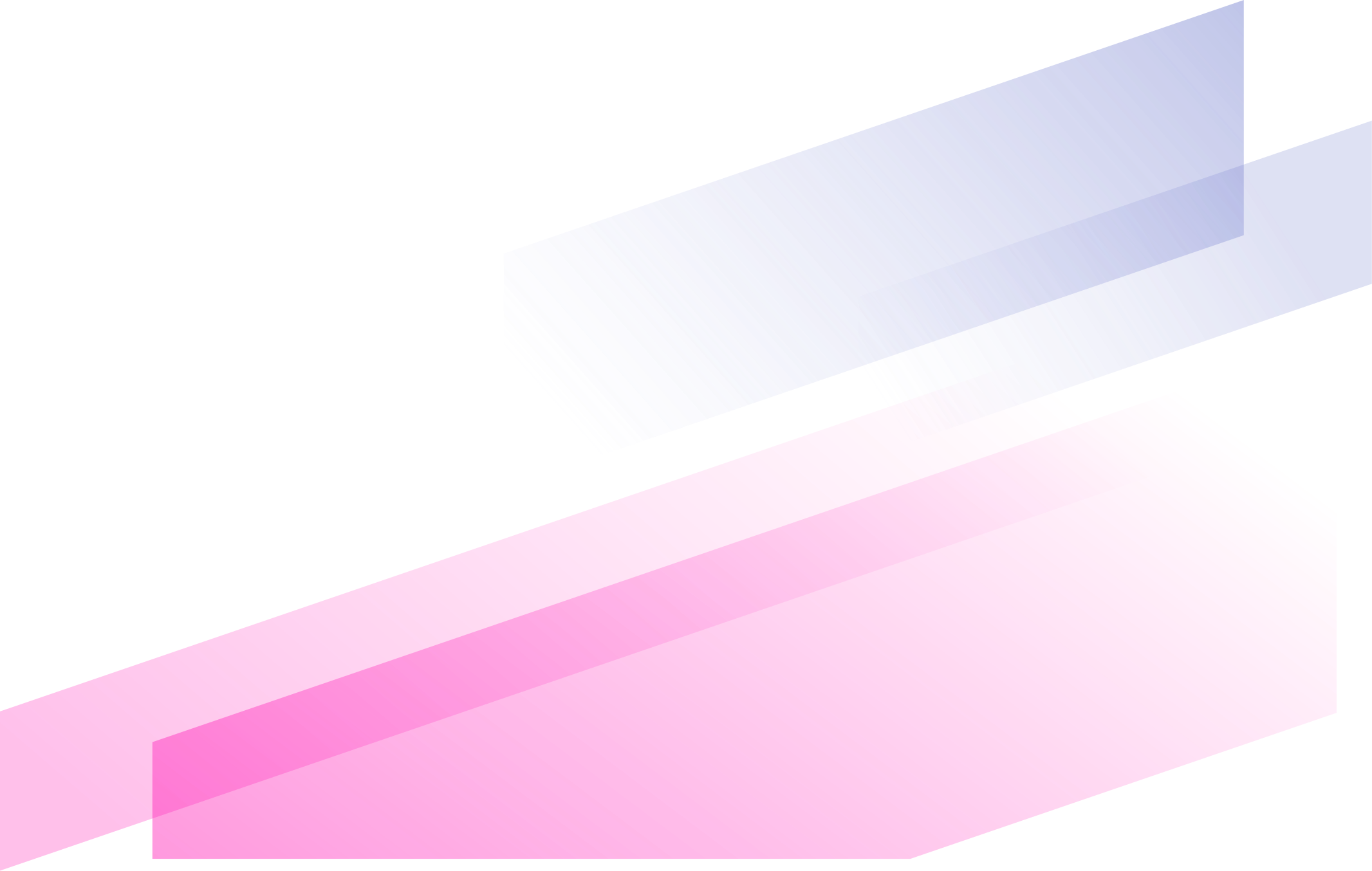
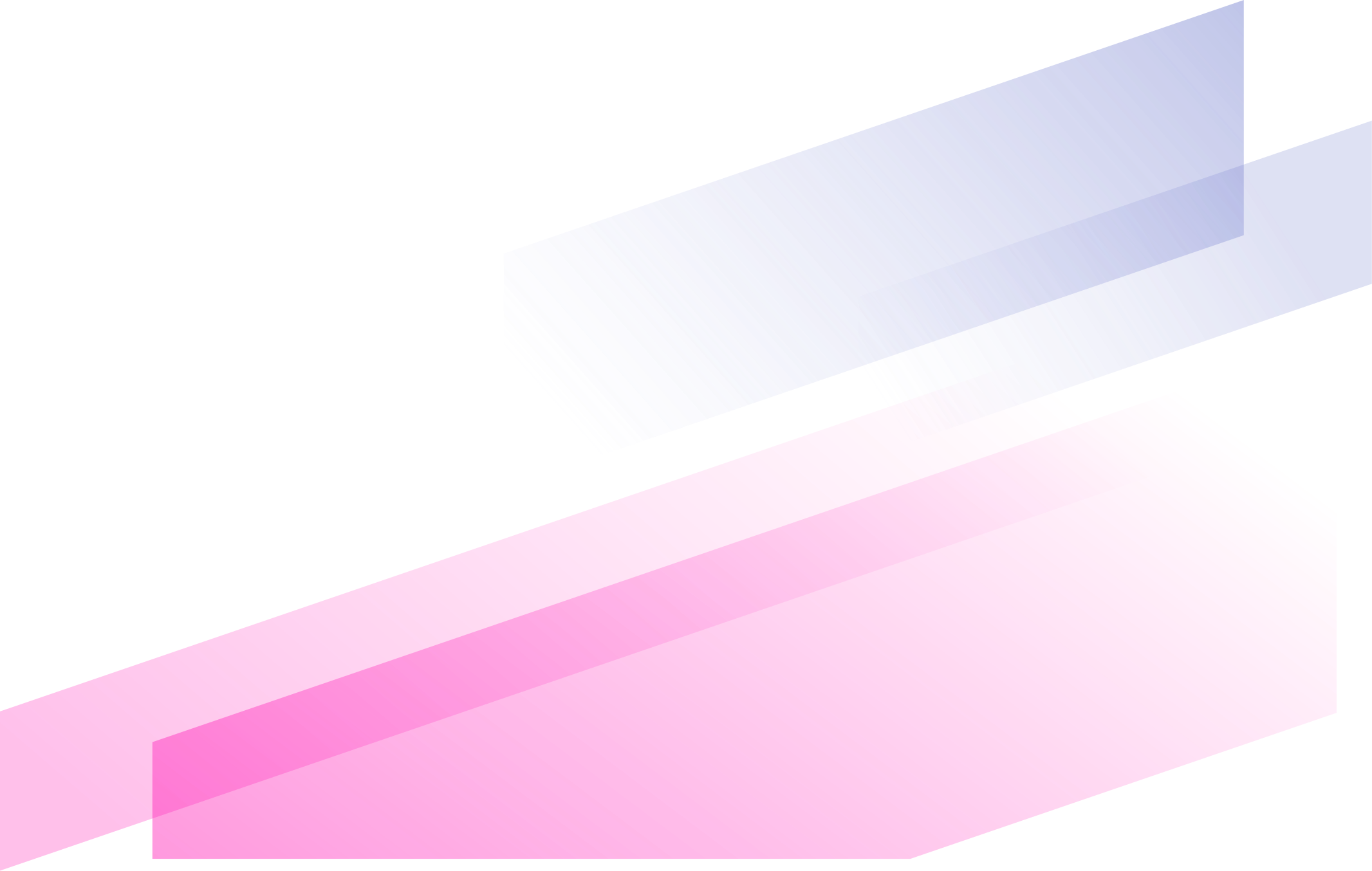
Quantitative and Systems Pharmacology 3. Network-Based Identification of New Targets for Natural Products Enables Potential Uses in Aging-Associated Disorders.
Exploration of charge states of balanol analogues acting as ATP-competitive inhibitors in kinases.
Predicting drug-disease interactions by semi-supervised graph cut algorithm and three-layer data integration.
Dependency-based long short term memory network for drug-drug interaction extraction.
Structure-based prediction of ligand-protein interactions on a genome-wide scale.
Genetic variation in human drug-related genes.
Construction and analysis of gene-gene dynamics influence networks based on a Boolean model.
Investigation and identification of functional post-translational modification sites associated with drug binding and protein-protein interactions.
Econazole nitrate inhibits PI3K activity and promotes apoptosis in lung cancer cells.
MICRA: an automatic pipeline for fast characterization of microbial genomes from high-throughput sequencing data.