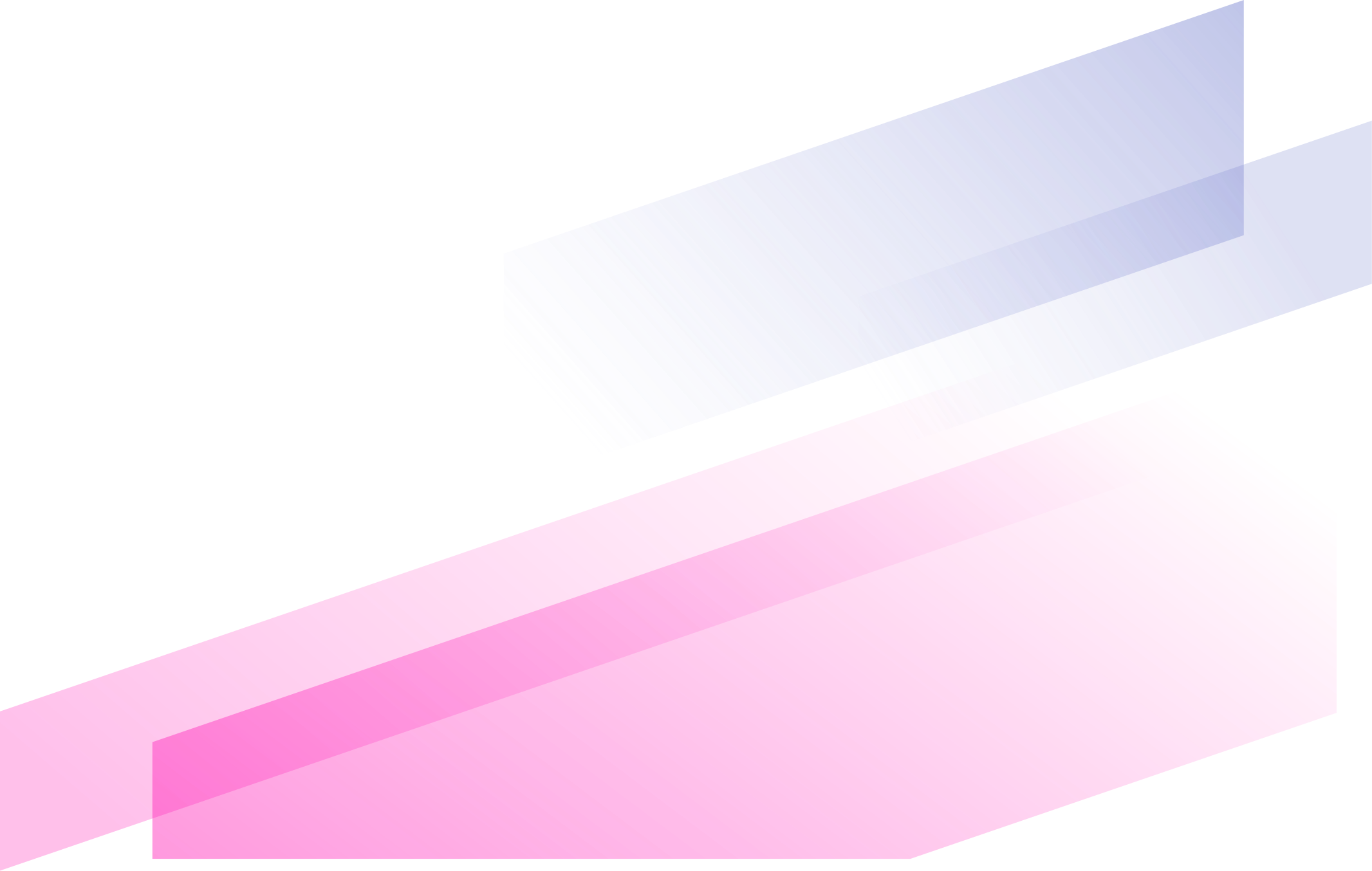
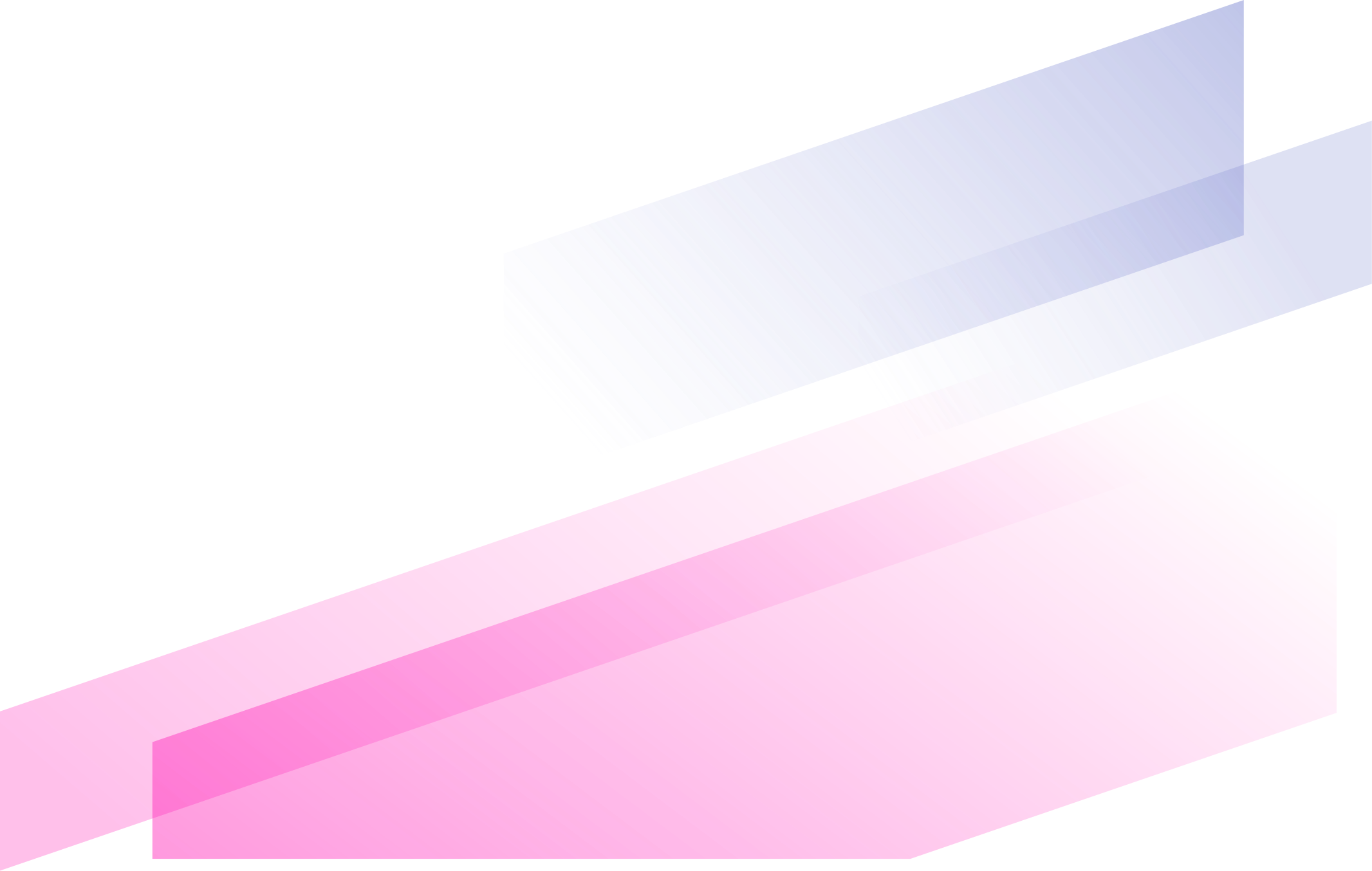
An integrated network platform for contextual prioritization of drugs and pathways.
Integrated Model of Chemical Perturbations of a Biological Pathway Using 18 In Vitro High-Throughput Screening Assays for the Estrogen Receptor.
Identification of potential inhibitors based on compound proposal contest: Tyrosine-protein kinase Yes as a target.
Inhibition by small-molecule ligands of formation of amyloid fibrils of an immunoglobulin light chain variable domain.
A Systems Biology-Based Investigation into the Pharmacological Mechanisms of Sheng-ma-bie-jia-tang Acting on Systemic Lupus Erythematosus by Multi-Level Data Integration.
Methods for biological data integration: perspectives and challenges.
Predicting drug side effects by multi-label learning and ensemble learning.
Design of chemical space networks using a Tanimoto similarity variant based upon maximum common substructures.
Publisher's Note:Abstraction for data integration:Fusing mammalian molecular, cellular and phenotype big datasets for better knowledge extraction.
Network analysis of gene essentiality in functional genomics experiments.