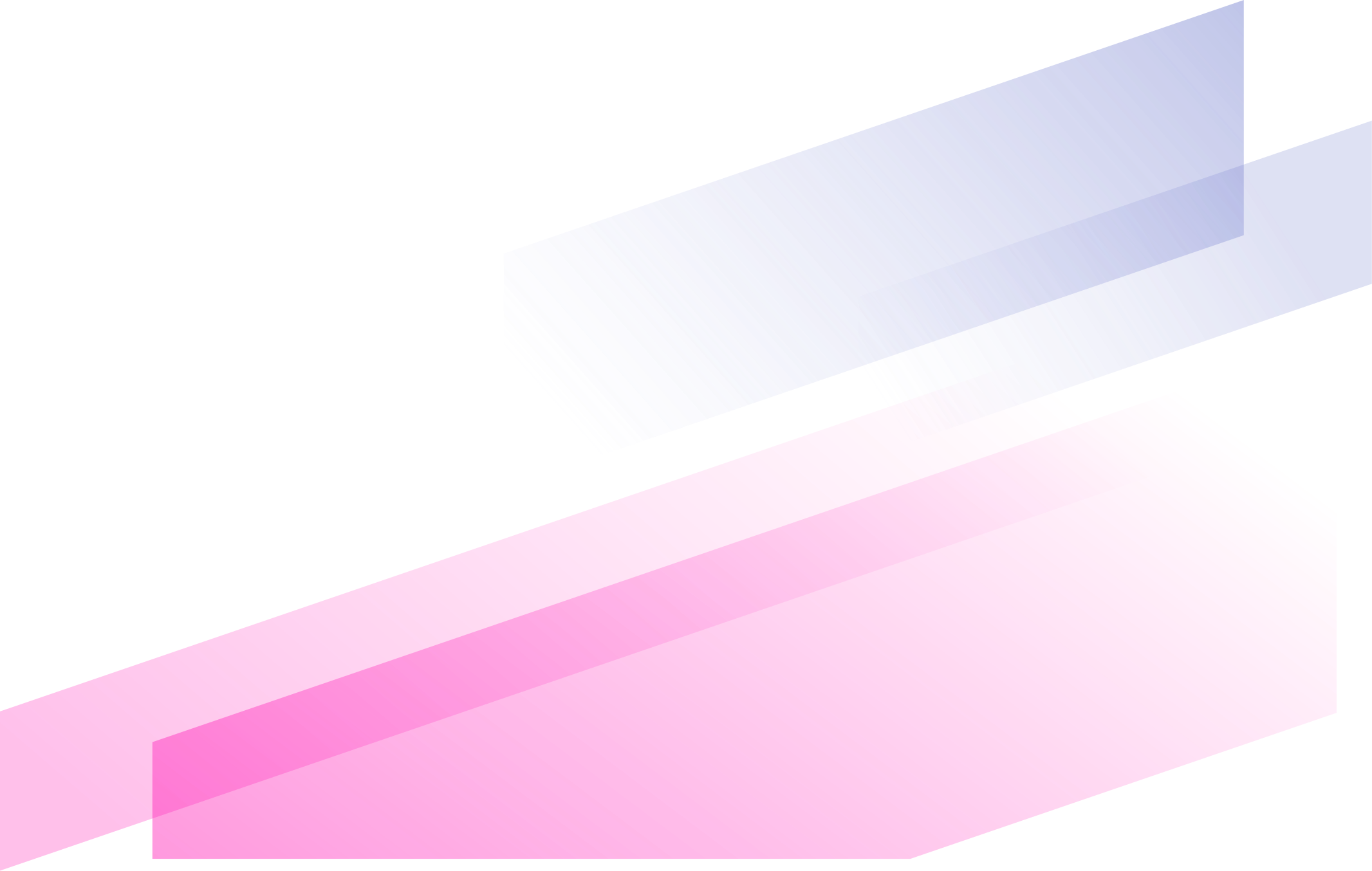
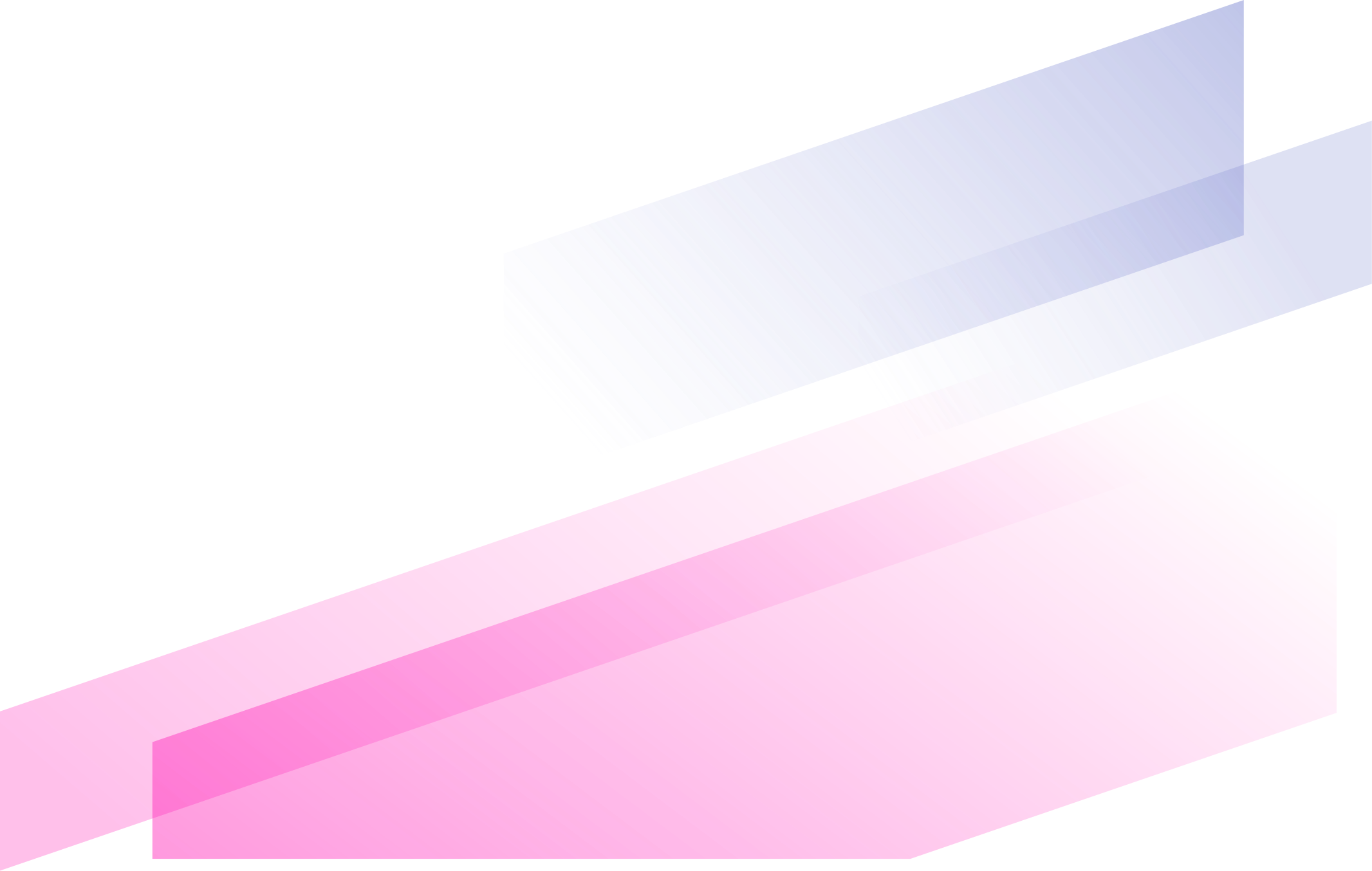
An automatic system to identify heart disease risk factors in clinical texts over time.
Conceptual Knowledge Discovery in Databases for Drug Combinations Predictions in Malignant Melanoma.
Evaluating drug-drug interaction information in NDF-RT and DrugBank.
FN14 and GRP94 expression are prognostic/predictive biomarkers of brain metastasis outcome that open up new therapeutic strategies.
PROXIMAL: a method for Prediction of Xenobiotic Metabolism.
Dintor: functional annotation of genomic and proteomic data.
Pharmacophore Models and Pharmacophore-Based Virtual Screening: Concepts and Applications Exemplified on Hydroxysteroid Dehydrogenases.
Predicting target proteins for drug candidate compounds based on drug-induced gene expression data in a chemical structure-independent manner.
Can physicochemical properties of antimicrobials be used to predict their pharmacokinetics during extracorporeal membrane oxygenation? Illustrative data from ovine models.
An integrated structure- and system-based framework to identify new targets of metabolites and known drugs.