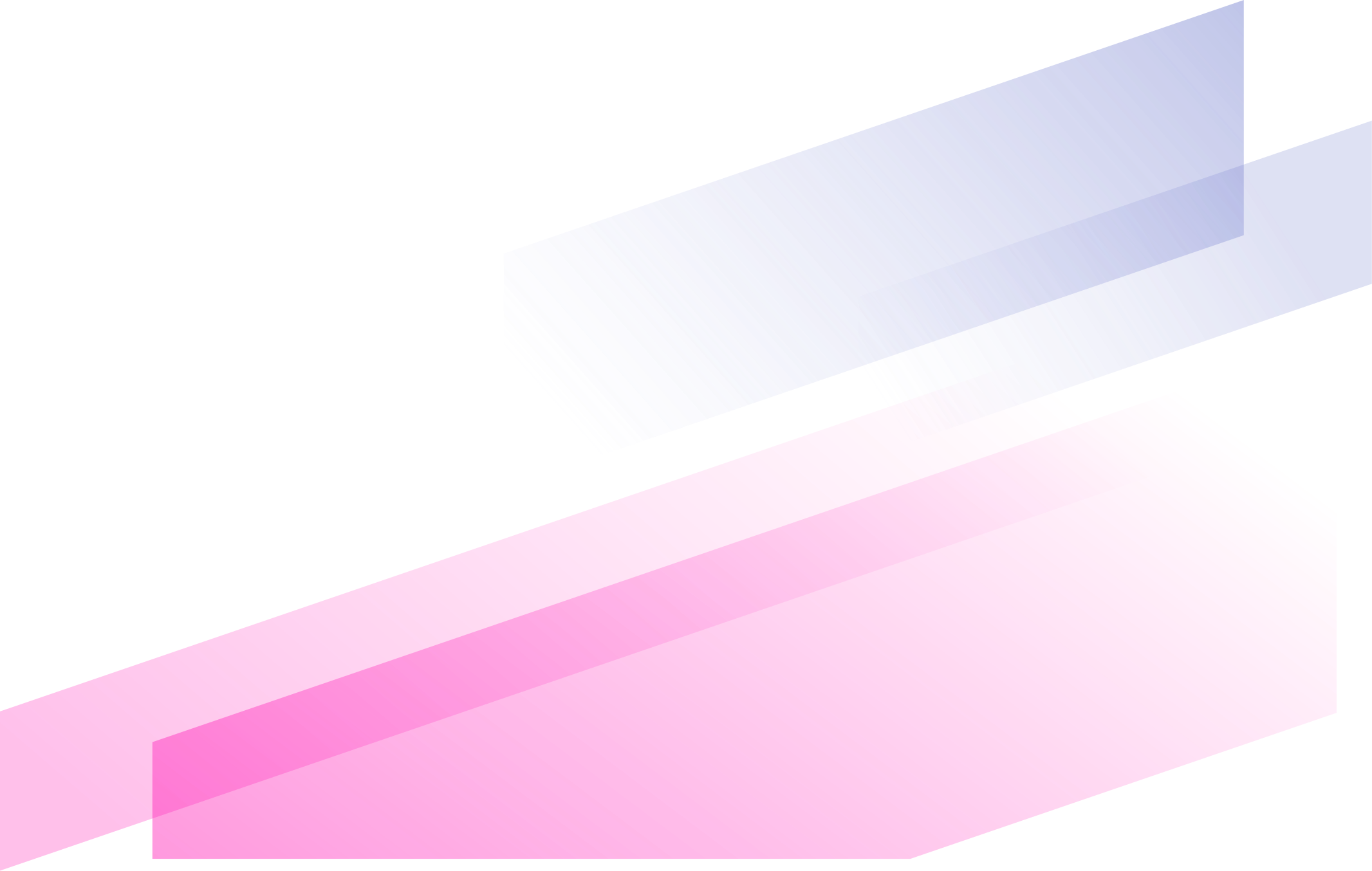
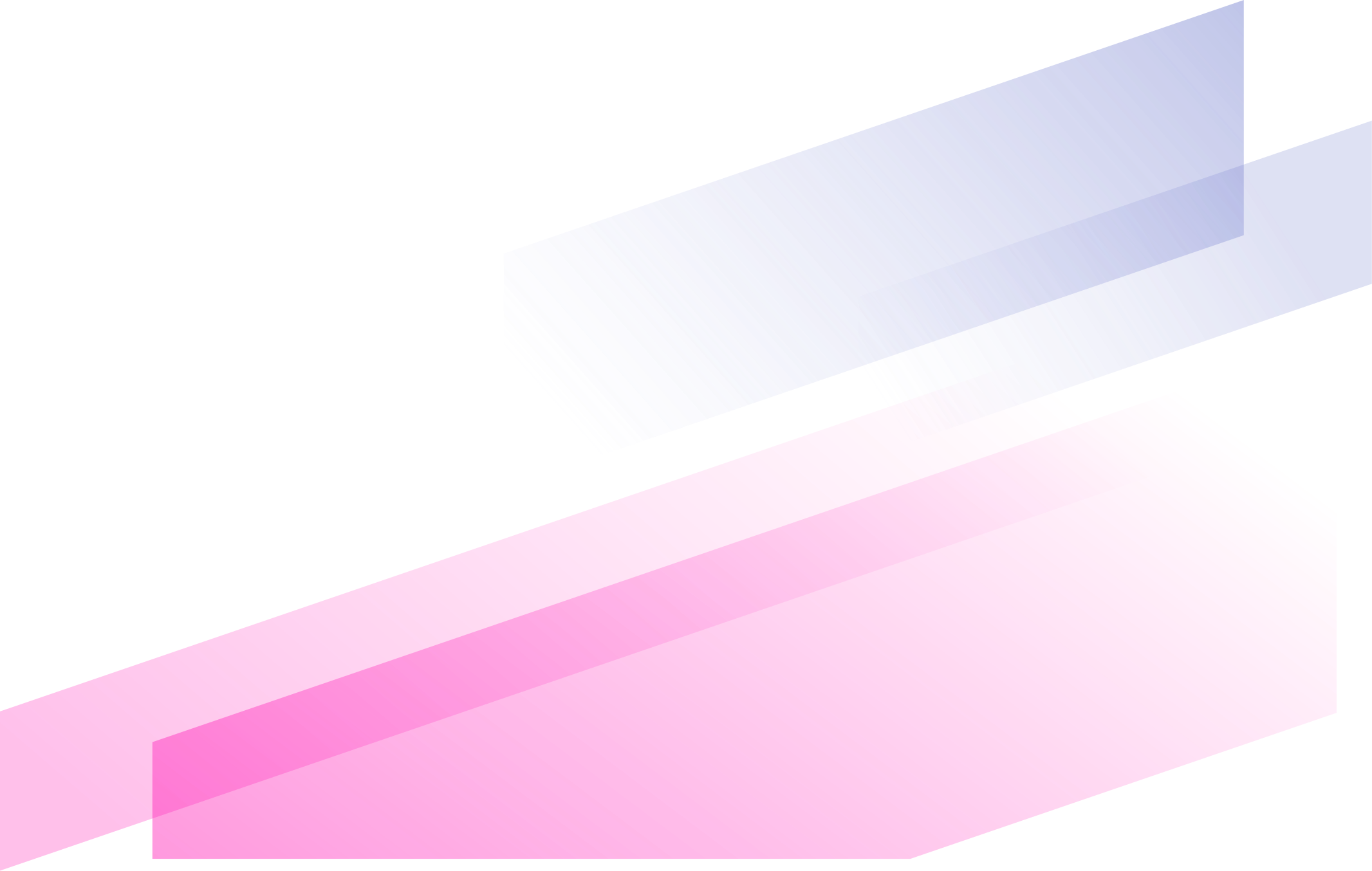
Analysis of glipizide binding to normal and glycated human serum albumin by high-performance affinity chromatography.
Targeting the schizophrenia genome: a fast track strategy from GWAS to clinic.
Relating Essential Proteins to Drug Side-Effects Using Canonical Component Analysis: A Structure-Based Approach.
QSAR Modeling Using Large-Scale Databases: Case Study for HIV-1 Reverse Transcriptase Inhibitors.
Molecular docking and structure-based drug design strategies.
Label Propagation Prediction of Drug-Drug Interactions Based on Clinical Side Effects.
Elucidating Compound Mechanism of Action by Network Perturbation Analysis.
Virtual Screening and Molecular Dynamics Study of Potential Negative Allosteric Modulators of mGluR1 from Chinese Herbs.
Regulation rewiring analysis reveals mutual regulation between STAT1 and miR-155-5p in tumor immunosurveillance in seven major cancers.
Deciding when to stop: efficient experimentation to learn to predict drug-target interactions.