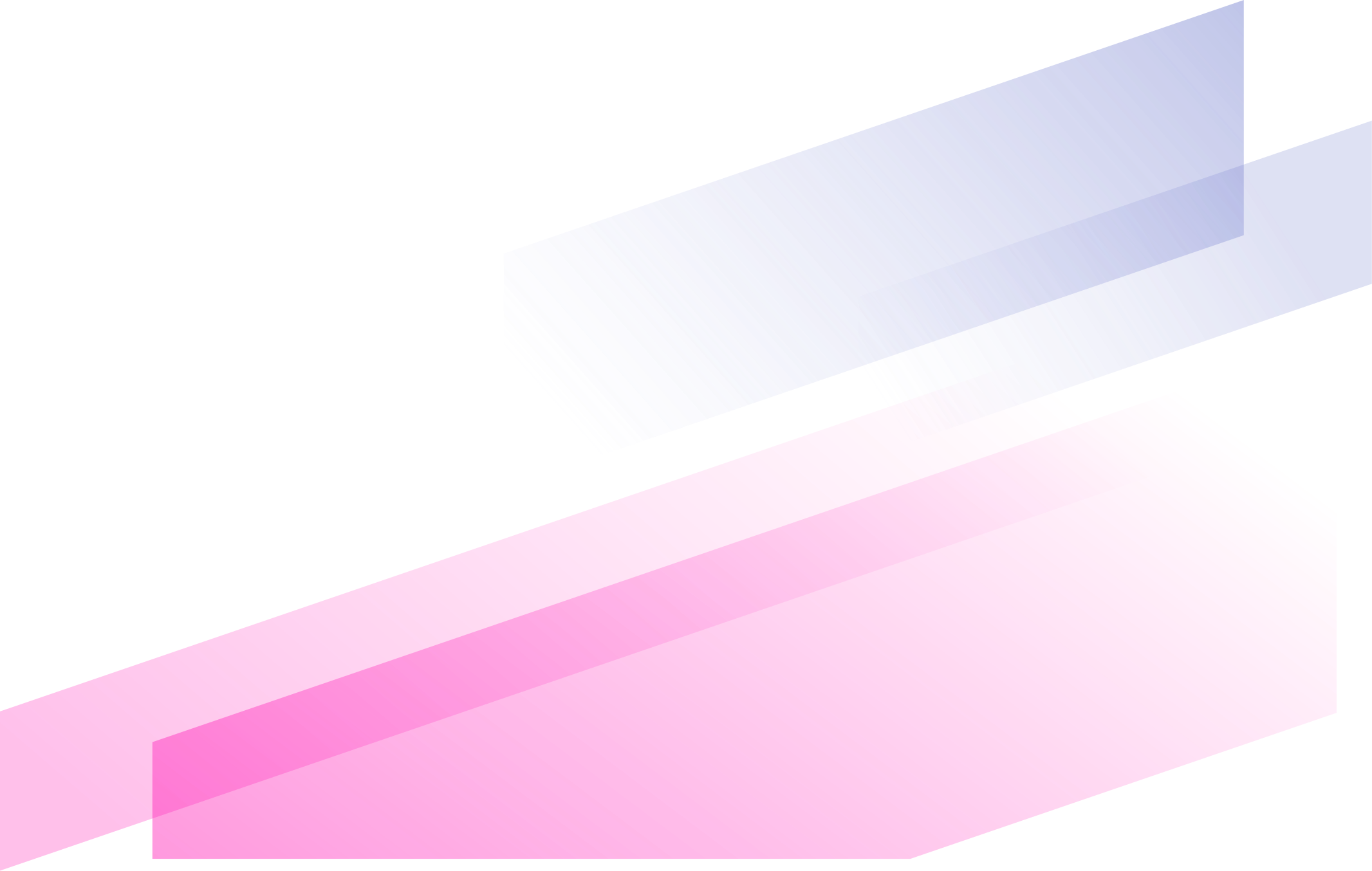
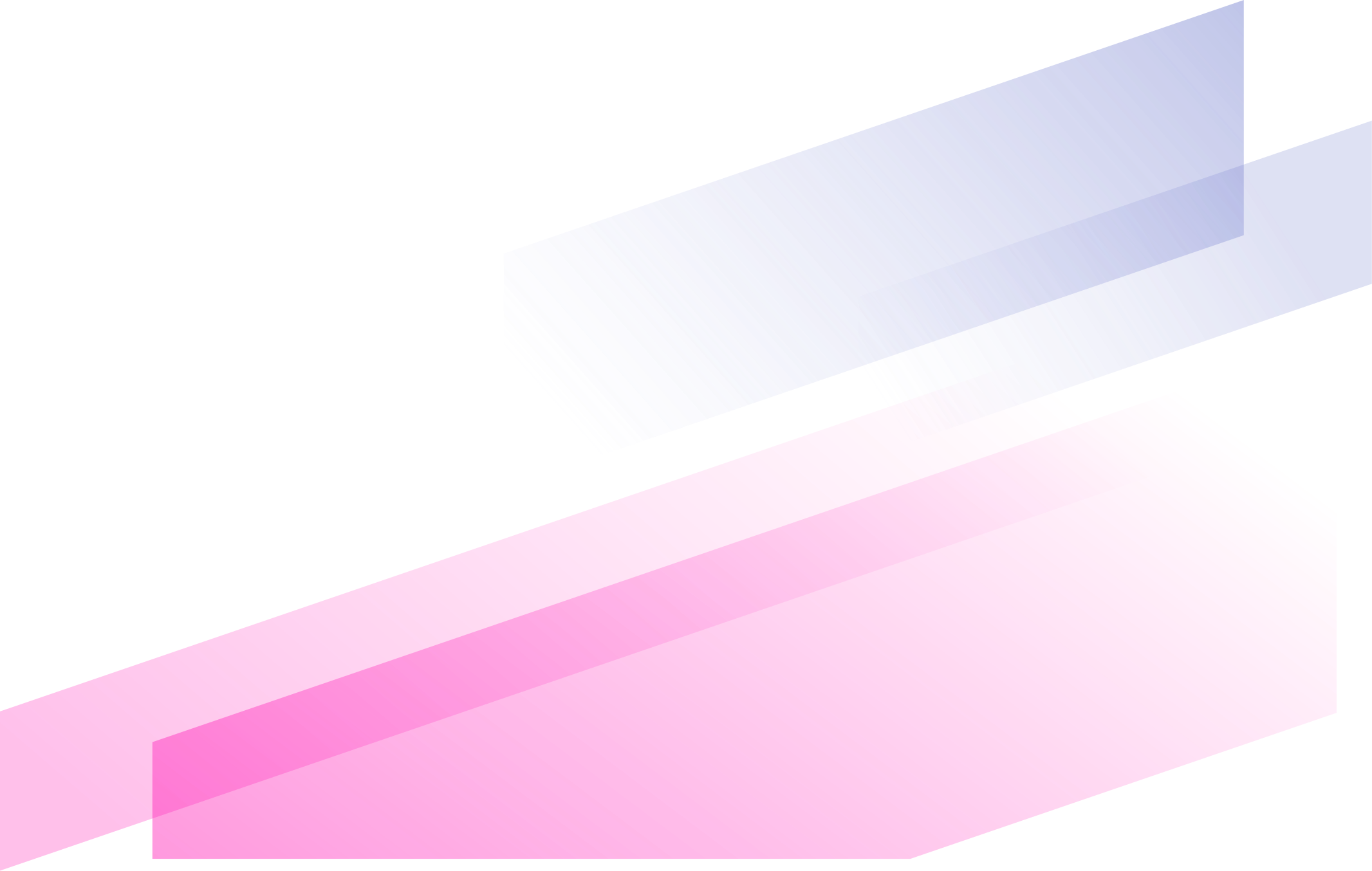
Semi-supervised drug-protein interaction prediction from heterogeneous biological spaces.
Image-based high-throughput drug screening targeting the intracellular stage of Trypanosoma cruzi, the agent of Chagas' disease.
Pancreatic carcinoma cells are susceptible to noninvasive radio frequency fields after treatment with targeted gold nanoparticles.
Mining connections between chemicals, proteins, and diseases extracted from Medline annotations.
A structure-based approach for mapping adverse drug reactions to the perturbation of underlying biological pathways.
Discovery of drug mode of action and drug repositioning from transcriptional responses.
Inhibition of Antiapoptotic BCL-XL, BCL-2, and MCL-1 Proteins by Small Molecule Mimetics.
Targeted Therapy Database (TTD): a model to match patient's molecular profile with current knowledge on cancer biology.
GPCRs, G-proteins, effectors and their interactions: human-gpDB, a database employing visualization tools and data integration techniques.
PharmMapper server: a web server for potential drug target identification using pharmacophore mapping approach.