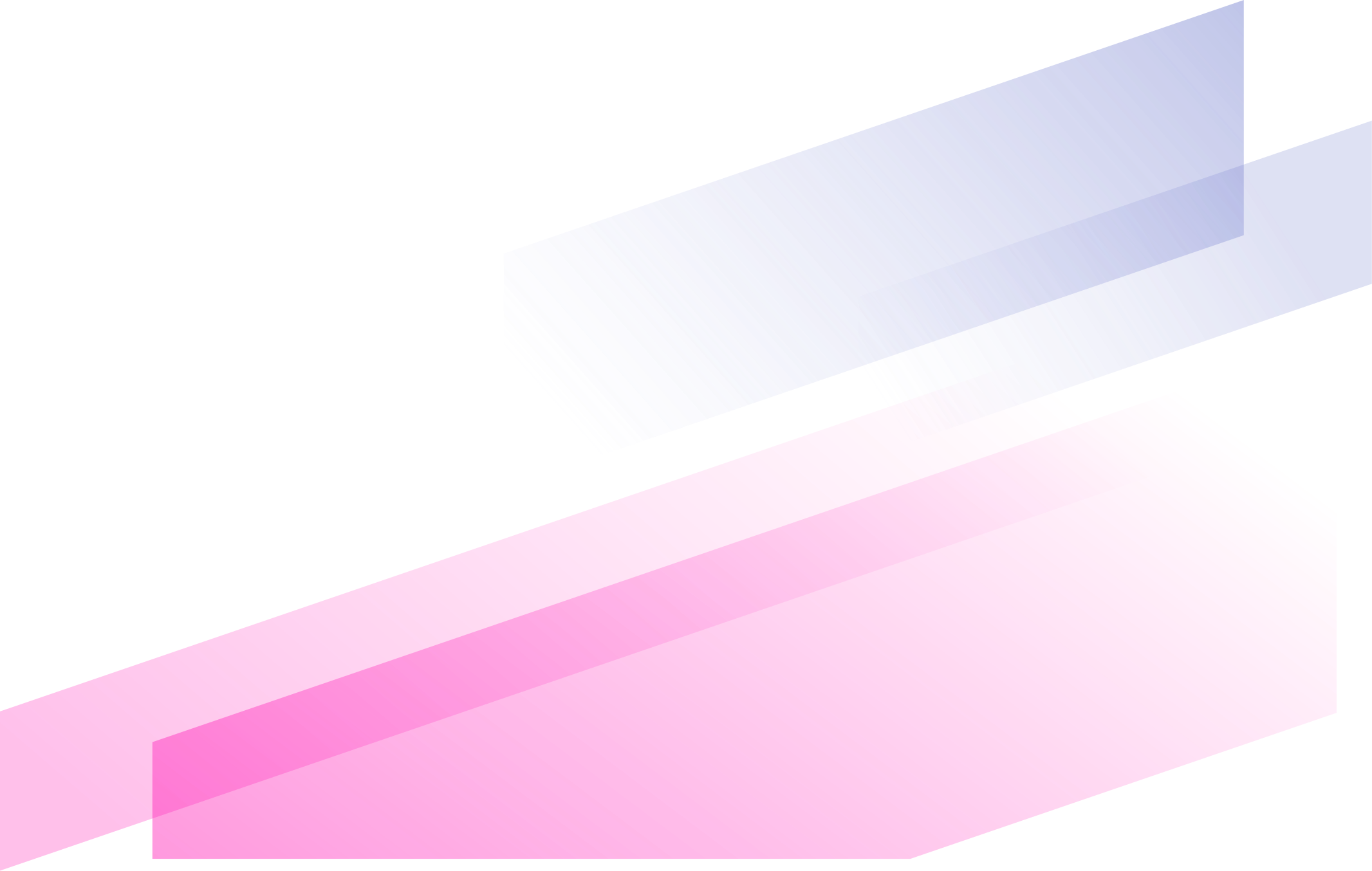
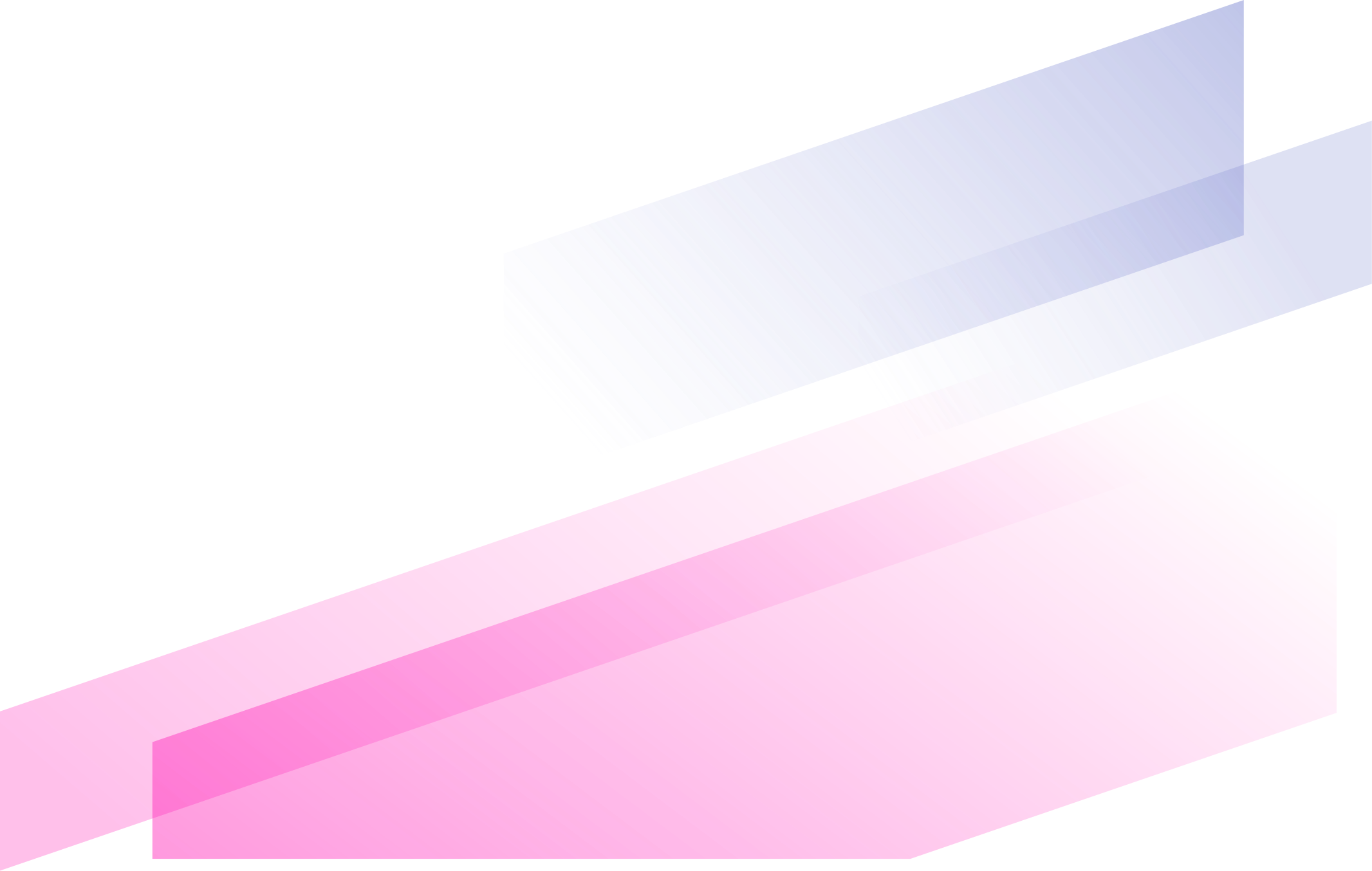
Genome-scale metabolic modeling reveals SARS-CoV-2-induced host metabolic reprogramming and identifies metabolic antiviral targets.
Construction of a TF-miRNA-gene feed-forward loop network predicts biomarkers and potential drugs for myasthenia gravis.
Forecasting System of Computational Time of DFT/TDDFT Calculations under the Multiverse Ansatz via Machine Learning and Cheminformatics.
Exploring the Active Components of Simotang Oral Liquid and Their Potential Mechanism of Action on Gastrointestinal Disorders by Integrating Ultrahigh-Pressure Liquid Chromatography Coupled with Linear Ion Trap-Orbitrap Analysis and Network Pharmacology.
Improving Exposure Assessment Using Non-Targeted and Suspect Screening: The ISO/IEC 17025: 2017 Quality Standard as a Guideline.
DiaNat-DB: a molecular database of antidiabetic compounds from medicinal plants.
A network pharmacology perspective for deciphering potential mechanisms of action of Solanum nigrum L. in bladder cancer.
Identification of 37 Heterogeneous Drug Candidates for Treatment of COVID-19 via a Rational Transcriptomics-Based Drug Repurposing Approach.
RNA dependent RNA polymerase (RdRp) as a drug target for SARS-CoV2.
SNF-NN: computational method to predict drug-disease interactions using similarity network fusion and neural networks.