resveratrol, Nimbolide, Lovastatin, Bortezomib, Vorinostat, Berberine, Pterostilbene, Deguelin, Andrographolide, and Colchicine.
Extraction of pharmacokinetic evidence of drug-drug interactions from the literature.
The apparent permeabilities of Caco-2 cells to marketed drugs: magnitude, and independence from both biophysical properties and endogenite similarities.